Perturbation Theory-Aided Learned Digital Back-Propagation Scheme for Optical Fiber Nonlinearity Compensation
Journal of Lightwave Technology(2022)
摘要
Derived from the regular perturbation treatment of the nonlinear Schrödinger equation, a machine learning-based scheme to mitigate the intra-channel optical fiber nonlinearity is proposed. Referred to as the perturbation theory-aided (PA) learned digital back propagation (LDBP), the proposed scheme constructs a deep neural network (DNN) in a way similar to the split-step Fourier method: linear and nonlinear operations alternate. Inspired by the perturbation analysis, the intra-channel cross phase modulation term is conveniently represented by matrix operations in the DNN. The introduction of this term in each nonlinear operation considerably improves the performance, as well as enables the flexibility of PA-LDBP by adjusting the numbers of spans per step. The proposed scheme is evaluated by numerical simulations of a single-carrier optical fiber communication system operating at 32 Gbaud with 64-quadrature amplitude modulation and 20 × 80 km transmission distance. The results show that the proposed scheme achieves approximately 1 dB, 1.2 dB, 1.2 dB, and 0.5 dB performance gain in terms of
${\it Q}$
factor over LDBP, when the numbers of spans per step are 1, 2, 4, and 10, respectively. Two methods are proposed to reduce the complexity of PA-LDBP, i.e., pruning the number of perturbation coefficients and chromatic dispersion compensation in the frequency domain for multi-span per step cases. Investigation of the performance and complexity suggests that PA-LDBP attains improved performance gains with reduced complexity when compared to LDBP in the cases of 4 and 10 spans per step.
更多查看译文
关键词
Digital signal processing,fiber nonlinearity compensation,machine learning,optical fiber communication,perturbation theory
AI 理解论文
溯源树
样例
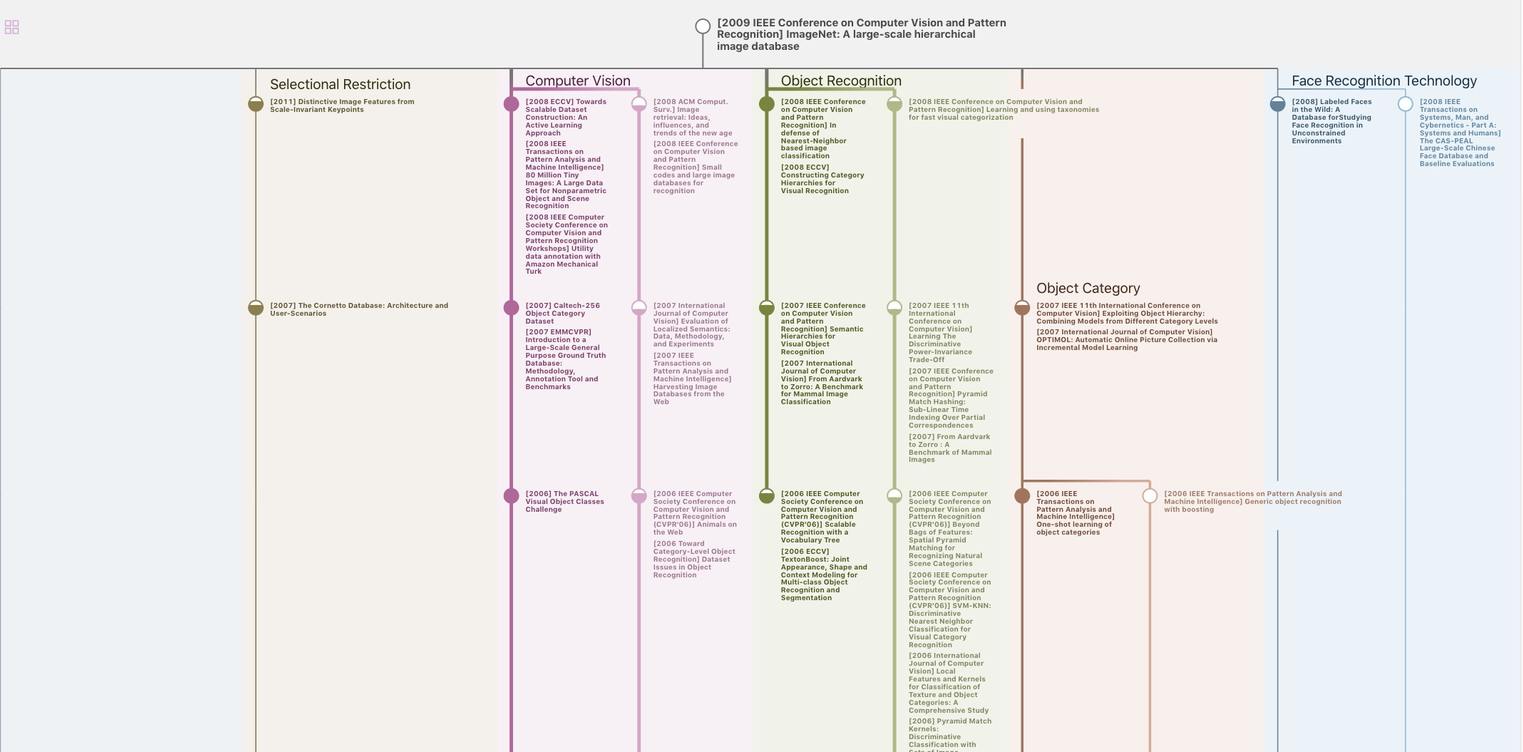
生成溯源树,研究论文发展脉络
Chat Paper
正在生成论文摘要