Revisiting Latent-Space Interpolation via a Quantitative Evaluation Framework
arxiv(2021)
摘要
Latent-space interpolation is commonly used to demonstrate the generalization ability of deep latent variable models. Various algorithms have been proposed to calculate the best trajectory between two encodings in the latent space. In this work, we show how data labeled with semantically continuous attributes can be utilized to conduct a quantitative evaluation of latent-space interpolation algorithms, for variational autoencoders. Our framework can be used to complement the standard qualitative comparison, and also enables evaluation for domains (such as graph) in which the visualization is difficult. Interestingly, our experiments reveal that the superiority of interpolation algorithms could be domain-dependent. While normalised interpolation works best for the image domain, spherical linear interpolation achieves the best performance in the graph domain. Next, we propose a simple-yet-effective method to restrict the latent space via a bottleneck structure in the encoder. We find that all interpolation algorithms evaluated in this work can benefit from this restriction. Finally, we conduct interpolation-aware training with the labeled attributes, and show that this explicit supervision can improve the interpolation performance.
更多查看译文
关键词
quantitative evaluation framework,quantitative evaluation,latent-space
AI 理解论文
溯源树
样例
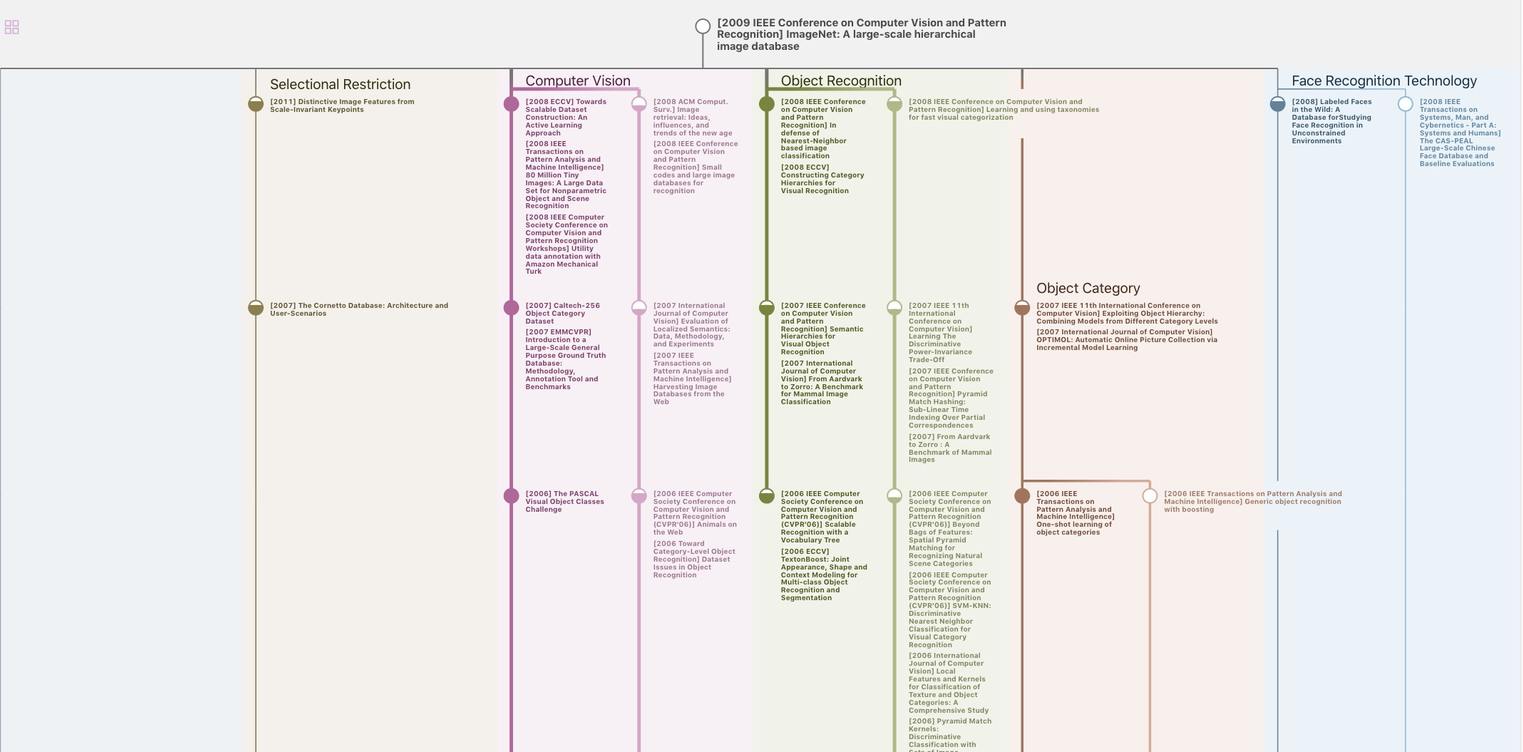
生成溯源树,研究论文发展脉络
Chat Paper
正在生成论文摘要