Tricks for Training Sparse Translation Models
North American Chapter of the Association for Computational Linguistics (NAACL)(2022)
摘要
Multi-task learning with an unbalanced data distribution skews model learning towards high resource tasks, especially when model capacity is fixed and fully shared across all tasks. Sparse scaling architectures, such as BASELayers, provide flexible mechanisms for different tasks to have a variable number of parameters, which can be useful to counterbalance skewed data distributions. We find that that sparse architectures for multilingual machine translation can perform poorly out of the box, and propose two straightforward techniques to mitigate this - a temperature heating mechanism and dense pre-training. Overall, these methods improve performance on two multilingual translation benchmarks compared to standard BASELayers and Dense scaling baselines, and in combination, more than 2x model convergence speed.
更多查看译文
关键词
translation,models
AI 理解论文
溯源树
样例
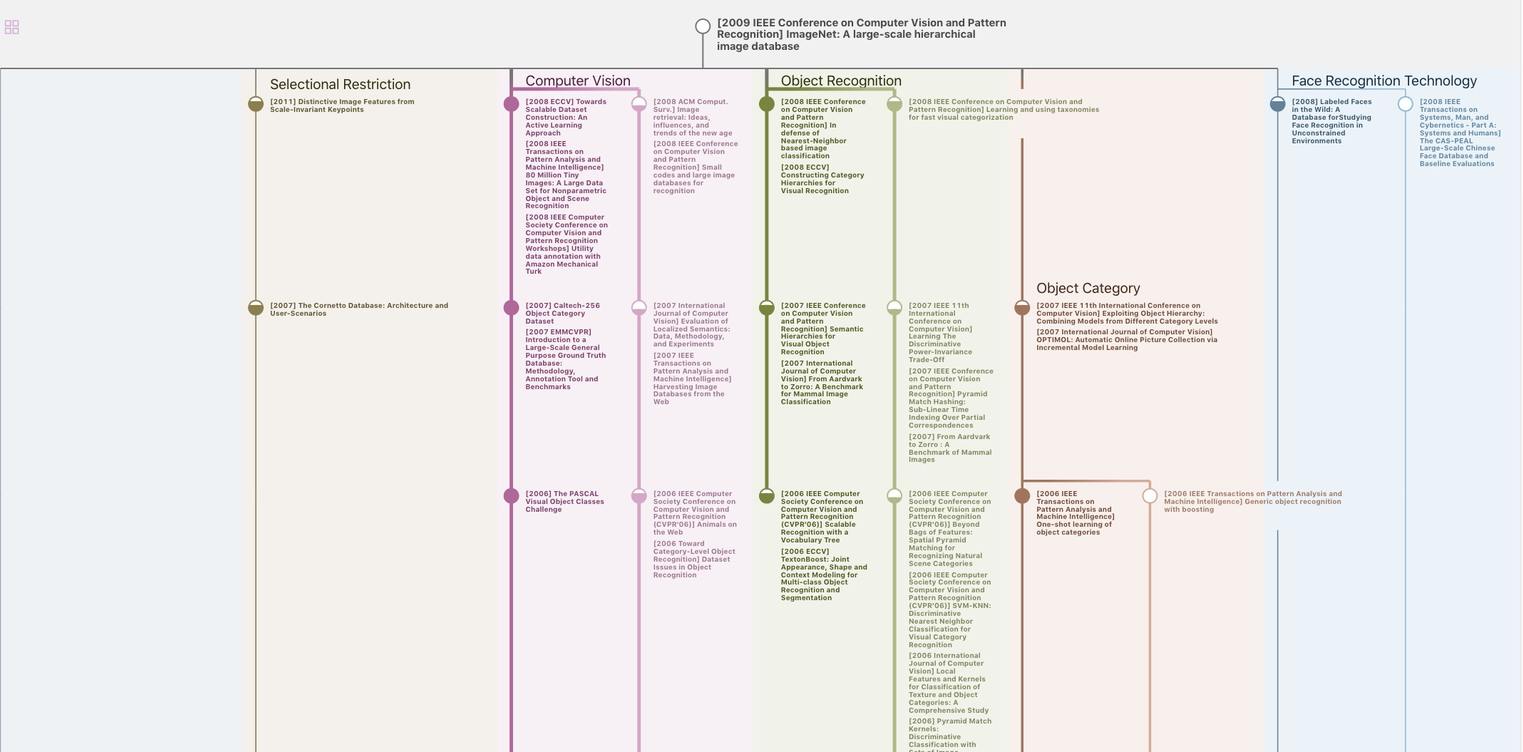
生成溯源树,研究论文发展脉络
Chat Paper
正在生成论文摘要