Semantically Distributed Robust Optimization for Vision-and-Language Inference
FINDINGS OF THE ASSOCIATION FOR COMPUTATIONAL LINGUISTICS (ACL 2022)(2022)
摘要
Analysis of vision-and-language models has revealed their brittleness under linguistic phenomena such as paraphrasing, negation, textual entailment, and word substitutions with synonyms or antonyms. While data augmentation techniques have been designed to mitigate against these failure modes, methods that can integrate this knowledge into the training pipeline remain under-explored. In this paper, we present SDRO+, a model-agnostic method that utilizes a set linguistic transformations in a distributed robust optimization setting, along with an ensembling technique to leverage these transformations during inference. Experiments on benchmark datasets with images (NLVR2) and video (VIOLIN) demonstrate performance improvements as well as robustness to adversarial attacks. Experiments on binary VQA explore the generalizability of this method to other V&L tasks.
更多查看译文
关键词
robust optimization,inference,vision-and-language
AI 理解论文
溯源树
样例
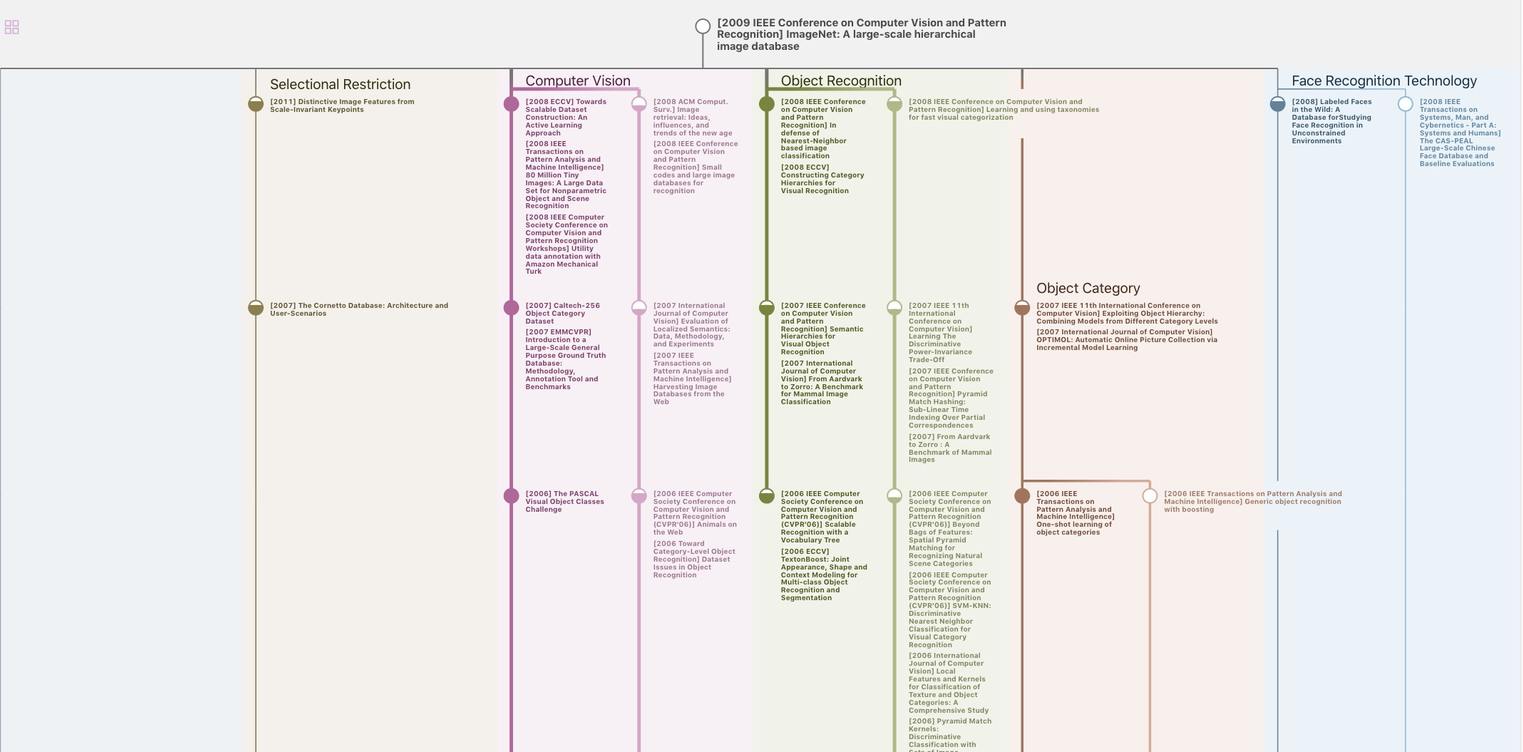
生成溯源树,研究论文发展脉络
Chat Paper
正在生成论文摘要