Multi-Task Learning for Situated Multi-Domain End-to-End Dialogue Systems
arxiv(2021)
摘要
Task-oriented dialogue systems have been a promising area in the NLP field. Previous work showed the effectiveness of using a single GPT-2 based model to predict belief states and responses via causal language modeling. In this paper, we leverage multi-task learning techniques to train a GPT-2 based model on a more challenging dataset with multiple domains, multiple modalities, and more diversity in output formats. Using only a single model, our method achieves better performance on all sub-tasks, across domains, compared to task and domain-specific models. Furthermore, we evaluated several proposed strategies for GPT-2 based dialogue systems with comprehensive ablation studies, showing that all techniques can further improve the performance.
更多查看译文
关键词
dialogue,learning,multi-task,multi-domain,end-to-end
AI 理解论文
溯源树
样例
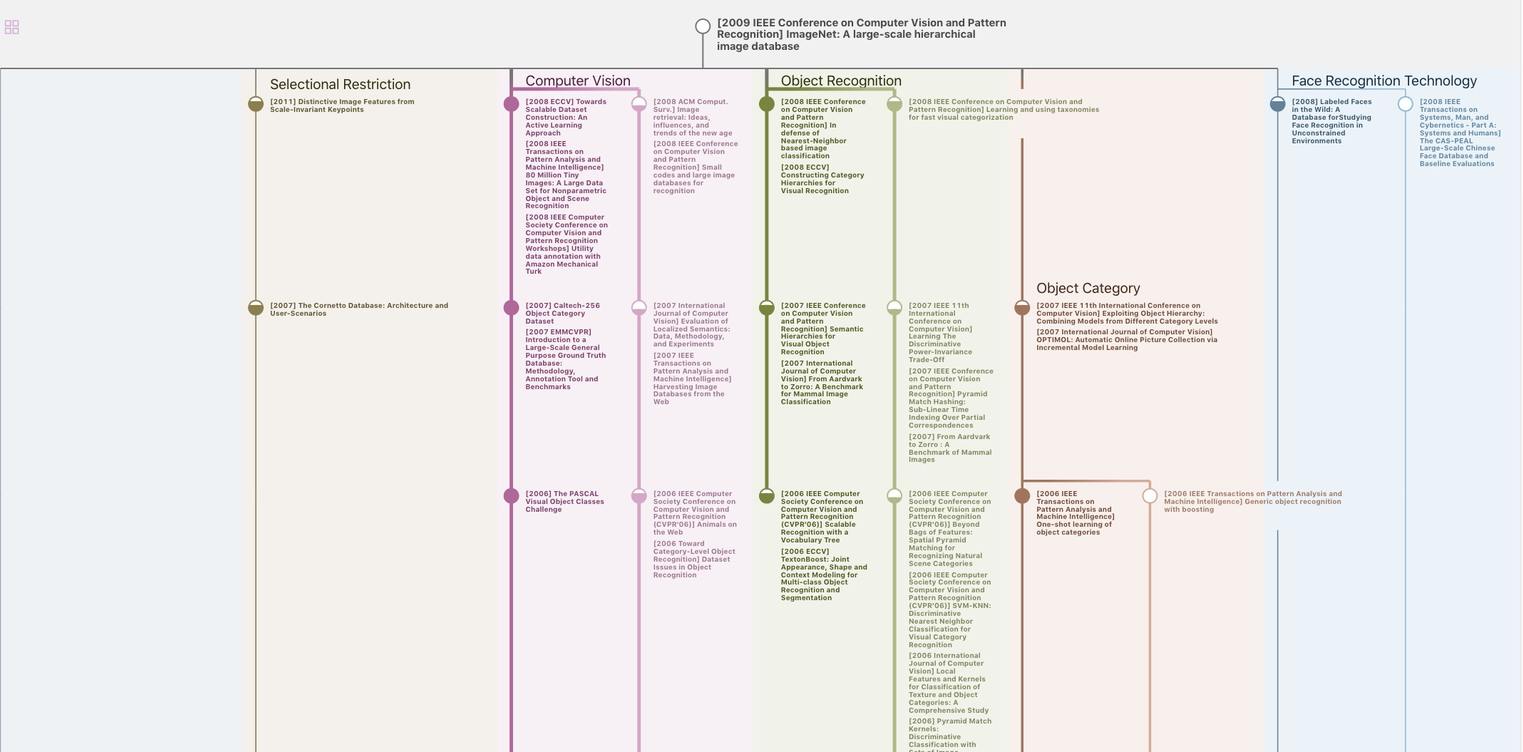
生成溯源树,研究论文发展脉络
Chat Paper
正在生成论文摘要