Fast data series indexing for in-memory data
The VLDB Journal(2021)
摘要
Data series similarity search is a core operation for several data series analysis applications across many different domains. However, the state-of-the-art techniques fail to deliver the time performance required for interactive exploration, or analysis of large data series collections. In this work, we propose MESSI, the first data series index designed for in-memory operation on modern hardware. Our index takes advantage of the modern hardware parallelization opportunities (i.e., SIMD instructions, multi-socket and multi-core architectures), in order to accelerate both index construction and similarity search processing times. Moreover, it benefits from a careful design in the setup and coordination of the parallel workers and data structures, so that it maximizes its performance for in-memory operations. MESSI supports similarity search using both the Euclidean and dynamic time warping (DTW) distances. Our experiments with synthetic and real datasets demonstrate that overall MESSI is up to 4x faster at index construction and up to 11x faster at query answering than the state-of-the-art parallel approach. MESSI is the first to answer exact similarity search queries on 100GB datasets in ∼ 50 ms (30–75 ms across diverse datasets), which enables real-time, interactive data exploration on very large data series collections.
更多查看译文
关键词
Data series, Indexing, Modern hardware
AI 理解论文
溯源树
样例
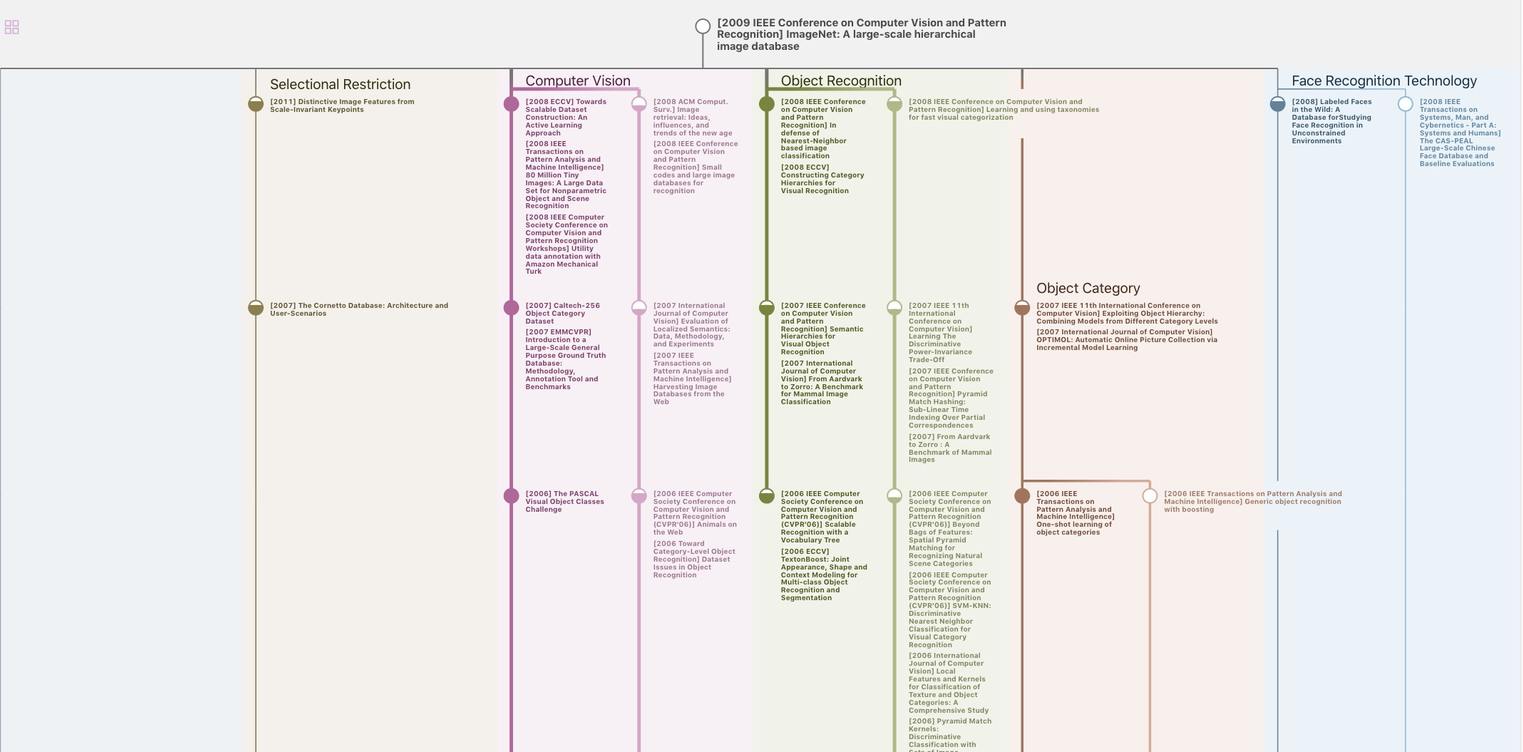
生成溯源树,研究论文发展脉络
Chat Paper
正在生成论文摘要