Sign and Relevance learning
arxiv(2022)
摘要
Standard models of biologically realistic, or inspired, reinforcement learning employ a global error signal which implies shallow networks. However, on the other hand, local learning rules allow networks with multiple layers. Here, we present a network combining local learning with global modulation where neuromodulation controls the amount of plasticity change in the whole network, while the sign of the error is passed via a bottom-up pathway through the network. Neuromodulation can be understood as a rectified error, or relevance, signal while the bottom-up sign of the error signal decides between long-term potentiation and long-term depression. We demonstrate the performance of this paradigm with a real robotic task as a proof of concept.
更多查看译文
关键词
relevance learning
AI 理解论文
溯源树
样例
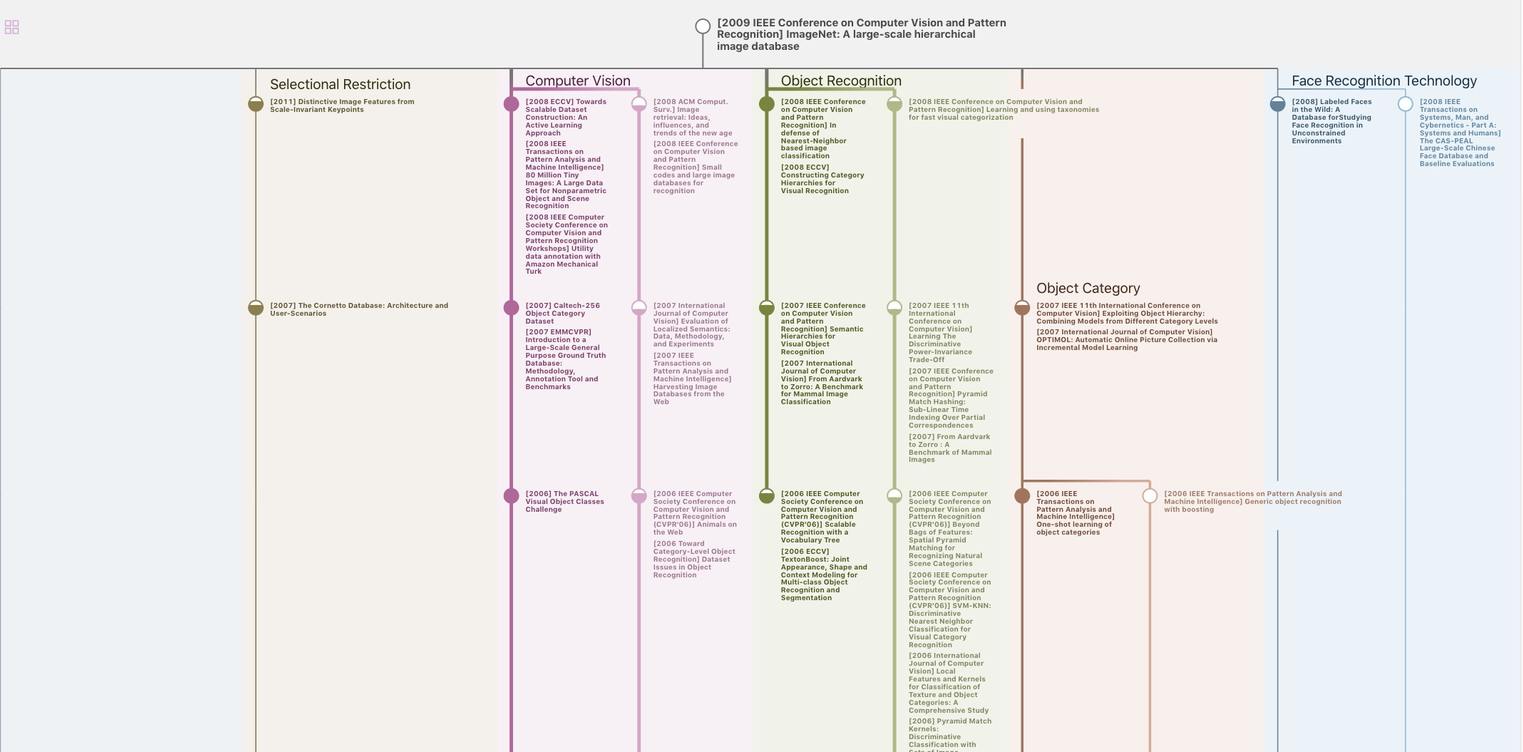
生成溯源树,研究论文发展脉络
Chat Paper
正在生成论文摘要