Building Footprint Generation Through Convolutional Neural Networks With Attraction Field Representation
IEEE TRANSACTIONS ON GEOSCIENCE AND REMOTE SENSING(2022)
摘要
Building footprint generation is a vital task in a wide range of applications, including, to name a few, land use management, urban planning and monitoring, and geographical database updating. Most existing approaches addressing this problem fall back on convolutional neural networks (CNNs) to learn semantic masks of buildings. However, one limitation of their results is blurred building boundaries. To address this, we propose to learn attraction field representation for building boundaries, which is capable of providing an enhanced representation power. Our method comprises two elemental modules: an Img2AFM module and an AFM2Mask module. More specifically, the former aims at learning an attraction field representation conditioned on an input image, which is capable of enhancing building boundaries and suppressing the background. The latter module predicts segmentation masks of buildings using the learned attraction field map. The proposed method is evaluated on three datasets with different spatial resolutions: the ISPRS dataset, the INRIA dataset, and the Planet dataset. From experimental results, we find that the proposed framework can well preserve geometric shapes and sharp boundaries of buildings, which brings significant improvements over other competitors. The trained model and code are available at https://github.com/lqycrystal/AFM_building.
更多查看译文
关键词
Buildings, Semantics, Image segmentation, Remote sensing, Feature extraction, Convolutional neural networks, Task analysis, Attraction field map (AFM), building footprint, convolutional neural network (CNN), semantic segmentation
AI 理解论文
溯源树
样例
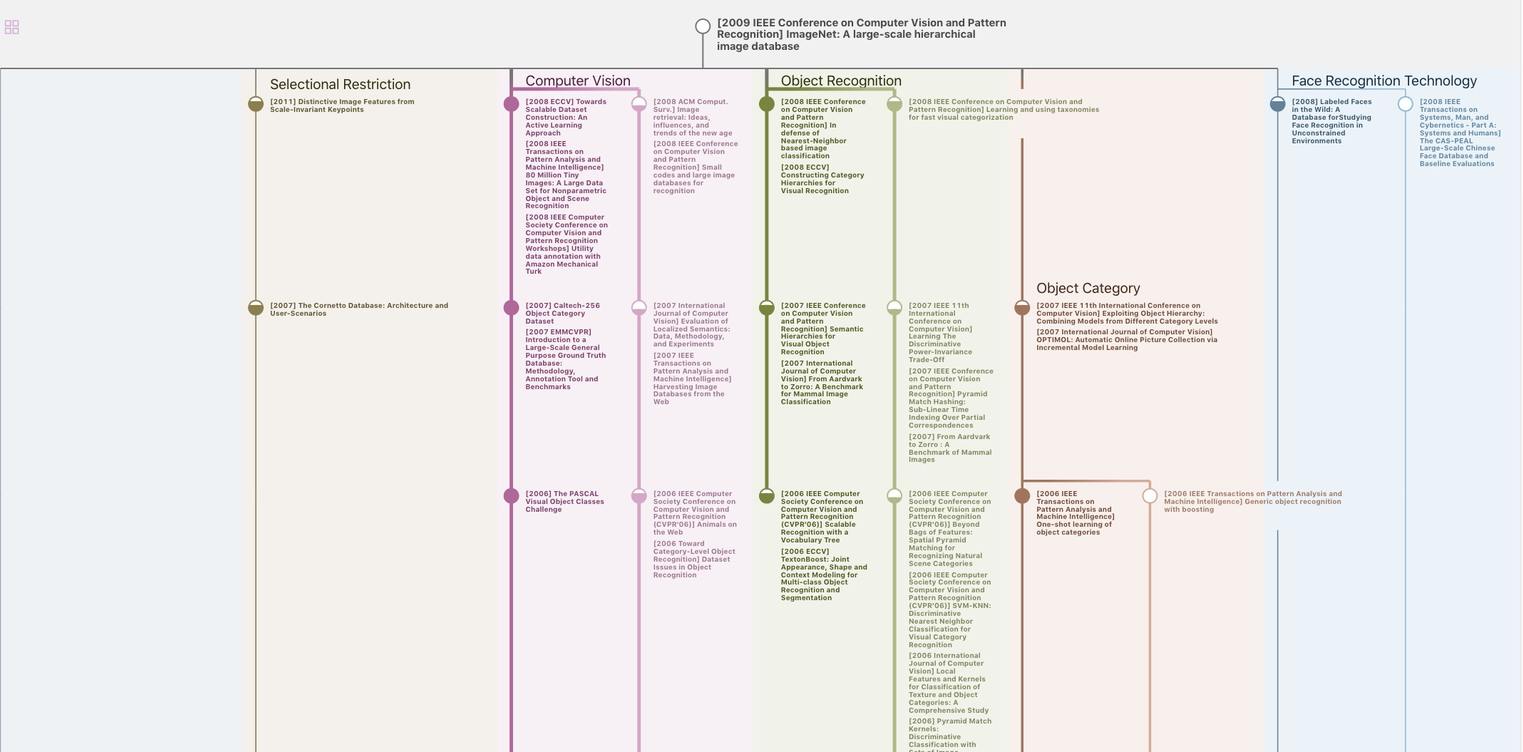
生成溯源树,研究论文发展脉络
Chat Paper
正在生成论文摘要