THOMAS: Trajectory Heatmap Output with learned Multi-Agent Sampling
International Conference on Learning Representations (ICLR)(2021)
摘要
In this paper, we propose THOMAS, a joint multi-agent trajectory prediction framework allowing for efficient and consistent prediction of multi-agent multi-modal trajectories. We present a unified model architecture for fast and simultaneous agent future heatmap estimation leveraging hierarchical and sparse image generation. We demonstrate that heatmap output enables a higher level of control on the predicted trajectories compared to vanilla multi-modal trajectory regression, allowing to incorporate additional constraints for tighter sampling or collision-free predictions in a deterministic way. However, we also highlight that generating scene-consistent predictions goes beyond the mere generation of collision-free trajectories. We therefore propose a learnable trajectory recombination model that takes as input a set of predicted trajectories for each agent and outputs its consistent reordered recombination. We report our results on the Interaction multi-agent prediction challenge and rank $1^{st}$ on the online test leaderboard.
更多查看译文
关键词
Trajectory prediction,Multi-agent,Motion forecasting,Motion estimation,Autonomous driving
AI 理解论文
溯源树
样例
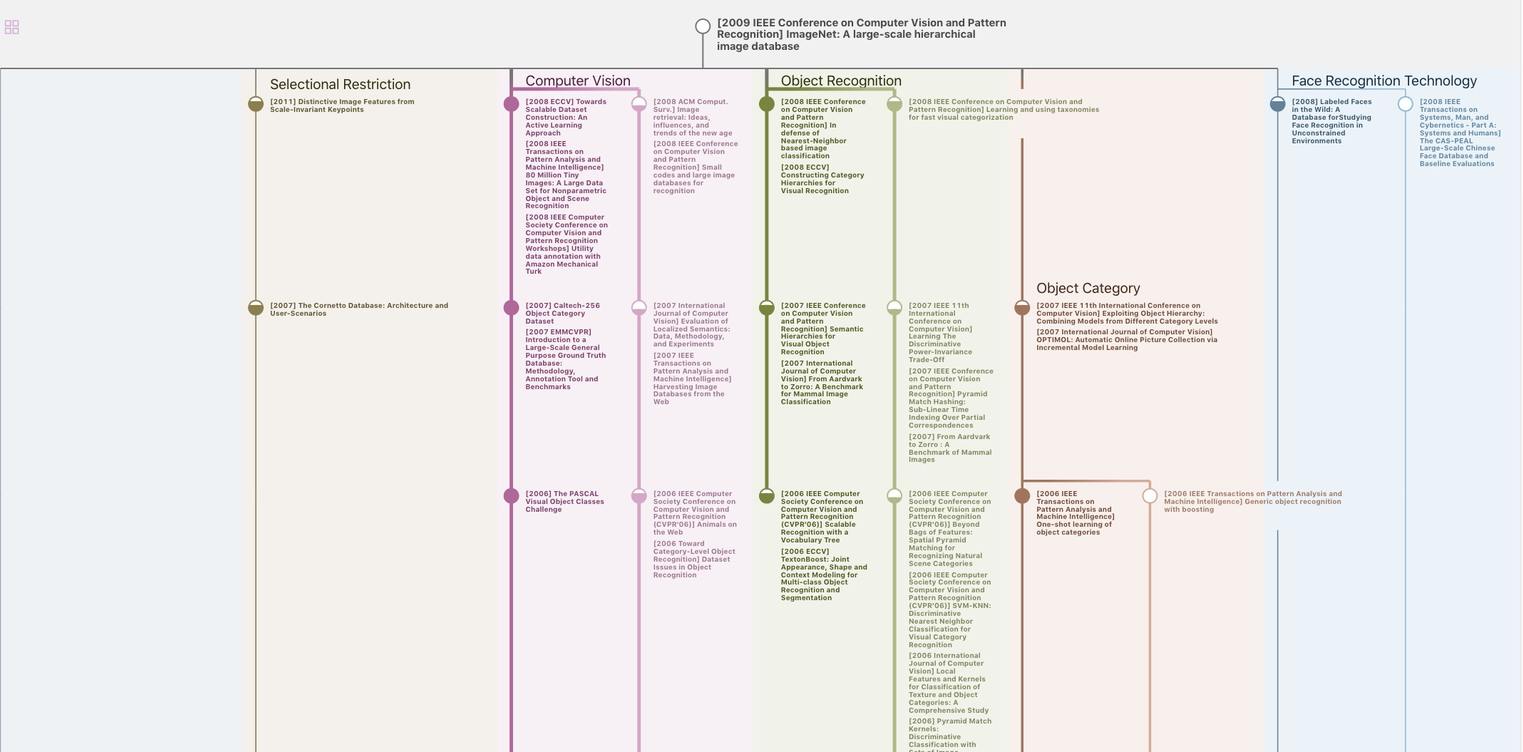
生成溯源树,研究论文发展脉络
Chat Paper
正在生成论文摘要