The Power of Prompt Tuning for Low-Resource Semantic Parsing
PROCEEDINGS OF THE 60TH ANNUAL MEETING OF THE ASSOCIATION FOR COMPUTATIONAL LINGUISTICS (ACL 2022): (SHORT PAPERS), VOL 2(2022)
摘要
Prompt tuning has recently emerged as an effective method for adapting pre-trained language models to a number of language understanding and generation tasks. In this paper, we investigate prompt tuning for semantic parsing-the task of mapping natural language utterances onto formal meaning representations. On the low-resource splits of Overnight and TOPv2, we find that a prompt tuned T5-xl significantly outperforms its fine-tuned counterpart, as well as strong GPT-3 and BART baselines. We also conduct ablation studies across different model scales and target representations, finding that, with increasing model scale, prompt tuned T5 models improve at generating target representations that are far from the pre-training distribution.
更多查看译文
关键词
semantic parsing,prompt tuning,low-resource
AI 理解论文
溯源树
样例
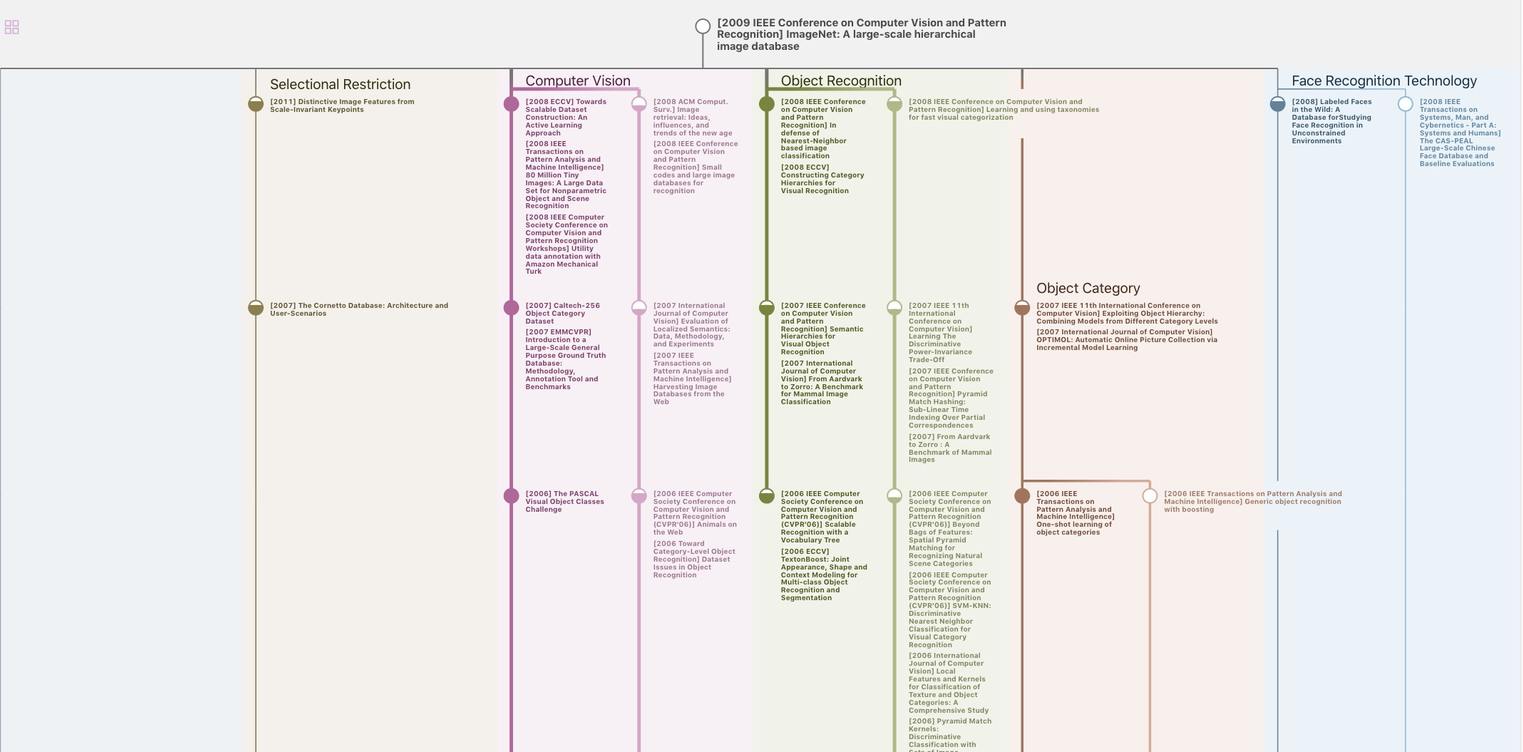
生成溯源树,研究论文发展脉络
Chat Paper
正在生成论文摘要