Towards Efficient NLP: A Standard Evaluation and A Strong Baseline
North American Chapter of the Association for Computational Linguistics (NAACL)(2022)
摘要
Supersized pre-trained language models have pushed the accuracy of various NLP tasks to a new state-of-the-art (SOTA). Rather than pursuing the reachless SOTA accuracy, most works are pursuing improvement on other dimensions such as efficiency, leading to "Pareto SOTA". Different from accuracy, the metric for efficiency varies across different studies, making them hard to be fairly compared. To that end, this work presents ELUE (Efficient Language Understanding Evaluation), a standard evaluation, and a public leaderboard for efficient NLP models. ELUE is dedicated to depicting the Pareto Front for various language understanding tasks, such that it can tell whether and how much a method achieves Pareto improvement. Along with the benchmark, we also pre-train and release a strong baseline, ElasticBERT, whose elasticity is both static and dynamic. ElasticBERT is static in that it allows reducing model layers on demand. ElasticBERT is dynamic in that it selectively executes parts of model layers conditioned on the input. We demonstrate the ElasticBERT, despite its simplicity, outperforms or performs on par with SOTA compressed and early exiting models. The ELUE benchmark is publicly available at http://eluebenchmark.fastnlp.top/.
更多查看译文
关键词
efficient nlp,standard evaluation
AI 理解论文
溯源树
样例
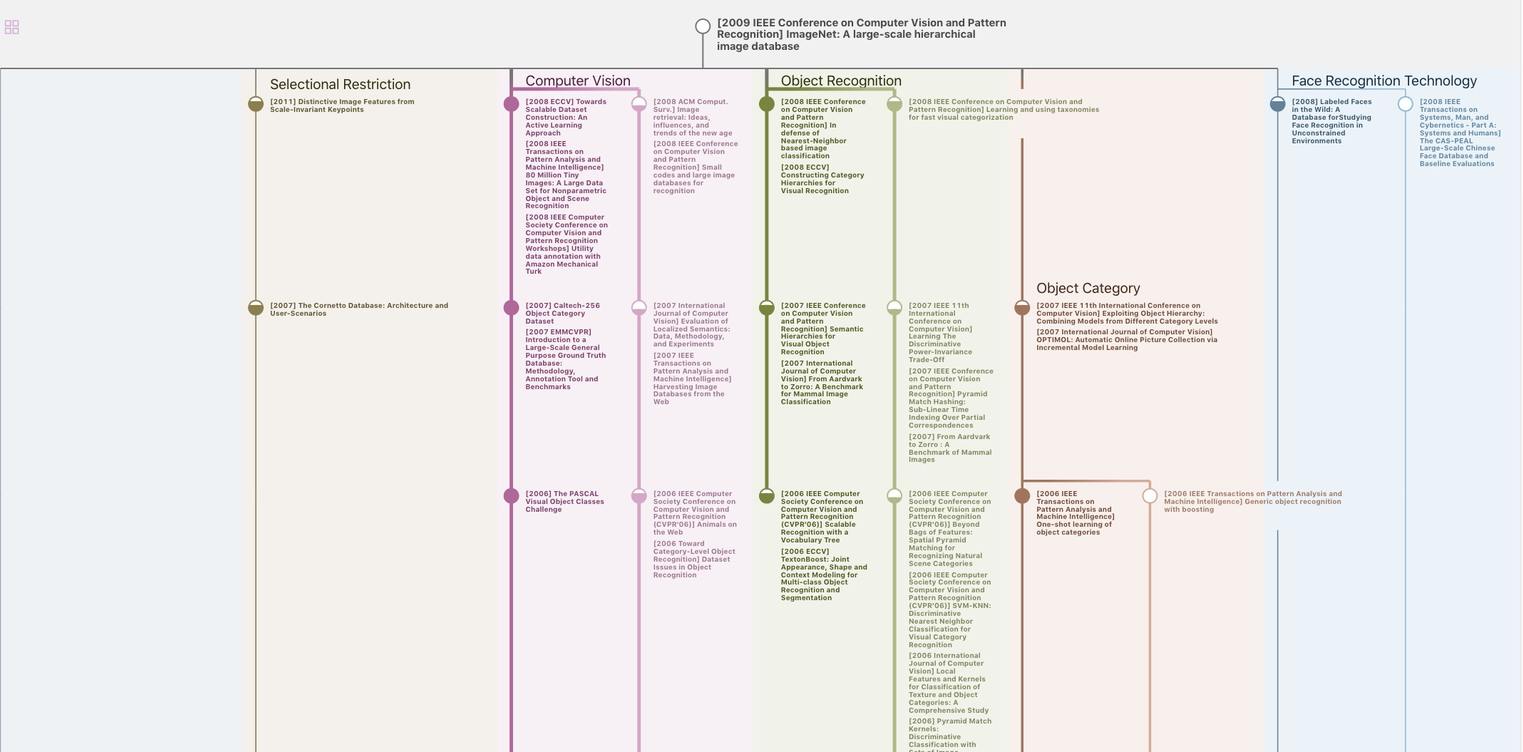
生成溯源树,研究论文发展脉络
Chat Paper
正在生成论文摘要