Estimation of Mechanical Power Output Employing Deep Learning on Inertial Measurement Data in Roller Ski Skating
SENSORS(2021)
摘要
The ability to optimize power generation in sports is imperative, both for understanding and balancing training load correctly, and for optimizing competition performance. In this paper, we aim to estimate mechanical power output by employing a time-sequential information-based deep Long Short-Term Memory (LSTM) neural network from multiple inertial measurement units (IMUs). Thirteen athletes conducted roller ski skating trials on a treadmill with varying incline and speed. The acceleration and gyroscope data collected with the IMUs were run through statistical feature processing, before being used by the deep learning model to estimate power output. The model was thereafter used for prediction of power from test data using two approaches. First, a user-dependent case was explored, reaching a power estimation within 3.5% error. Second, a user-independent case was developed, reaching an error of 11.6% for the power estimation. Finally, the LSTM model was compared to two other machine learning models and was found to be superior. In conclusion, the user-dependent model allows for precise estimation of roller skiing power output after training the model on data from each athlete. The user-independent model provides less accurate estimation; however, the accuracy may be sufficient for providing valuable information for recreational skiers.
更多查看译文
关键词
cross country skiing, IMU, wearable sensors, LSTM, neural network
AI 理解论文
溯源树
样例
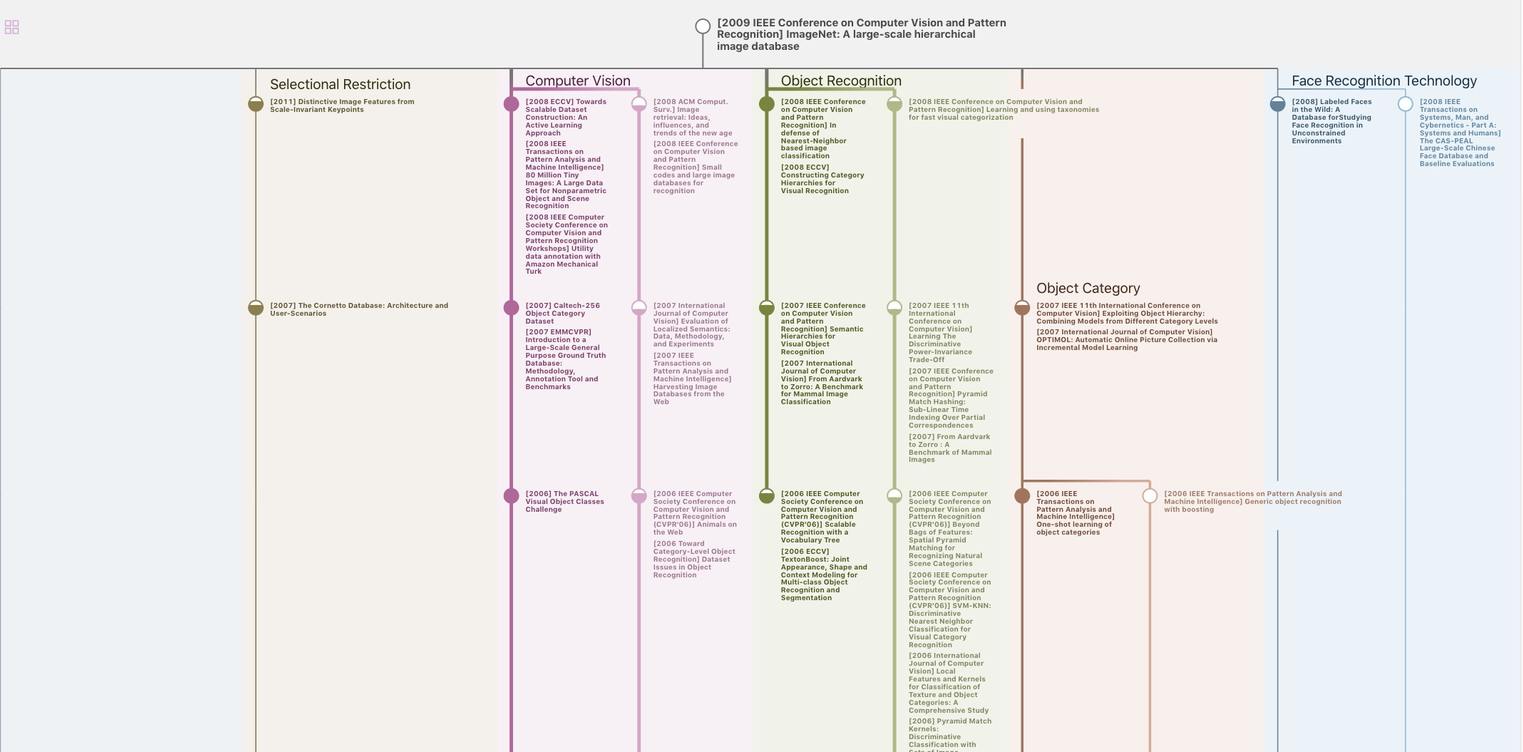
生成溯源树,研究论文发展脉络
Chat Paper
正在生成论文摘要