Aggregating Inter-viewpoint Relationships of User’s Review for Accurate Recommendation
NLPCC(2021)
摘要
User reviews contain rich information about user interests in items. Recently, many deep learning methods have attempted to integrate review contents into user preference rating prediction, helping to solve data sparseness problems. However, existing methods suffered from an inherent limitation that many reviews are noisy and contain non-consecutive viewpoints, besides, they are insufficient to capture inter-viewpoint relationships. Incorporating useful information is helpful for more accurate recommendations. In this paper, we propose a neural recommendation approach with a Diversity Penalty mechanism and Capsule Networks, named DPCN. Specifically, the diversity penalty component employs weight distributed matrices with the penalization term to capture different viewpoints in textual reviews. The capsule networks are designed to aggregate individual viewpoint vectors to form high-level feature representations for feature interaction. Then we combine the review feature representations with the user and item ID embedding for final rating prediction. Extensive experiments on five real-world datasets validate the effectiveness of our approach.
更多查看译文
关键词
Recommender systems,User reviews,Capsule networks,Rating prediction
AI 理解论文
溯源树
样例
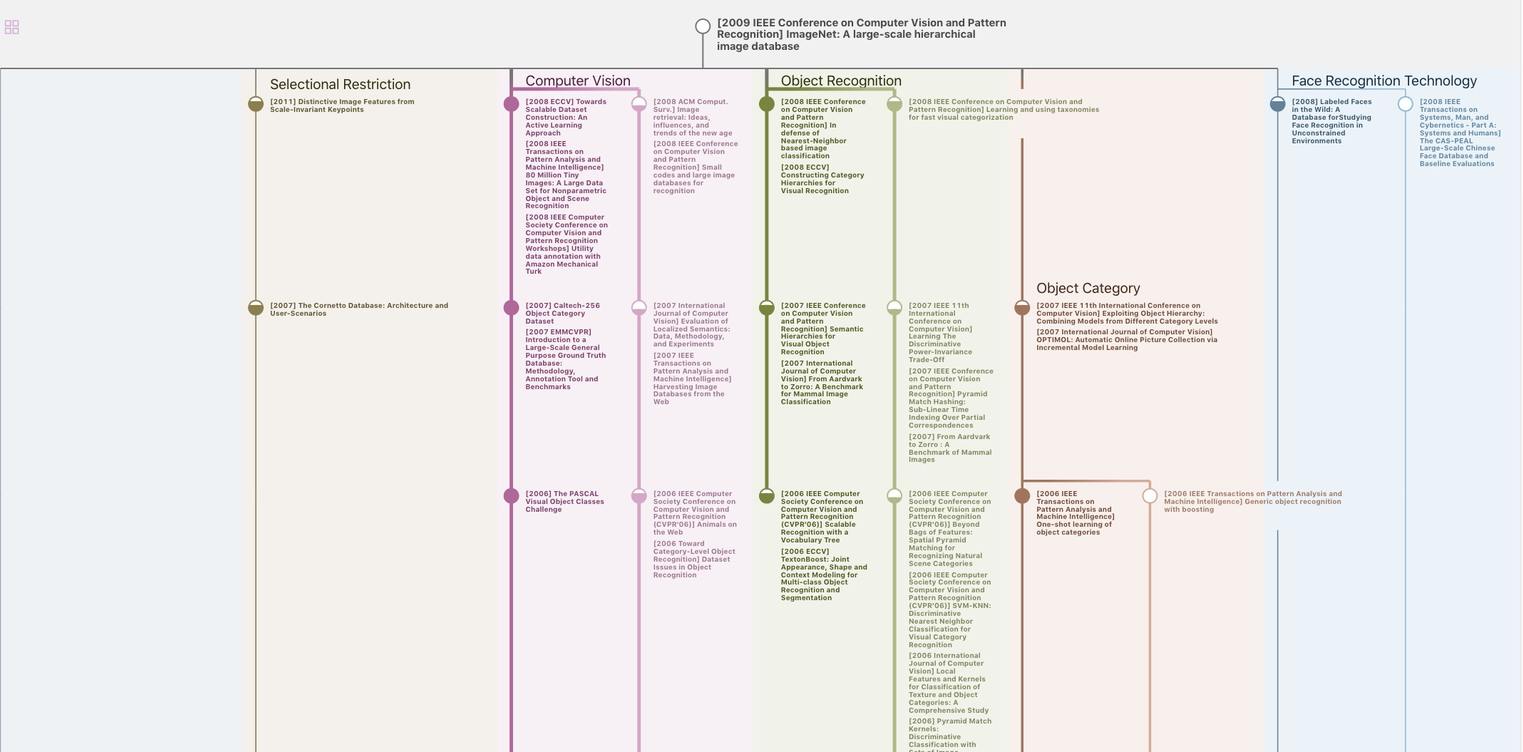
生成溯源树,研究论文发展脉络
Chat Paper
正在生成论文摘要