Deep Progressive Attention For Person Re-Identification
JOURNAL OF ELECTRONIC IMAGING(2021)
摘要
Person re-identification (Re-ID) aims to retrieve specific individuals across non overlapping camera views. In recent years, attention-based models contribute to many computer vision tasks due to their great ability for learning discriminative features. We propose the deep progressive attention (DPA) in a more natural manner for person Re-ID. Similar to human visual attention mechanism, the proposed DPA progressively selects the most discriminative parts of a specific individual and formulates feature representation for comparison purpose. Concretely, on the one hand, the proposed DPA uses a long-term reward to optimize the discriminative feature selection. On the other hand, a deep convolutional architecture is integrated into a recurrent model for feature representation learning. Extensive experiments on three person Re-ID benchmarks Market-1501, DukeMTMC-reID, and CUHK03-NP demonstrate the proposed DPA is on par with the state-of-the-art. Moreover, the experiments on partial person Re-ID datasets indicate the proposed DPA is competitive with the specially designed partial person Re-ID methods. (c) 2021 SPIE and IS&T [DOI: 10.1117/1.JEI.30.4.043028]
更多查看译文
关键词
person reidentification, attention, reinforcement learning
AI 理解论文
溯源树
样例
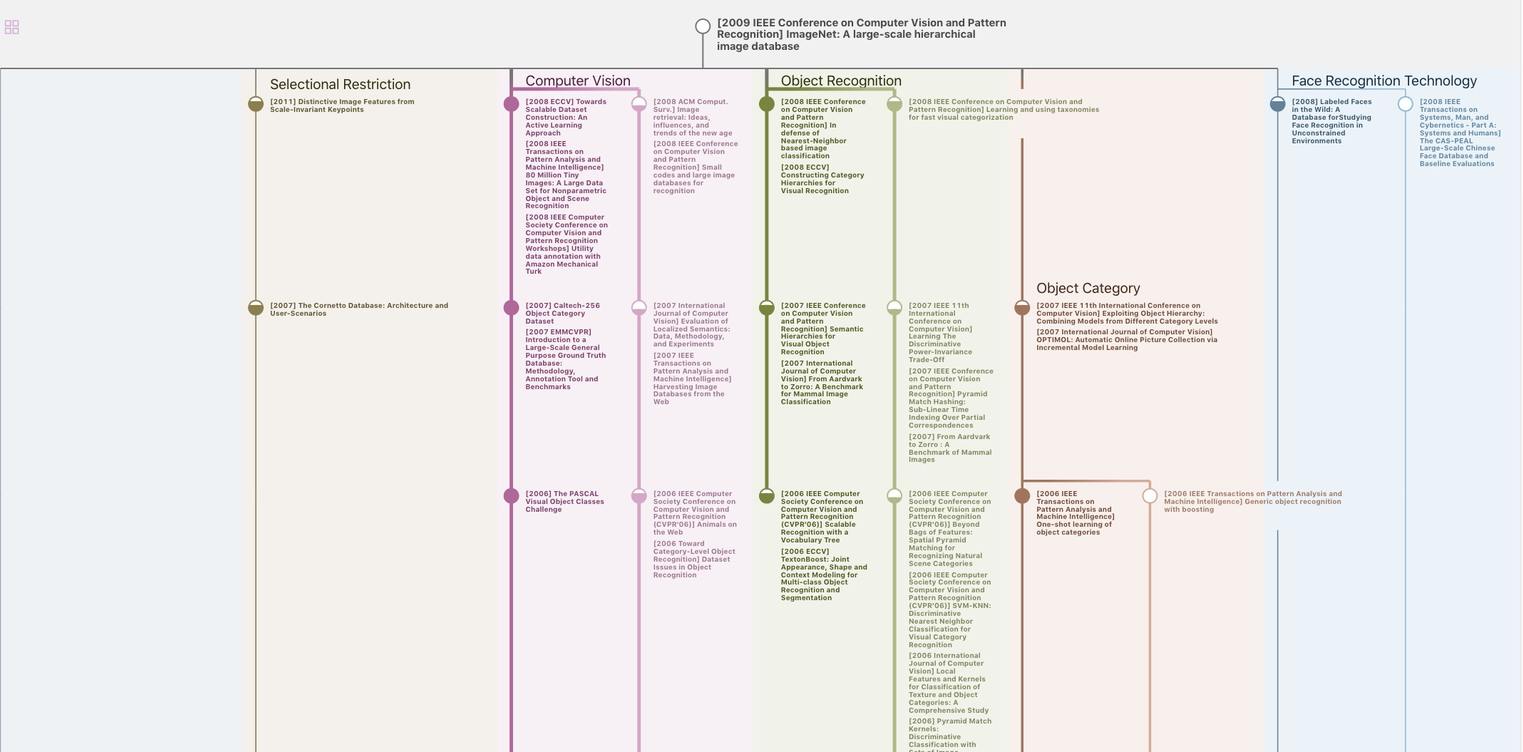
生成溯源树,研究论文发展脉络
Chat Paper
正在生成论文摘要