Omni-Training: Bridging Pre-Training and Meta-Training for Few-Shot Learning
IEEE TRANSACTIONS ON PATTERN ANALYSIS AND MACHINE INTELLIGENCE(2023)
摘要
Few-shot learning aims to fast adapt a deep model from a few examples. While pre-training and meta-training can create deep models powerful for few-shot generalization, we find that pre-training and meta-training focus respectively on cross-domain transferability and cross-task transferability, which restricts their data efficiency in the entangled settings of domain shift and task shift. We thus propose the Omni-Training framework to seamlessly bridge pre-training and meta-training for data-efficient few-shot learning. Our first contribution is a tri-flow Omni-Net architecture. Besides the joint representation flow, Omni-Net introduces two parallel flows for pre-training and meta-training, responsible for improving domain transferability and task transferability respectively. Omni-Net further coordinates the parallel flows by routing their representations via the joint-flow, enabling knowledge transfer across flows. Our second contribution is the Omni-Loss, which introduces a self-distillation strategy separately on the pre-training and meta-training objectives for boosting knowledge transfer throughout different training stages. Omni-Training is a general framework to accommodate many existing algorithms. Evaluations justify that our single framework consistently and clearly outperforms the individual state-of-the-art methods on both cross-task and cross-domain settings in a variety of classification, regression and reinforcement learning problems.
更多查看译文
关键词
Task analysis,Training,Adaptation models,Data models,Feature extraction,Deep learning,Bridges,Few-shot learning,data efficiency,transferability,meta-learning,pre-training
AI 理解论文
溯源树
样例
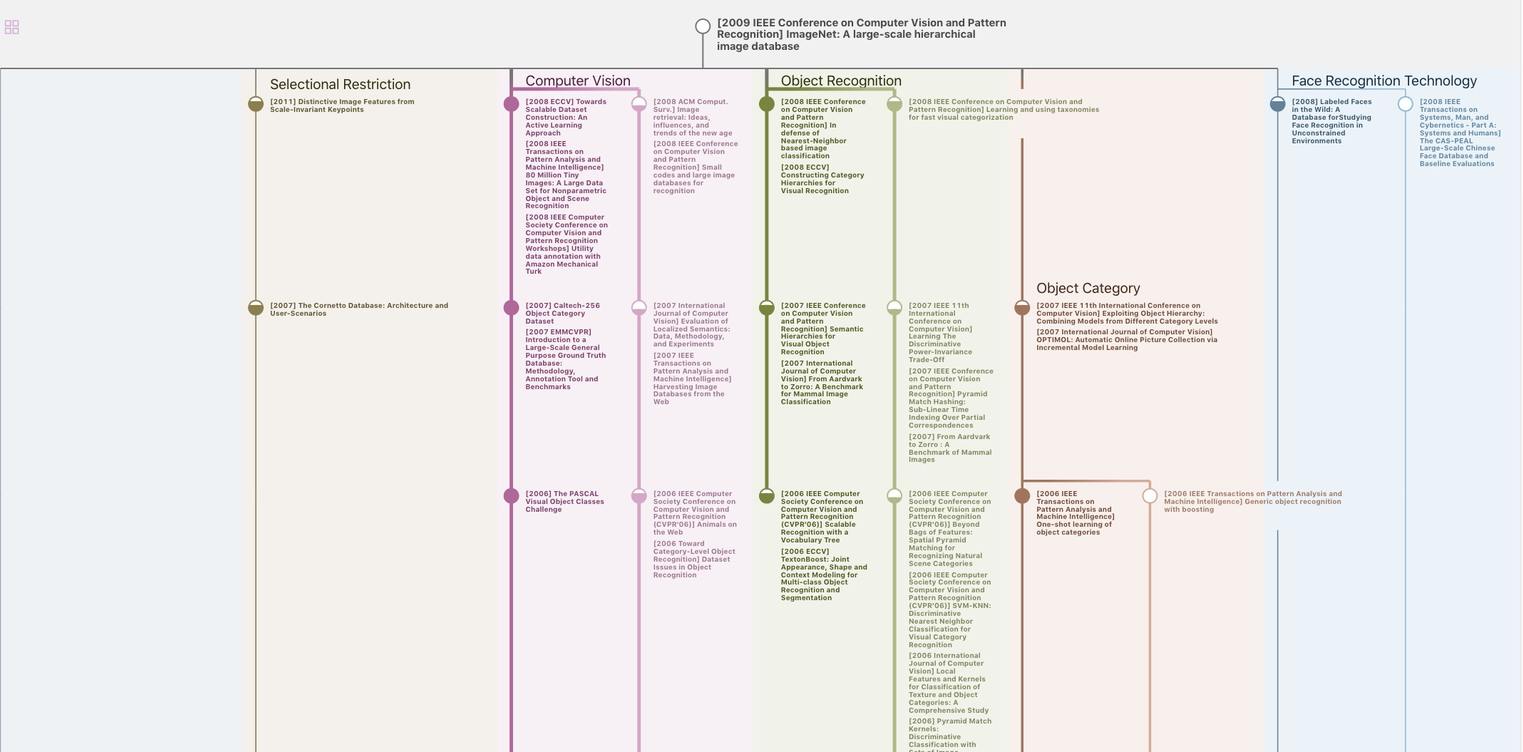
生成溯源树,研究论文发展脉络
Chat Paper
正在生成论文摘要