Open-Set Recognition: A Good Closed-Set Classifier is All You Need
International Conference on Learning Representations (ICLR)(2022)
摘要
The ability to identify whether or not a test sample belongs to one of the semantic classes in a classifier's training set is critical to practical deployment of the model. This task is termed open-set recognition (OSR) and has received significant attention in recent years. In this paper, we first demonstrate that the ability of a classifier to make the 'none-of-above' decision is highly correlated with its accuracy on the closed-set classes. We find that this relationship holds across loss objectives and architectures, and further demonstrate the trend both on the standard OSR benchmarks as well as on a large-scale ImageNet evaluation. Second, we use this correlation to boost the performance of the cross-entropy OSR 'baseline' by improving its closed-set accuracy, and with this strong baseline achieve a new state-of-the-art on the most challenging OSR benchmark. Similarly, we boost the performance of the existing state-of-the-art method by improving its closed-set accuracy, but this does not surpass the strong baseline on the most challenging dataset. Our third contribution is to reappraise the datasets used for OSR evaluation, and construct new benchmarks which better respect the task of detecting semantic novelty, as opposed to low-level distributional shifts as tackled by neighbouring machine learning fields. In this new setting, we again demonstrate that there is negligible difference between the strong baseline and the existing state-of-the-art.
更多查看译文
关键词
open set recognition,image recognition,computer vision
AI 理解论文
溯源树
样例
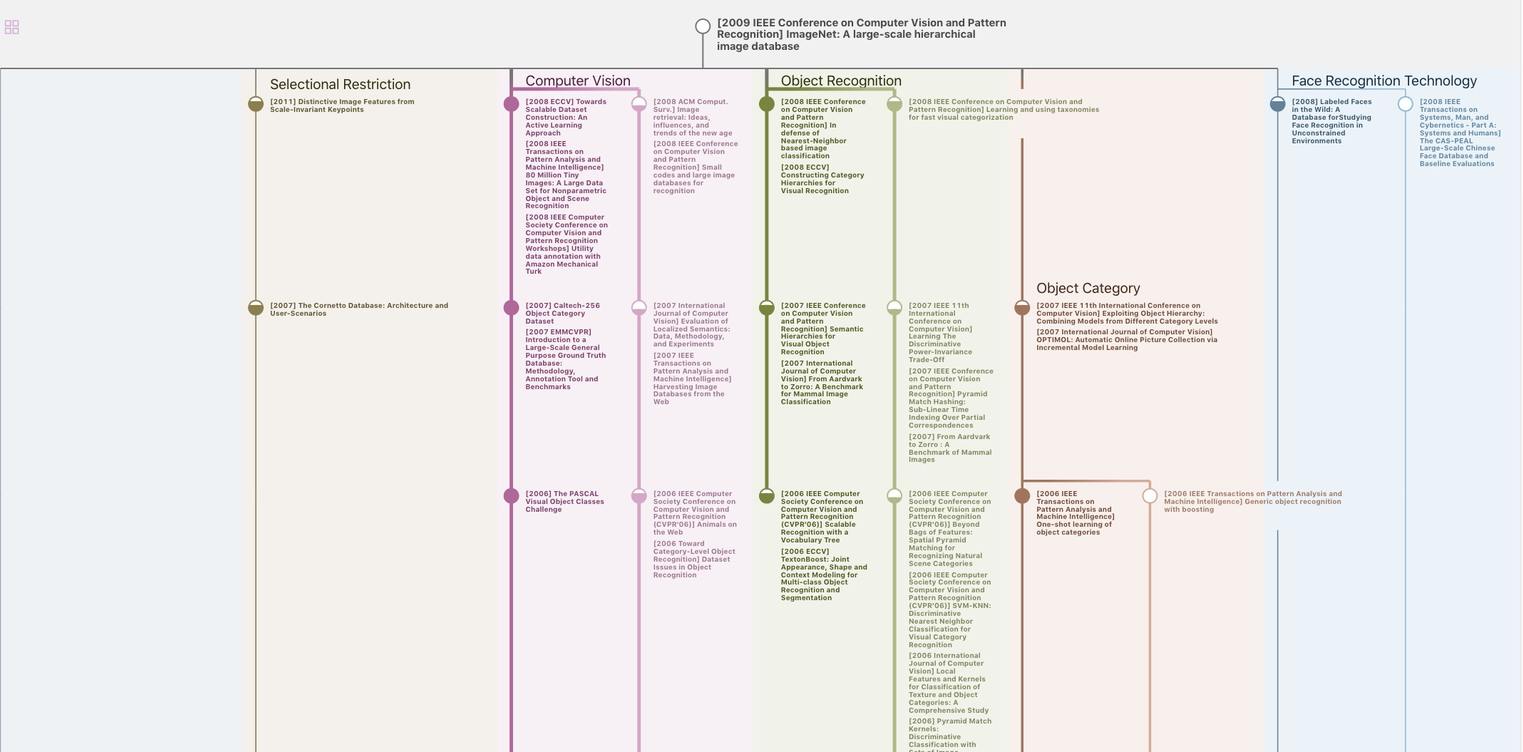
生成溯源树,研究论文发展脉络
Chat Paper
正在生成论文摘要