Learn Proportional Derivative Controllable Latent Space from Pixels.
IEEE Conference on Automation Science and Engineering (CASE)(2022)
摘要
Recent advances in latent space dynamics model from pixels show promising progress in vision-based model predictive control (MPC). However, executing MPC in real time can be challenging due to its intensive computational cost in each timestep. We propose to introduce additional learning objectives to enforce that the learned latent space is proportional derivative controllable. In execution time, the simple PD-controller can be applied directly to the latent space encoded from pixels, to produce simple and effective control to systems with visual observations. We show that our method outperforms baseline methods to produce robust goal reaching and trajectory tracking in various environments.
更多查看译文
关键词
proportional derivative controllable latent space,latent space dynamics model,pixels show,promising progress,vision-based model predictive control,MPC,intensive computational cost,additional learning objectives,learned latent space,execution time,simple PD-controller,simple control
AI 理解论文
溯源树
样例
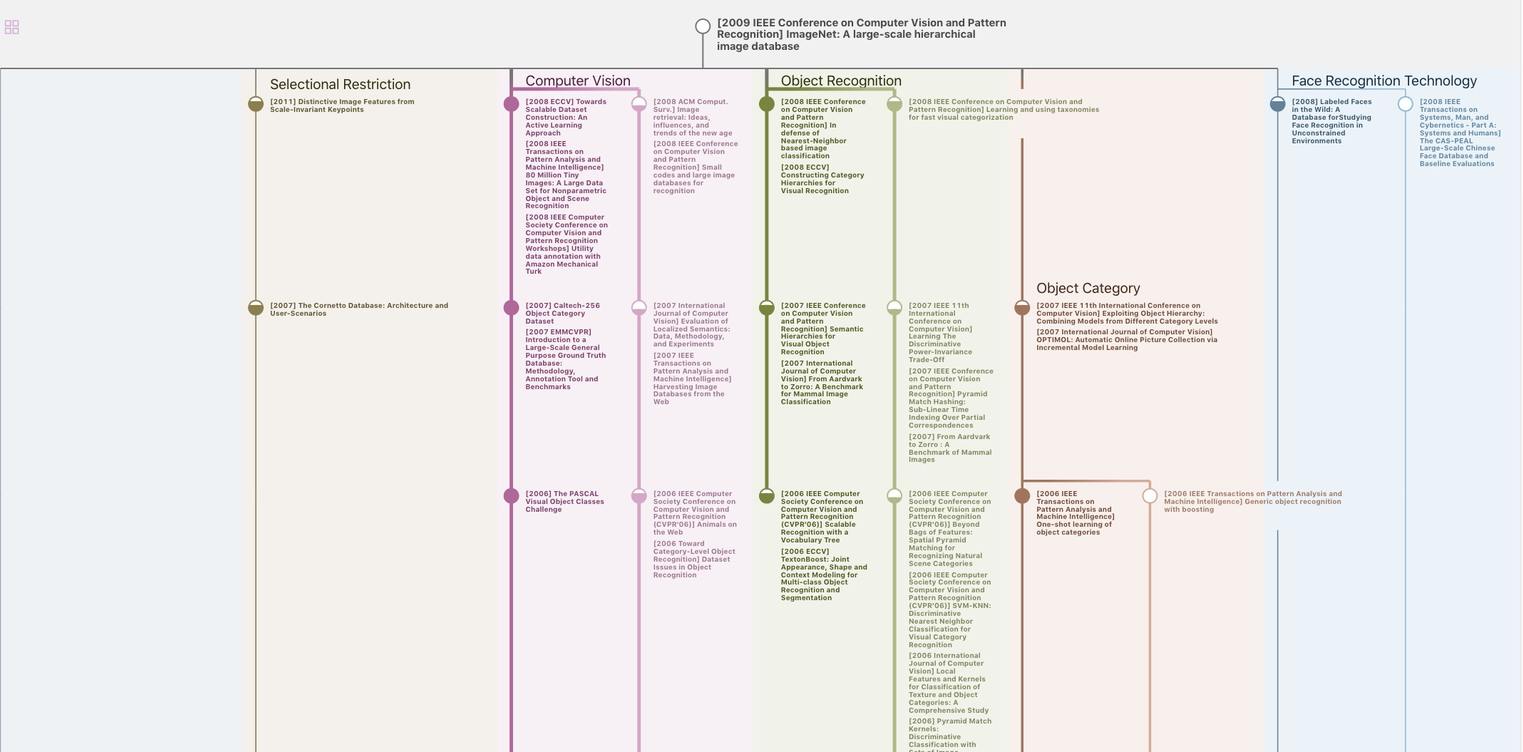
生成溯源树,研究论文发展脉络
Chat Paper
正在生成论文摘要