TESDA: Transform Enabled Statistical Detection of Attacks in Deep Neural Networks
arxiv(2021)
摘要
Deep neural networks (DNNs) are now the de facto choice for computer vision tasks such as image classification. However, their complexity and "black box" nature often renders the systems they're deployed in vulnerable to a range of security threats. Successfully identifying such threats, especially in safety-critical real-world applications is thus of utmost importance, but still very much an open problem. We present TESDA, a low-overhead, flexible, and statistically grounded method for {online detection} of attacks by exploiting the discrepancies they cause in the distributions of intermediate layer features of DNNs. Unlike most prior work, we require neither dedicated hardware to run in real-time, nor the presence of a Trojan trigger to detect discrepancies in behavior. We empirically establish our method's usefulness and practicality across multiple architectures, datasets and diverse attacks, consistently achieving detection coverages of above 95% with operation count overheads as low as 1-2%.
更多查看译文
关键词
attacks,deep neural networks,statistical detection,neural networks
AI 理解论文
溯源树
样例
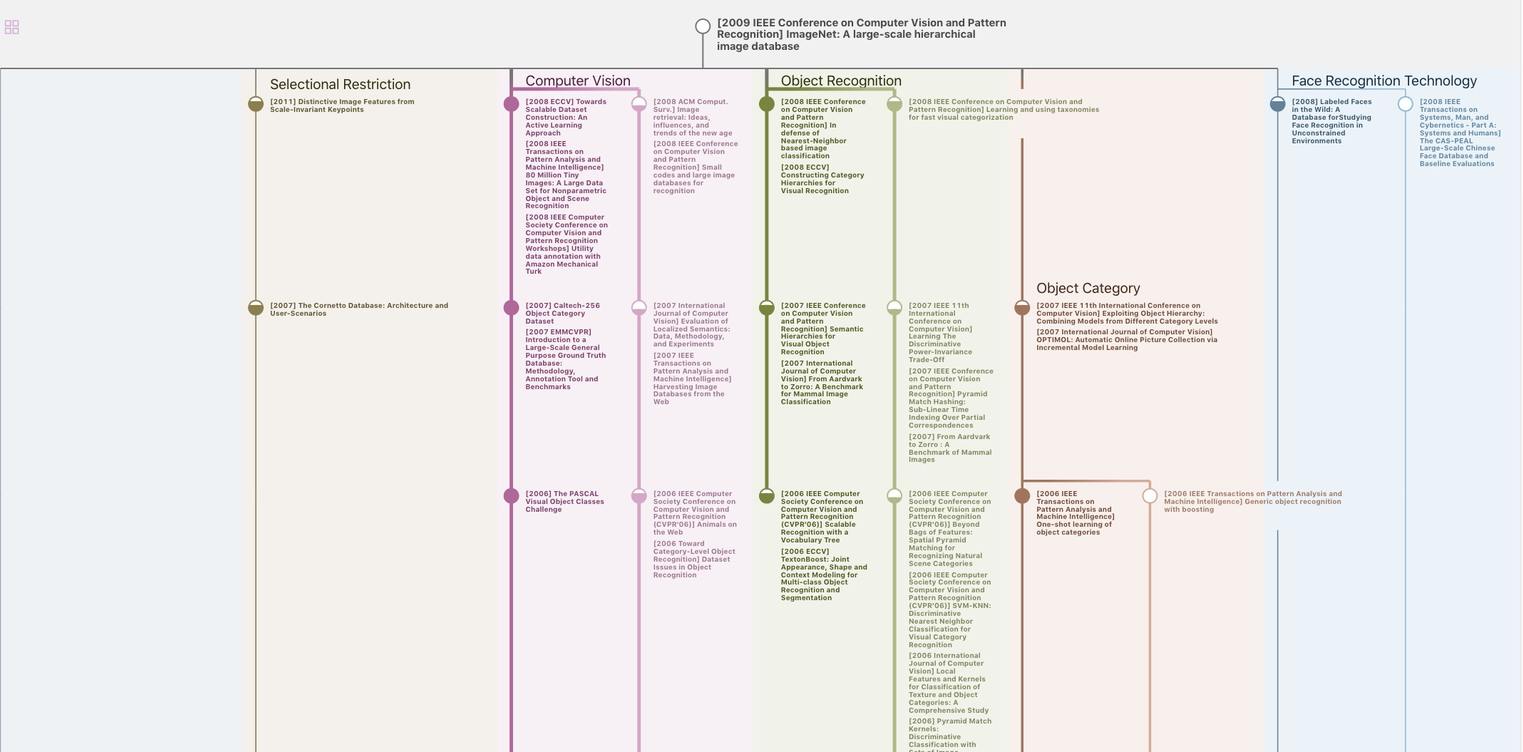
生成溯源树,研究论文发展脉络
Chat Paper
正在生成论文摘要