Learning from Ambiguous Demonstrations with Self-Explanation Guided Reinforcement Learning
AAAI 2024(2024)
摘要
Our work aims at efficiently leveraging ambiguous demonstrations for the training of a reinforcement learning (RL) agent. An ambiguous demonstration can usually be interpreted in multiple ways, which severely hinders the RL agent from learning stably and efficiently. Since an optimal demonstration may also suffer from being ambiguous, previous works that combine RL and learning from demonstration (RLfD works) may not work well. Inspired by how humans handle such situations, we propose to use self-explanation (an agent generates explanations for itself) to recognize valuable high-level relational features as an interpretation of why a successful trajectory is successful. This way, the agent can leverage the explained important relations as guidance for its RL learning. Our main contribution is to propose the Self-Explanation for RL from Demonstrations (SERLfD) framework, which can overcome the limitations of existing RLfD works. Our experimental results show that an RLfD model can be improved by using our SERLfD framework in terms of training stability and performance. To foster further research in self-explanation-guided robot learning, we have made our demonstrations and code publicly accessible at https://github.com/YantianZha/SERLfD. For a deeper understanding of our work, interested readers can refer to our arXiv version at https://arxiv.org/pdf/2110.05286.pdf, including an accompanying appendix.
更多查看译文
关键词
ROB: Cognitive Robotics
AI 理解论文
溯源树
样例
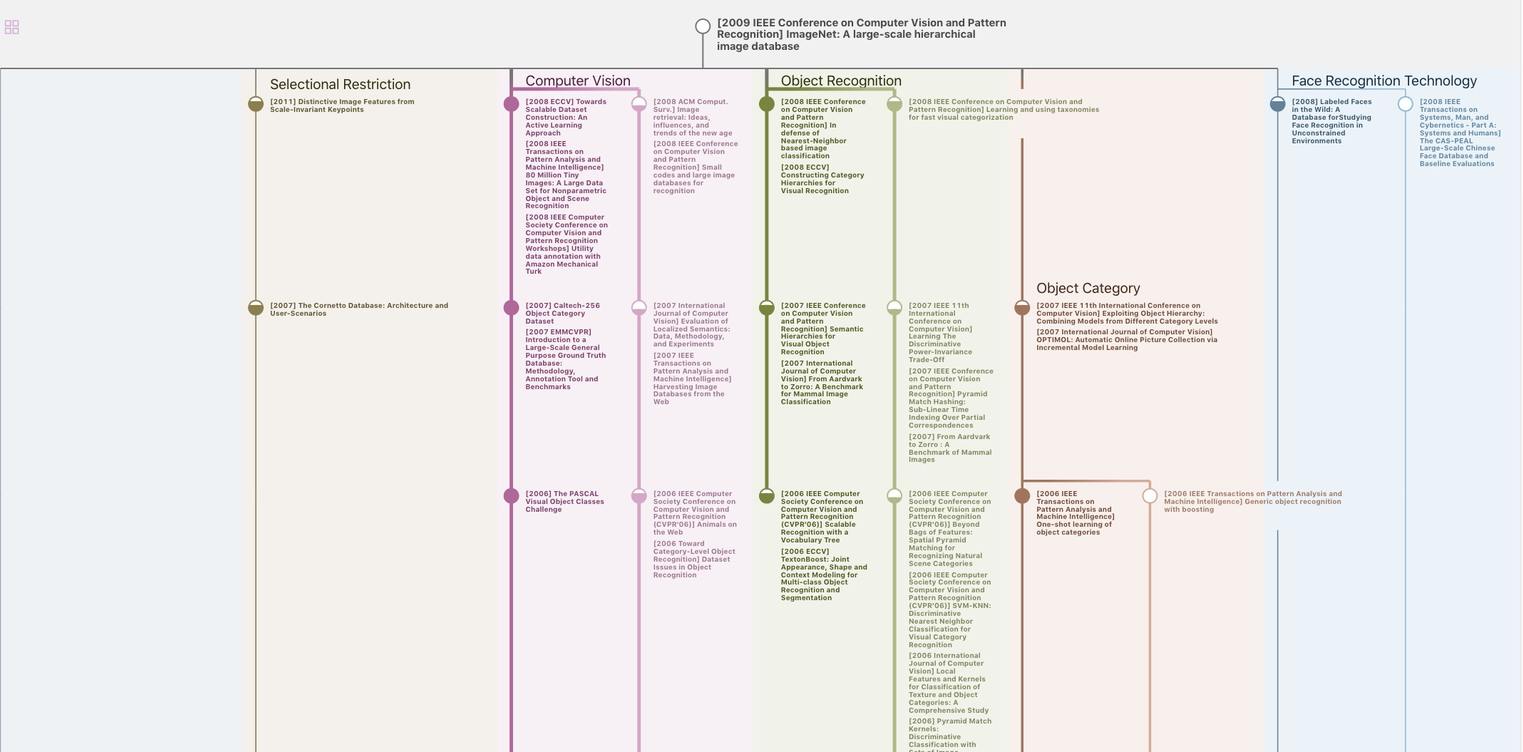
生成溯源树,研究论文发展脉络
Chat Paper
正在生成论文摘要