The Case for Latent Variable Vs Deep Learning Methods in Misinformation Detection - An Application to COVID-19.
DS(2021)
摘要
The detection and removal of misinformation from social media during high impact events, e.g., COVID-19 pandemic, is a sensitive application since the agency in charge of this process must ensure that no unwarranted actions are taken. This suggests that any automated system used for this process must display both high prediction accuracy as well as high explainability. Although Deep Learning methods have shown remarkable prediction accuracy, accessing the contextual information that Deep Learning-based representations carry is a significant challenge. In this paper, we propose a data-driven solution that is based on a popular latent variable model called Independent Component Analysis (ICA), where a slight loss in accuracy with respect to a BERT model is compensated by interpretable contextual representations. Our proposed solution provides direct interpretability without affecting the computational complexity of the model and without designing a separate system. We carry this study on a novel labeled COVID-19 Twitter dataset that is based on socio-linguistic criteria and show that our model's explanations highly correlate with humans' reasoning.
更多查看译文
关键词
Misinformation detection,Knowledge discovery,Independent Component Analysis,Explainability
AI 理解论文
溯源树
样例
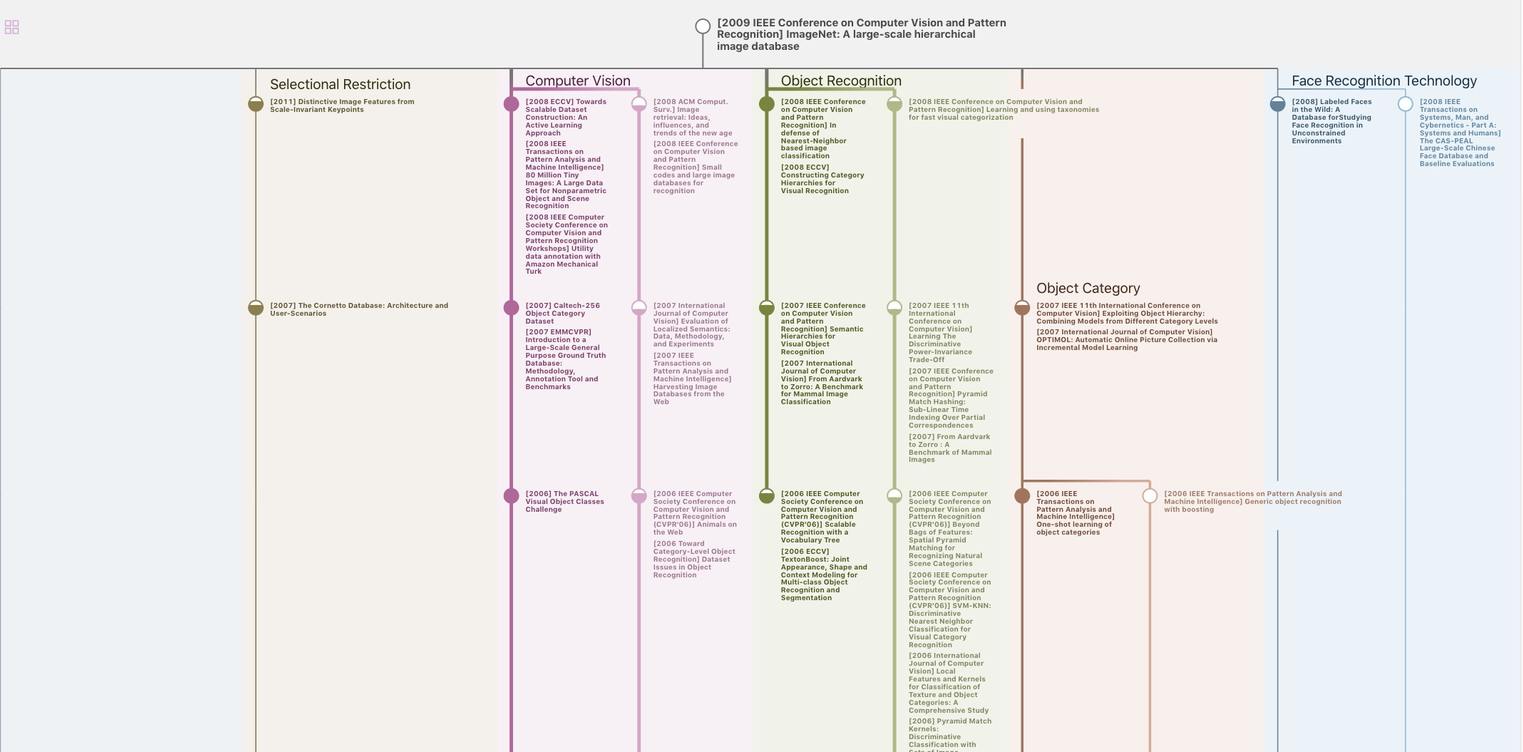
生成溯源树,研究论文发展脉络
Chat Paper
正在生成论文摘要