Nonlinear Reduced DNN Models for State Estimation
COMMUNICATIONS IN COMPUTATIONAL PHYSICS(2022)
摘要
We propose in this paper a data driven state estimation scheme for generating nonlinear reduced models for parametric families of PDEs, directly providing data-to-state maps, represented in terms of Deep Neural Networks. A major constituent is a sensor-induced decomposition of a model-compliant Hilbert space warranting approximation in problem relevant metrics. It plays a similar role as in a Parametric Background Data Weak framework for state estimators based on Reduced Basis concepts. Extensive numerical tests shed light on several optimization strategies that are to improve robustness and performance of such estimators.
更多查看译文
关键词
State estimation in model-compliant norms, deep neural networks, sensor coordi-nates, reduced bases, ResNet structures, network expansion
AI 理解论文
溯源树
样例
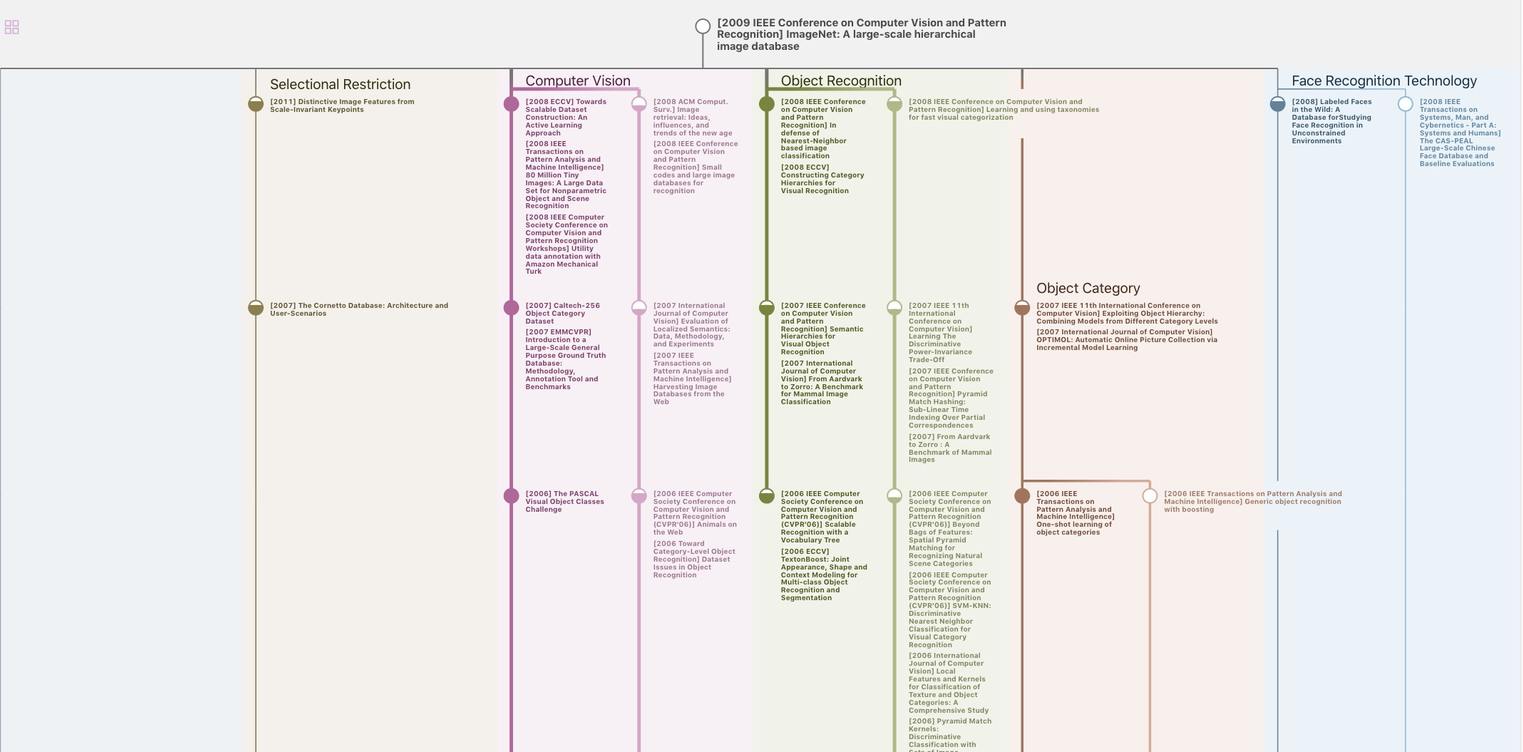
生成溯源树,研究论文发展脉络
Chat Paper
正在生成论文摘要