Deep Federated Learning for Autonomous Driving
2022 IEEE INTELLIGENT VEHICLES SYMPOSIUM (IV)(2022)
摘要
Autonomous driving is an active research topic in both academia and industry. However, most of the existing solutions focus on improving the accuracy by training learnable models with centralized large-scale data. Therefore, these methods do not take into account the user's privacy. In this paper, we present a new approach to learn autonomous driving policy while respecting privacy concerns. We propose a peer-to-peer Deep Federated Learning (DFL) approach to train deep architectures in a fully decentralized manner and remove the need for central orchestration. We design a new Federated Autonomous Driving network (FADNet) that can improve the model stability, ensure convergence, and handle imbalanced data distribution problems while is being trained with federated learning methods. Intensively experimental results on three datasets show that our approach with FADNet and DFL achieves superior accuracy compared with other recent methods. Furthermore, our approach can maintain privacy by not collecting user data to a central server. Our source code can be found at: https://github.com/aioz-ai/FADNet
更多查看译文
关键词
autonomous driving,learning
AI 理解论文
溯源树
样例
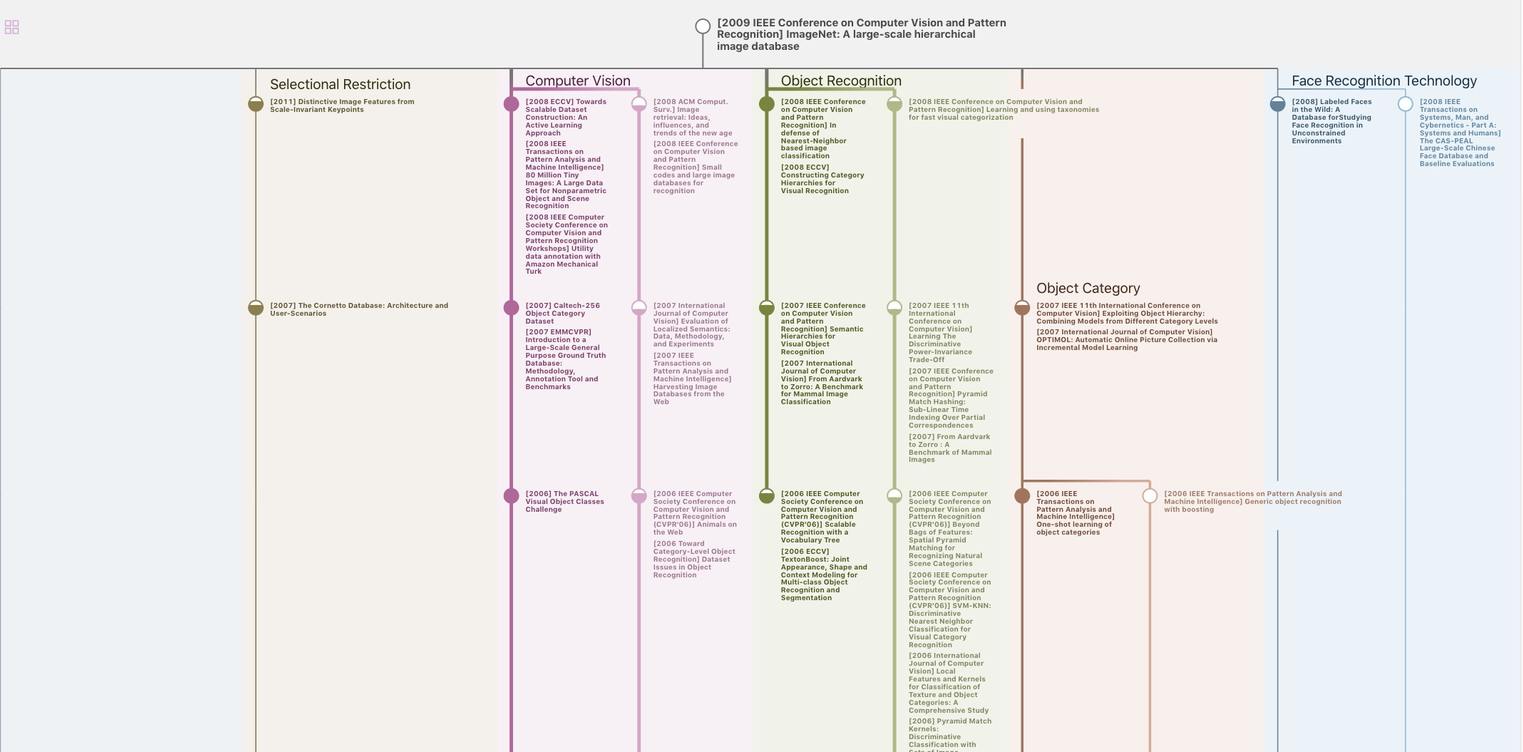
生成溯源树,研究论文发展脉络
Chat Paper
正在生成论文摘要