Variance Minimization in the Wasserstein Space for Invariant Causal Prediction
INTERNATIONAL CONFERENCE ON ARTIFICIAL INTELLIGENCE AND STATISTICS, VOL 151(2022)
摘要
Selecting powerful predictors for an outcome is a cornerstone task for machine learning. However, some types of questions can only be answered by identifying the predictors that causally affect the outcome. A recent approach to this causal inference problem leverages the invariance property of a causal mechanism across differing experimental environments (Peters et al., 2016; Heinze-Deml et al., 2018). This method, invariant causal prediction (ICP), has a substantial computational defect - the runtime scales exponentially with the number of possible causal variables. In this work, we show that the approach taken in ICP may be reformulated as a series of nonparametric tests that scales linearly in the number of predictors. Each of these tests relies on the minimization of a novel loss function - the Wasserstein variance - that is derived from tools in optimal transport theory and is used to quantify distributional variability across environments. We prove under mild assumptions that our method is able to recover the set of identifiable direct causes, and we demonstrate in our experiments that it is competitive with other benchmark causal discovery algorithms.
更多查看译文
关键词
invariant causal prediction,wasserstein space,variance
AI 理解论文
溯源树
样例
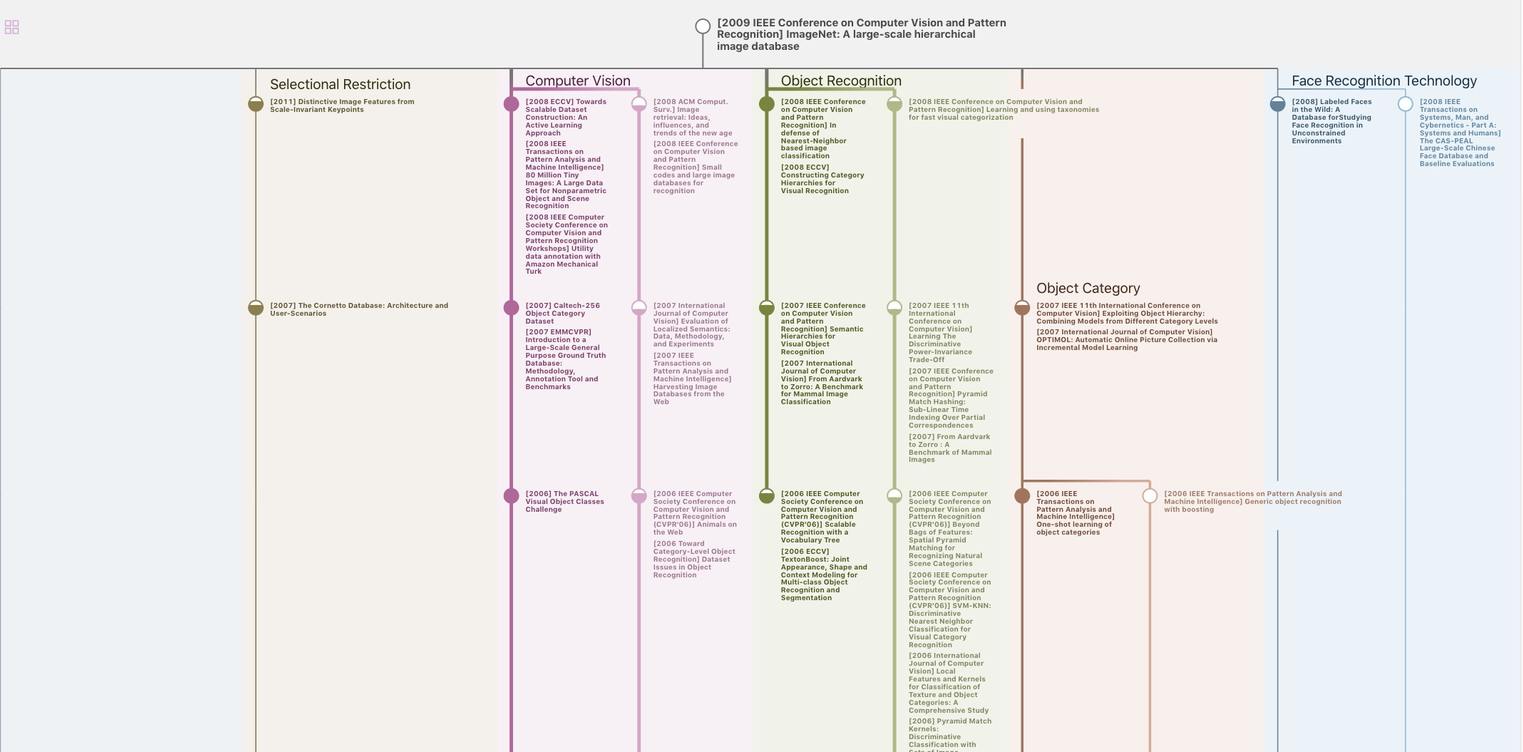
生成溯源树,研究论文发展脉络
Chat Paper
正在生成论文摘要