Kernel learning for intra-hour solar forecasting with infrared sky images and cloud dynamic feature extraction
Renewable and Sustainable Energy Reviews(2023)
摘要
Power grid operators incur additional costs to guarantee a reliable energy supply due to the uncertainty of the energy generated by photovoltaic systems. These additional costs are derived from the need for energy storage or increasing the planning reserve margin requirements. This investigation aims to decrease the costs by introducing a multi-task intra-hour solar forecast (feasible in real-time applications) to optimize energy dispatch and increase the participation of photovoltaics in power grids. The proposed method estimates the motion of clouds in a sequence of consecutive sky images by extracting features of cloud dynamics to forecast the global horizontal irradiance reaching a photovoltaic system. The sky images are acquired using a low-cost infrared sky imager mounted on a solar tracker. The solar forecasting algorithm is based on kernel learning methods and uses the clear sky index as the response variable and features extracted from clouds as covariates. The proposed algorithm achieved 16.48% forecasting skill 8 min ahead with a resolution of 1 min. Previous work reached 15.4% forecasting skill with 1 min resolution. Additionally, this investigation evaluates and compares the performances of multi-task Bayesian learning methods which provide a probabilistic forecast. The proposed solar forecasting algorithm can potentially assist grid operators in managing the inherent uncertainties of power grids with a high participation of solar energy resources.
更多查看译文
关键词
Flow visualization,Girasol dataset,Kernel learning,Machine learning,Solar forecasting,Sky imaging
AI 理解论文
溯源树
样例
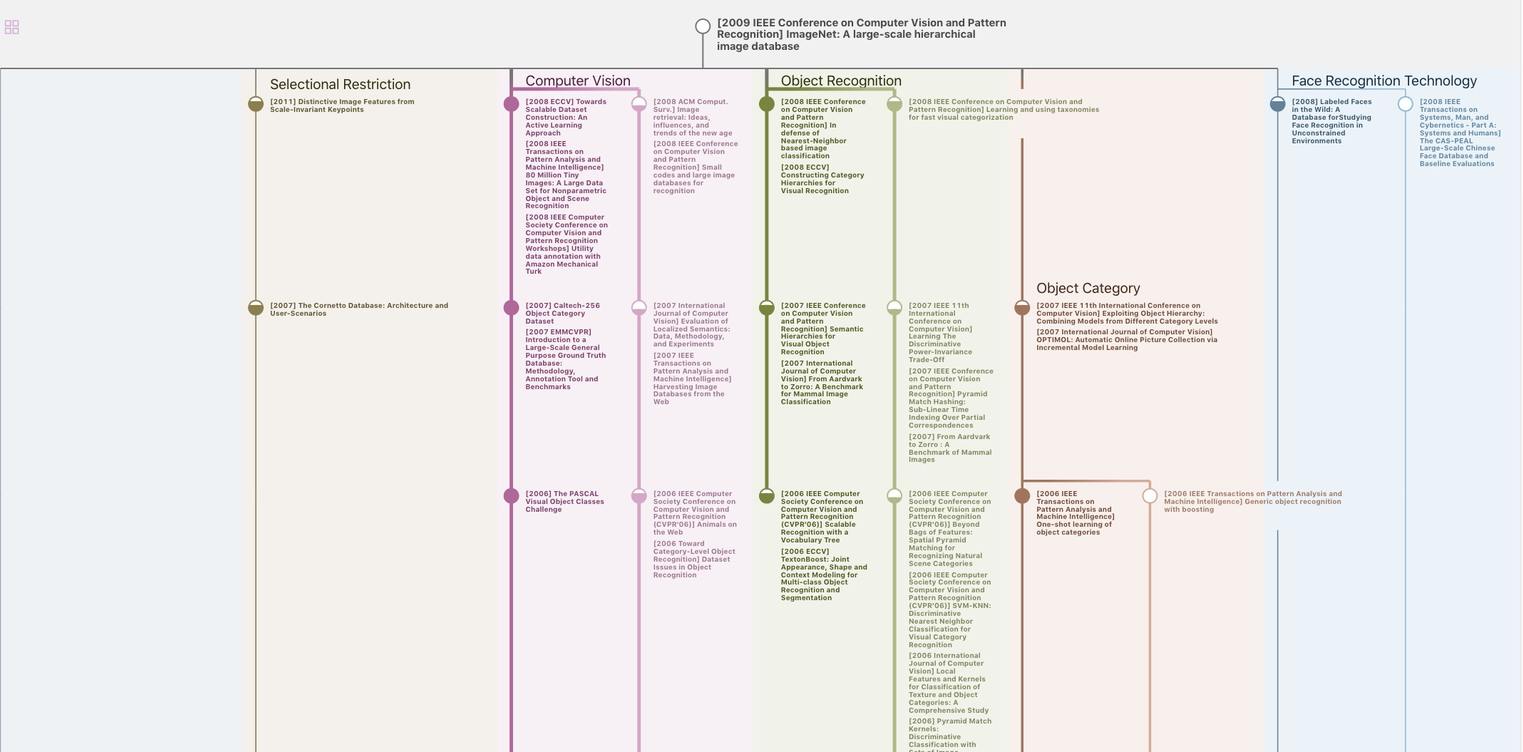
生成溯源树,研究论文发展脉络
Chat Paper
正在生成论文摘要