Nuances in Margin Conditions Determine Gains in Active Learning
INTERNATIONAL CONFERENCE ON ARTIFICIAL INTELLIGENCE AND STATISTICS, VOL 151(2022)
摘要
We consider nonparametric classification with smooth regression functions, where it is well known that notions of margin in E[Y vertical bar X] determine fast or slow rates in both active and passive learning. Here we elucidate a striking distinction between the two settings. Namely, we show that some seemingly benign nuances in notions of margin somehow involving the uniqueness of the Bayes classifier, and which have no apparent effect on rates in passive learning determine whether or not any active learner can outperform passive learning rates. In particular, for Audibert-Tsybakov's margin condition (allowing general situations with non-unique Bayes classifiers), no active learner can gain over passive learning in commonly studied settings where the marginal on X is near uniform. Our results thus negate the usual intuition from past literature that active rates should generally improve over passive rates in non-parametric settings.
更多查看译文
关键词
margin conditions determine gains,learning,active
AI 理解论文
溯源树
样例
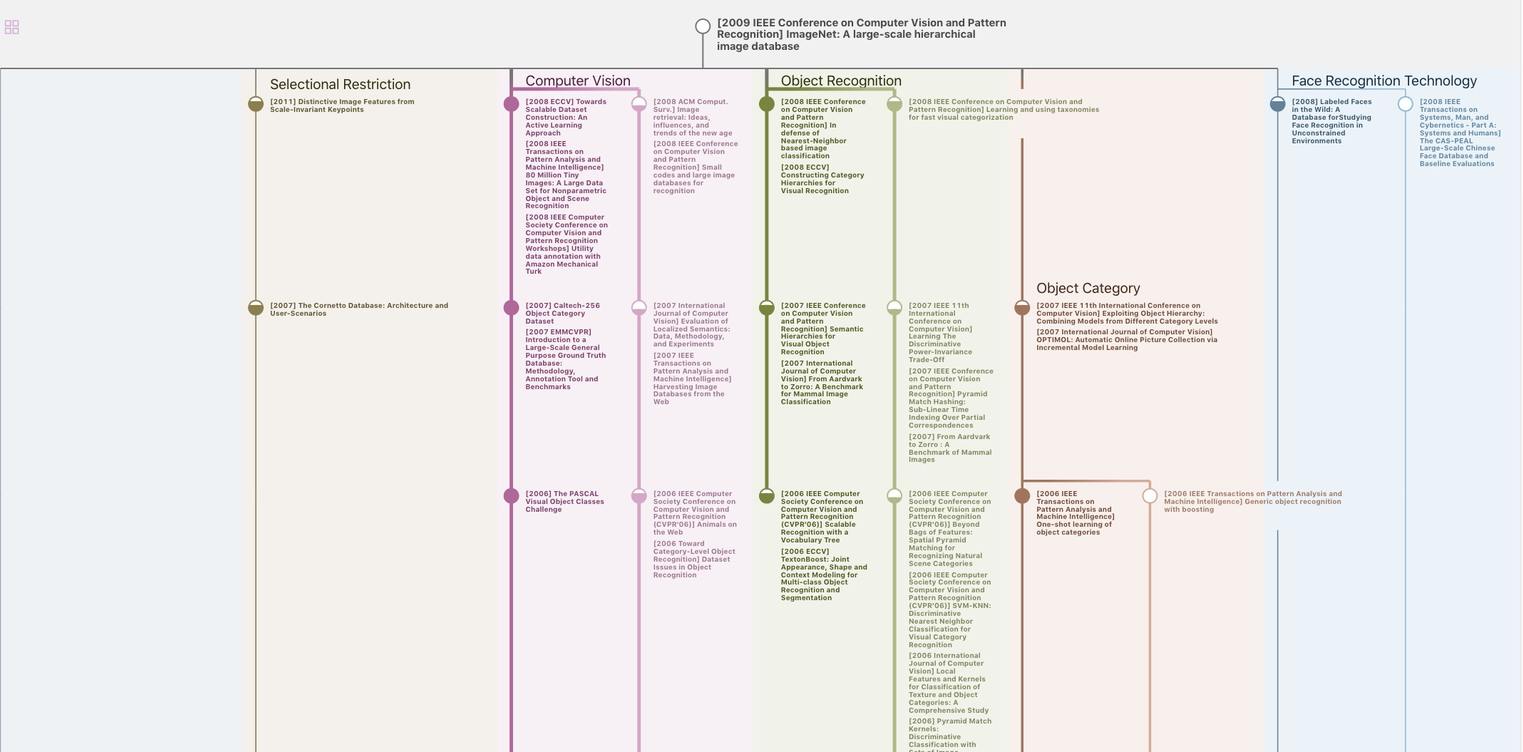
生成溯源树,研究论文发展脉络
Chat Paper
正在生成论文摘要