Imbalanced Data Enhancement Method Based On Improved Dcgan And Its Application
JOURNAL OF INTELLIGENT & FUZZY SYSTEMS(2021)
摘要
Machinery operates well under normal conditions in most cases; far fewer samples are collected in a fault state (minority samples) than in a normal state, resulting in an imbalance of samples. Common machine learning algorithms such as deep neural networks require a significant amount of data during training to avoid overfitting. These models often fail to detect minority samples when the input samples are imbalanced, which results in missed diagnoses of equipment faults. As an effective method to enhance minority samples, Deep Convolution Generative Adversarial Network (DCGAN) does not fundamentally address the problem of unstable Generative Adversarial Network (GAN) training This study proposes an improved DCGAN model with improved stability and sample balance for achieving greater classification accuracy over minority samples. First, spectral normalization is performed on each convolutional layer, improving stability in the DCGAN discriminator. Then, the improved DCGAN model is trained to generate new samples that are different from the original samples but with a similar distribution when the Nash equilibrium is reached. Four indices-Inception Score (IS), Frechet Inception Distance Score (FID), Peak Signal to Noise Ratio (PSNR), and Structural Similarity (SSIM)-were used to quantitatively evaluate of the generated images. Finally, the Balance Degree of Samples (BDS) index was proposed, and the new samples are proportionally added to the original samples to improve sample balance, resulting in the formation of several groups of datasets with different balance degrees, and Convolutional Neural Network (CNN) models are used to classify these samples. With experimental analysis on the reciprocating compressor, the variance of lost data is found to be less than 1% of the original value, representing an increase in stability of the model to generate diverse and high-quality sample images, as compared with that of the unmodified model. The classification accuracy exceeds 95% and tends to remain stable when the balance degree of samples is greater than 80%.
更多查看译文
关键词
Imbalanced, data enhancement, fault diagnosis, DCGAN, CNN
AI 理解论文
溯源树
样例
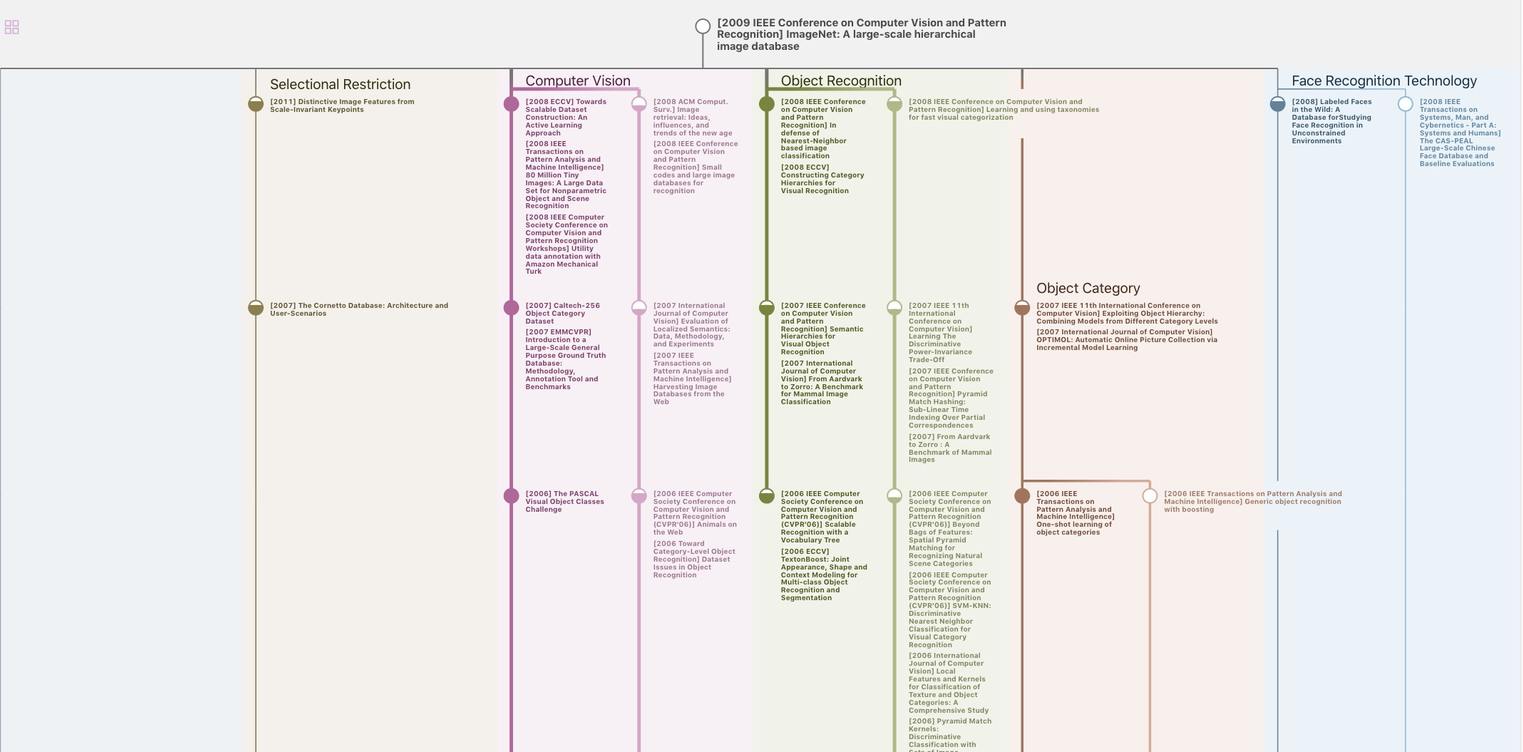
生成溯源树,研究论文发展脉络
Chat Paper
正在生成论文摘要