Uncertainty Estimation For Machine Learning Models In Multiphase Flow Applications
INFORMATICS-BASEL(2021)
摘要
In oil and gas production, it is essential to monitor some performance indicators that are related to the composition of the extracted mixture, such as the liquid and gas content of the flow. These indicators cannot be directly measured and must be inferred with other measurements by using soft sensor approaches that model the target quantity. For the purpose of production monitoring, point estimation alone is not enough, and a confidence interval is required in order to assess the uncertainty in the provided measure. Decisions based on these estimations can have a large impact on production costs; therefore, providing a quantification of uncertainty can help operators make the most correct choices. This paper focuses on the estimation of the performance indicator called the water-in-liquid ratio by using data-driven tools: firstly, anomaly detection techniques are employed to find data that can alter the performance of the subsequent model; then, different machine learning models, such as Gaussian processes, random forests, linear local forests, and neural networks, are tested and employed to perform uncertainty-aware predictions on data coming from an industrial tool, the multiphase flow meter, which collects multiple signals from the flow mixture. The reported results show the differences between the discussed approaches and the advantages of the uncertainty estimation; in particular, they show that methods such as the Gaussian process and linear local forest are capable of reaching competitive performance in terms of both RMSE (1.9-2.1) and estimated uncertainty (1.6-2.6).
更多查看译文
关键词
Bayesian inference, confidence interval, machine learning, multiphase flow, uncertainty estimation
AI 理解论文
溯源树
样例
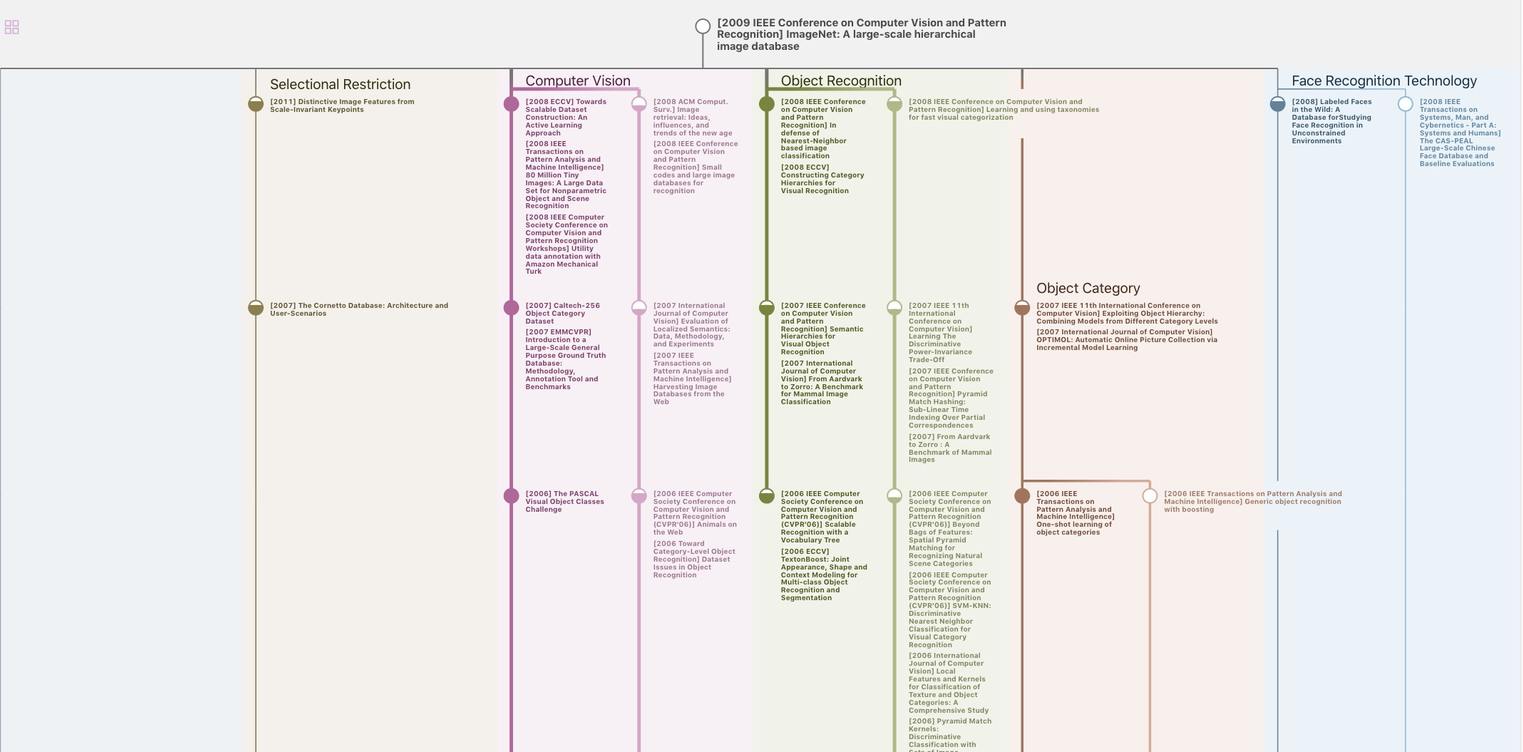
生成溯源树,研究论文发展脉络
Chat Paper
正在生成论文摘要