Entropy-Based Learning of Compositional Models from Data
BELIEF FUNCTIONS: THEORY AND APPLICATIONS (BELIEF 2021)(2021)
摘要
We investigate learning of belief function compositional models from data using information content and mutual information based on two different definitions of entropy proposed by Jirousek and Shenoy in 2018 and 2020, respectively. The data consists of 2,310 randomly generated basic assignments of 26 binary variables from a pairwise consistent and decomposable compositional model. We describe results achieved by three simple greedy algorithms for constructing compositional models from the randomly generated low-dimensional basic assignments.
更多查看译文
关键词
Compositional models, Entropy of Dempster-Shafer belief functions, Decomposable entropy of Dempster-Shafer belief functions, Mutual information, Information content
AI 理解论文
溯源树
样例
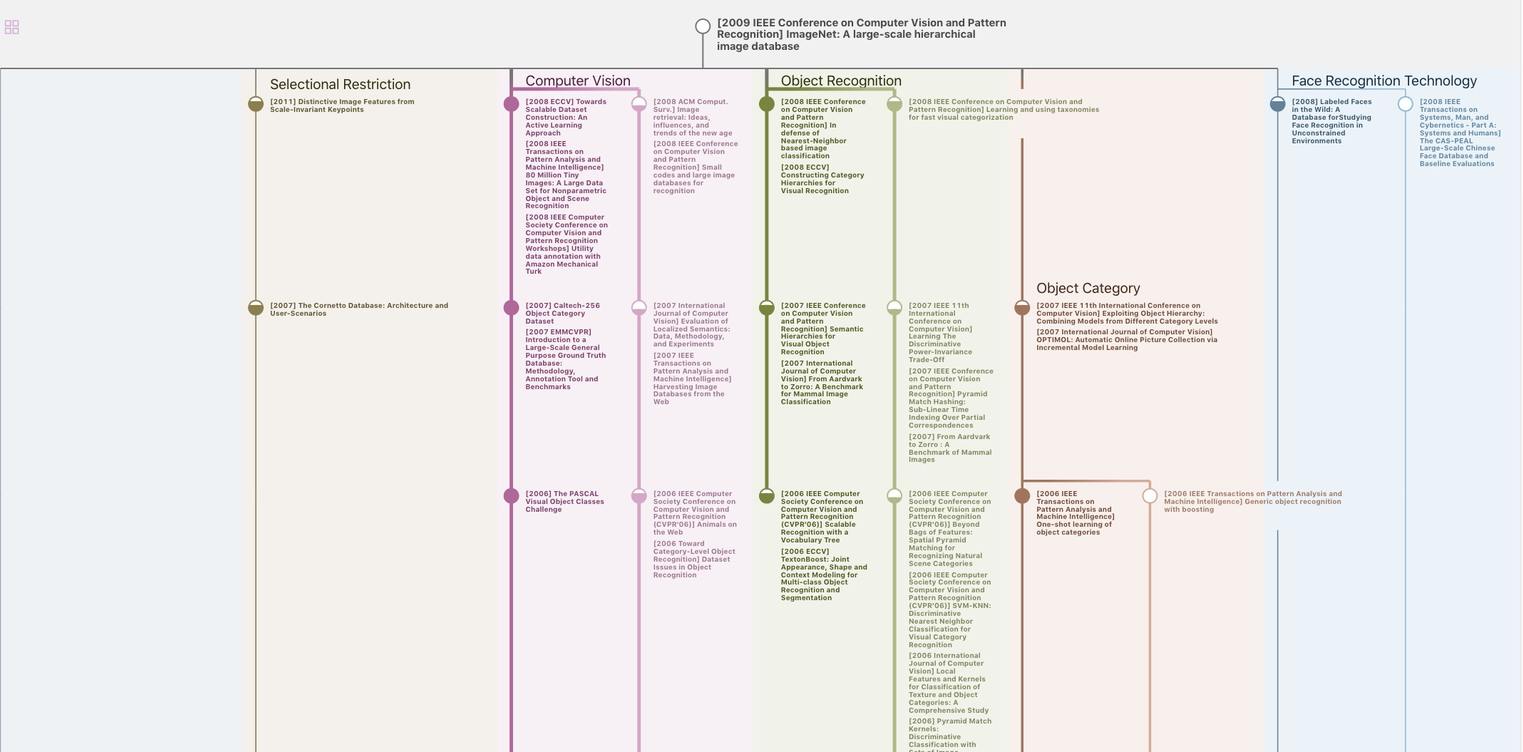
生成溯源树,研究论文发展脉络
Chat Paper
正在生成论文摘要