Collaborative Semantic Aggregation and Calibration for Federated Domain Generalization
arxiv(2023)
摘要
Domain generalization (DG) aims to learn from multiple known source domains a model that can generalize well to unknown target domains. The existing DG methods usually exploit the fusion of shared multi-source data to train a generalizable model. However, tremendous data is distributed across lots of places nowadays that can not be shared due to privacy policies. In this paper, we tackle the problem of federated domain generalization where the source datasets can only be accessed and learned locally for privacy protection. We propose a novel framework called Collaborative Semantic Aggregation and Calibration (CSAC) to enable this challenging problem. To fully absorb multi-source semantic information while avoiding unsafe data fusion, we conduct data-free semantic aggregation by fusing the models trained on the separated domains layer-by-layer. To address the semantic dislocation problem caused by domain shift, we further design cross-layer semantic calibration with an attention mechanism to align each semantic level and enhance domain invariance. We unify multi-source semantic learning and alignment in a collaborative way by repeating the semantic aggregation and calibration alternately, keeping each dataset localized, and the data privacy is carefully protected. Extensive experiments show the significant performance of our method in addressing this challenging problem.
更多查看译文
关键词
federated domain generalization,collaborative semantic aggregation
AI 理解论文
溯源树
样例
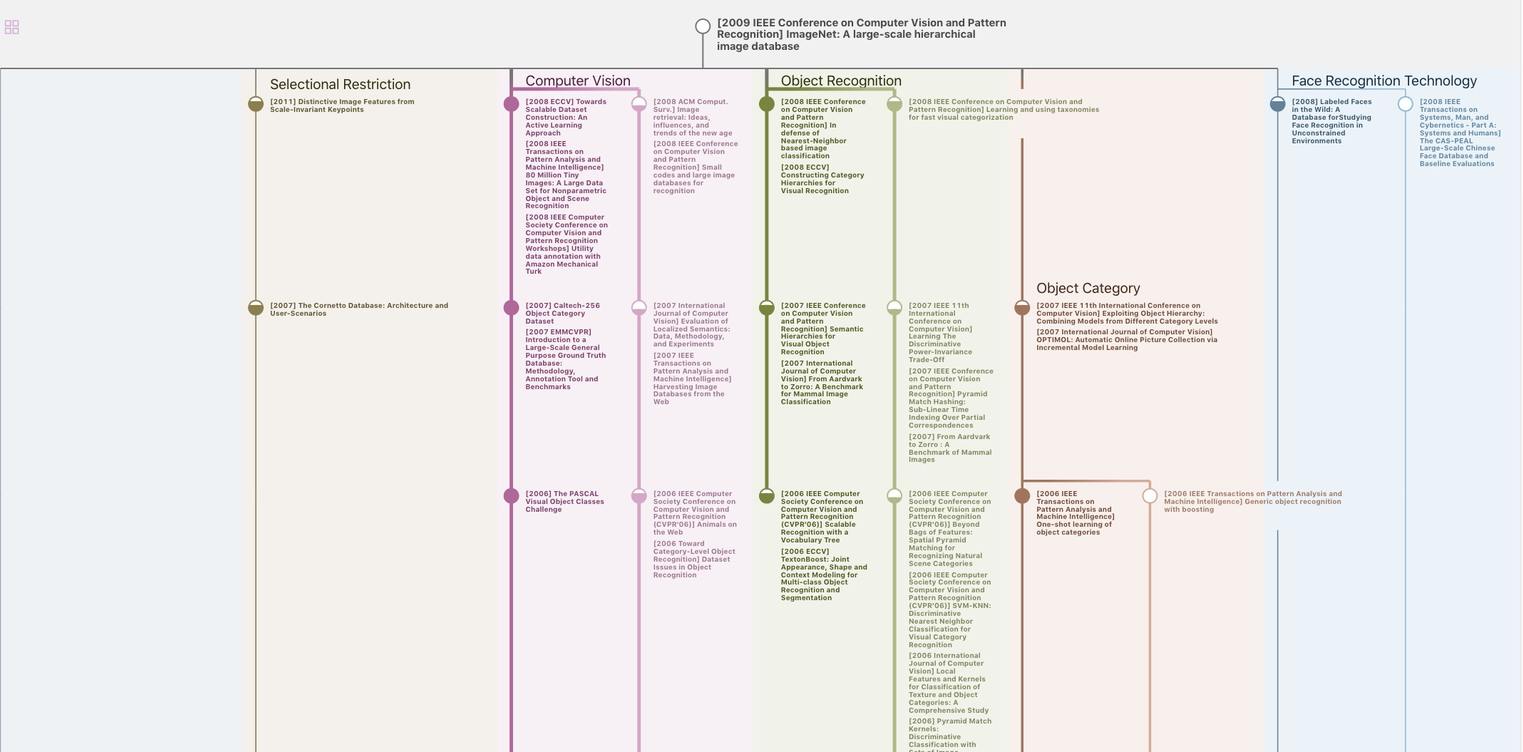
生成溯源树,研究论文发展脉络
Chat Paper
正在生成论文摘要