Considering User Agreement in Learning to Predict the Aesthetic Quality
IEEE International Conference on Acoustics, Speech, and Signal Processing (ICASSP)(2022)
摘要
How to robustly rank the aesthetic quality of given images has been a long-standing ill-posed topic. Such challenge stems mainly from the diverse subjective opinions of different observers about the varied types of content. There is a growing interest in estimating the user agreement by considering the standard deviation of the scores, instead of only predicting the mean aesthetic opinion score. Nevertheless, when comparing a pair of contents, few studies consider how confident are we regarding the difference in the aesthetic scores. In this paper, we thus propose (1) a re-adapted multi-task attention network to predict both the mean opinion score and the standard deviation in an end-to-end manner; (2) a brand-new confidence interval ranking loss that encourages the model to focus on image-pairs that are less certain about the difference of their aesthetic scores. With such loss, the model is encouraged to learn the uncertainty of the content that is relevant to the diversity of observers' opinions, i.e., user disagreement. Extensive experiments have demonstrated that the proposed multi-task aesthetic model achieves state-of-the-art performance on two different types of aesthetic datasets, i.e., AVA and TMGA.
更多查看译文
关键词
Aesthetic assessment,multi-task learning,observers' diversity of opinions,confidence interval loss
AI 理解论文
溯源树
样例
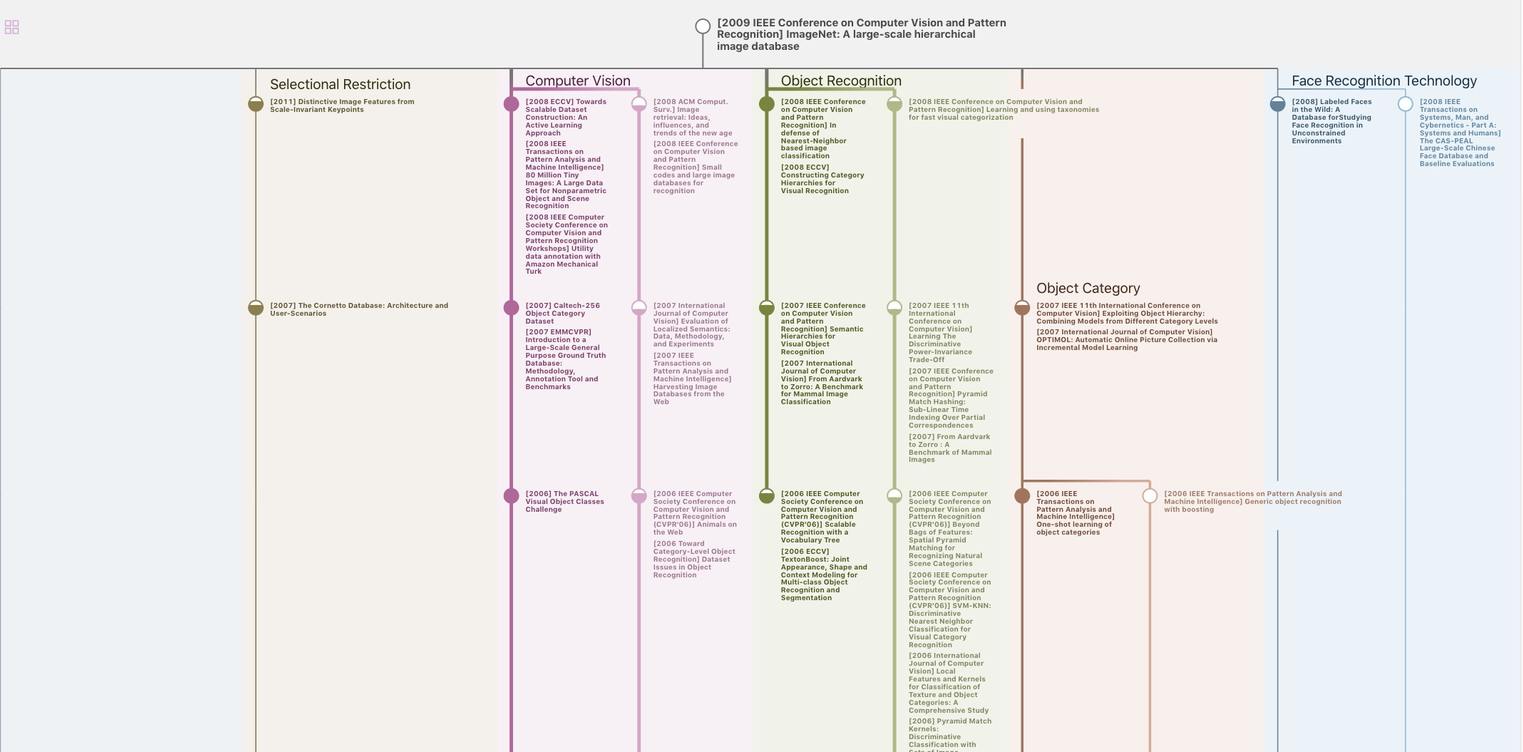
生成溯源树,研究论文发展脉络
Chat Paper
正在生成论文摘要