Rethinking Person Re-Identification via Semantic-based Pretraining
ACM TRANSACTIONS ON MULTIMEDIA COMPUTING COMMUNICATIONS AND APPLICATIONS(2024)
摘要
Pretraining is a dominant paradigm in computer vision. Generally, supervised ImageNet pretraining is commonly used to initialize the backbones of person re-identification (Re-ID) models. However, recent works show a surprising result that CNN-based pretraining on ImageNet has limited impacts on Re-ID system due to the large domain gap between ImageNet and person Re-ID data. To seek an alternative to traditional pretraining, here we investigate semantic-based pretraining as another method to utilize additional textual data against ImageNet pretraining. Specifically, we manually construct a diversified FineGPR-C caption dataset for the first time on person Re-ID events. Based on it, a pure semantic-based pretraining approach named VTBR is proposed to adopt dense captions to learn visual representations with fewer images. We train convolutional neural networks from scratch on the captions of FineGPR-C dataset, and then transfer them to downstream Re-ID tasks. Comprehensive experiments conducted on benchmark datasets show that our VTBR can achieve competitive performance compared with ImageNet pretraining-despite using up to 1.4x fewer images, revealing its potential in Re-ID pretraining. Our source code is also publicly available at https://github.com/JeremyXSC/VTBR.
更多查看译文
关键词
Person re-identification,synthetic data,efficient training
AI 理解论文
溯源树
样例
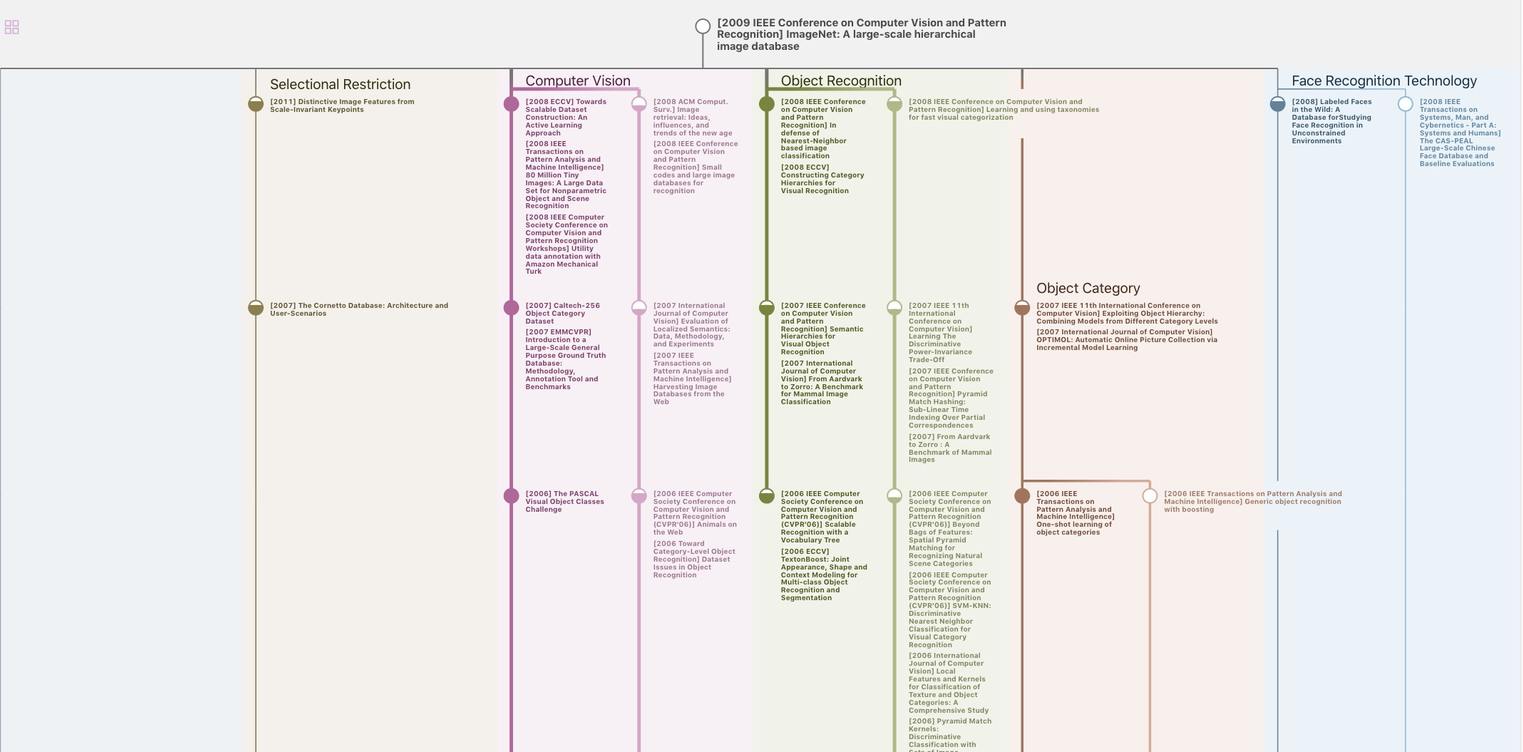
生成溯源树,研究论文发展脉络
Chat Paper
正在生成论文摘要