Predicting compound amenability with liquid chromatography-mass spectrometry to improve non-targeted analysis
ANALYTICAL AND BIOANALYTICAL CHEMISTRY(2021)
摘要
With the increasing availability of high-resolution mass spectrometers, suspect screening and non-targeted analysis are becoming popular compound identification tools for environmental researchers. Samples of interest often contain a large (unknown) number of chemicals spanning the detectable mass range of the instrument. In an effort to separate these chemicals prior to injection into the mass spectrometer, a chromatography method is often utilized. There are numerous types of gas and liquid chromatographs that can be coupled to commercially available mass spectrometers. Depending on the type of instrument used for analysis, the researcher is likely to observe a different subset of compounds based on the amenability of those chemicals to the selected experimental techniques and equipment. It would be advantageous if this subset of chemicals could be predicted prior to conducting the experiment, in order to minimize potential false-positive and false-negative identifications. In this work, we utilize experimental datasets to predict the amenability of chemical compounds to detection with liquid chromatography-electrospray ionization-mass spectrometry (LC–ESI–MS). The assembled dataset totals 5517 unique chemicals either explicitly detected or not detected with LC–ESI–MS. The resulting detected/not-detected matrix has been modeled using specific molecular descriptors to predict which chemicals are amenable to LC–ESI–MS, and to which form(s) of ionization. Random forest models, including a measure of the applicability domain of the model for both positive and negative modes of the electrospray ionization source, were successfully developed. The outcome of this work will help to inform future suspect screening and non-targeted analyses of chemicals by better defining the potential LC–ESI–MS detectable chemical landscape of interest. Graphical abstract
更多查看译文
关键词
Non-targeted analysis, Suspect screening analysis, Mass spectrometry, Machine learning, Random forest, Predictive modeling
AI 理解论文
溯源树
样例
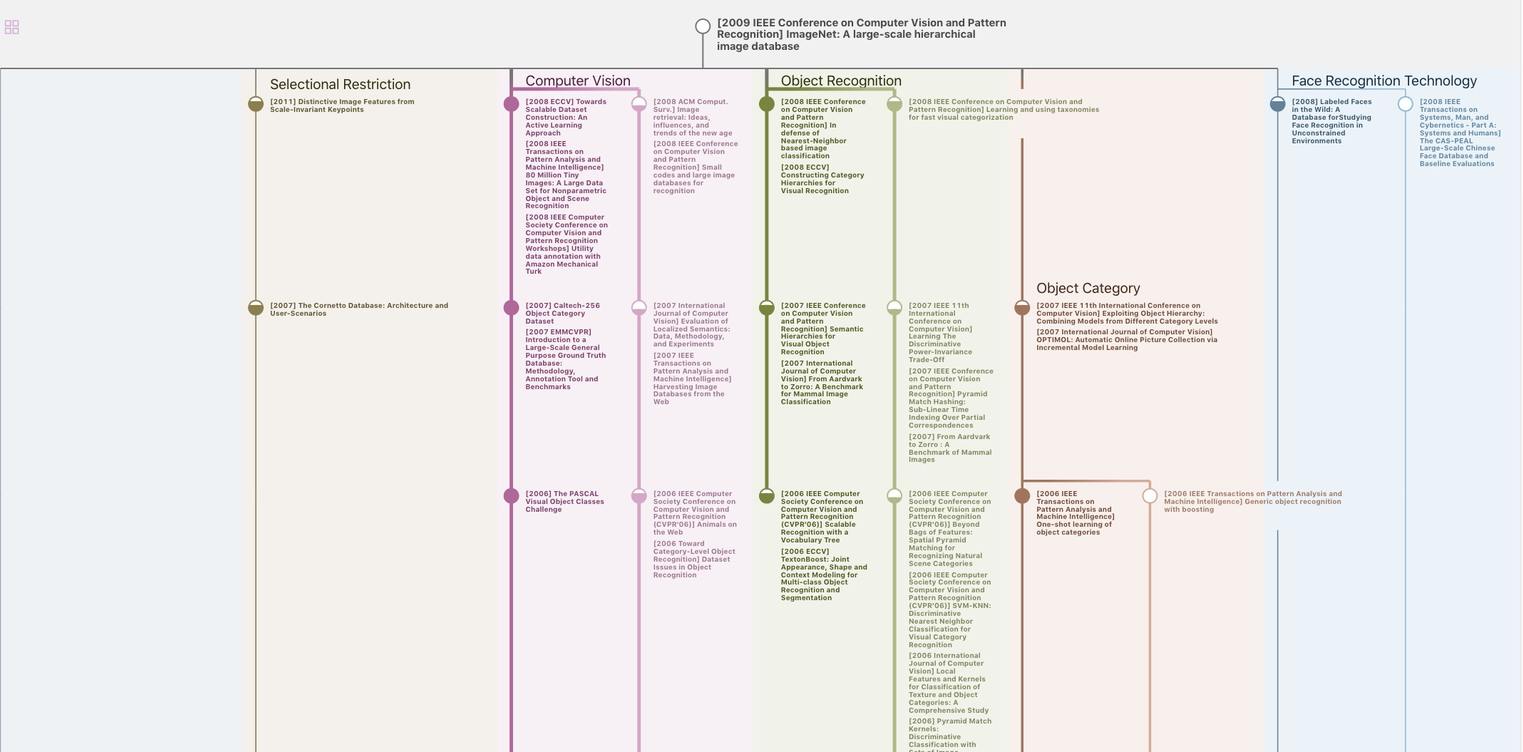
生成溯源树,研究论文发展脉络
Chat Paper
正在生成论文摘要