Poisoning Attacks on Fair Machine Learning
Database Systems for Advanced Applications(2022)
摘要
Both fair machine learning and adversarial learning have been extensively studied. However, attacking fair machine learning models has received less attention. In this paper, we present a framework that seeks to effectively generate poisoning samples to attack both model accuracy and algorithmic fairness. Our attacking framework can target fair machine learning models trained with a variety of group based fairness notions such as demographic parity and equalized odds. We develop three online attacks, adversarial sampling, adversarial labeling, and adversarial feature modification. All three attacks effectively and efficiently produce poisoning samples via sampling, labeling, or modifying a fraction of training data in order to reduce the test accuracy. Our framework enables attackers to flexibly adjust the attack’s focus on prediction accuracy or fairness and accurately quantify the impact of each candidate point to both accuracy loss and fairness violation, thus producing effective poisoning samples. Experiments on two real datasets demonstrate the effectiveness and efficiency of our framework.
更多查看译文
关键词
Poisoning attacks, Algorithmic fairness, Adversarial machine learning.
AI 理解论文
溯源树
样例
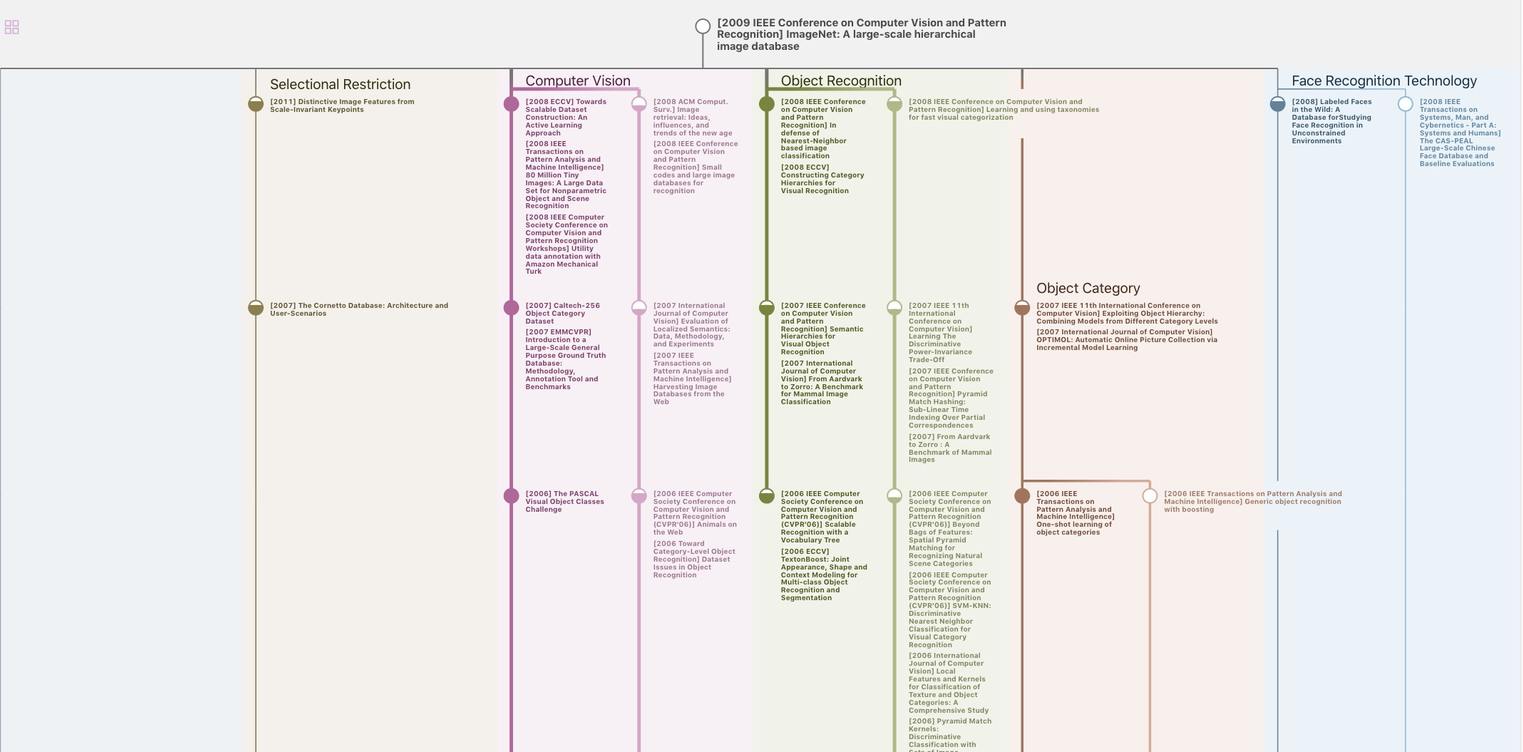
生成溯源树,研究论文发展脉络
Chat Paper
正在生成论文摘要