Learning to Select Relevant Knowledge for Neural Machine Translation.
NLPCC(2021)
摘要
Most memory-based methods use encoded retrieved pairs as the translation memory (TM) to provide external guidance, but there still exist some noisy words in the retrieved pairs. In this paper, we propose a simple and effective end-to-end model to select useful sentence words from the encoded memory and incorporate them into the NMT model. Our model uses a novel memory selection mechanism to avoid the noise from similar sentences and provide external guidance simultaneously. To verify the positive influence of selected retrieved words, we evaluate our model on the single-domain dataset namely JRC-Acquis and multi-domain dataset comprised of existing benchmarks including WMT, IWSLT, JRC-Acquis, and OpenSubtitles. Experimental results demonstrate our method can improve the translation quality under different scenarios.
更多查看译文
关键词
Neural machine translation, Selective translation memory
AI 理解论文
溯源树
样例
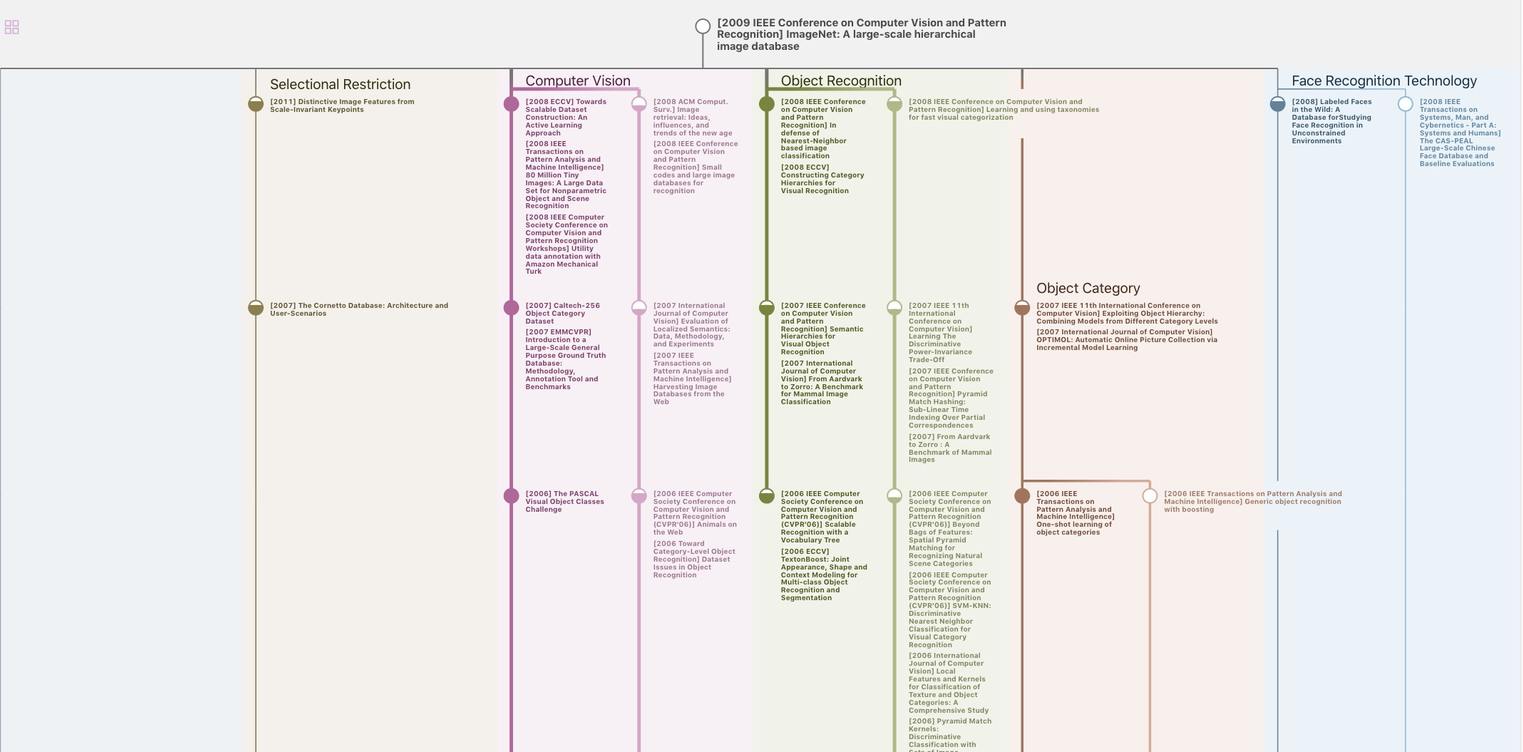
生成溯源树,研究论文发展脉络
Chat Paper
正在生成论文摘要