Adaptive joint distribution learning
arxiv(2021)
摘要
We develop a new framework for embedding joint probability distributions in
tensor product reproducing kernel Hilbert spaces (RKHS). Our framework
accommodates a low-dimensional, normalized and positive model of a
Radon-Nikodym derivative, which we estimate from sample sizes of up to several
million data points, alleviating the inherent limitations of RKHS modeling.
Well-defined normalized and positive conditional distributions are natural
by-products to our approach. The embedding is fast to compute and accommodates
learning problems ranging from prediction to classification. Our theoretical
findings are supplemented by favorable numerical results.
更多查看译文
关键词
adaptive joint distribution learning
AI 理解论文
溯源树
样例
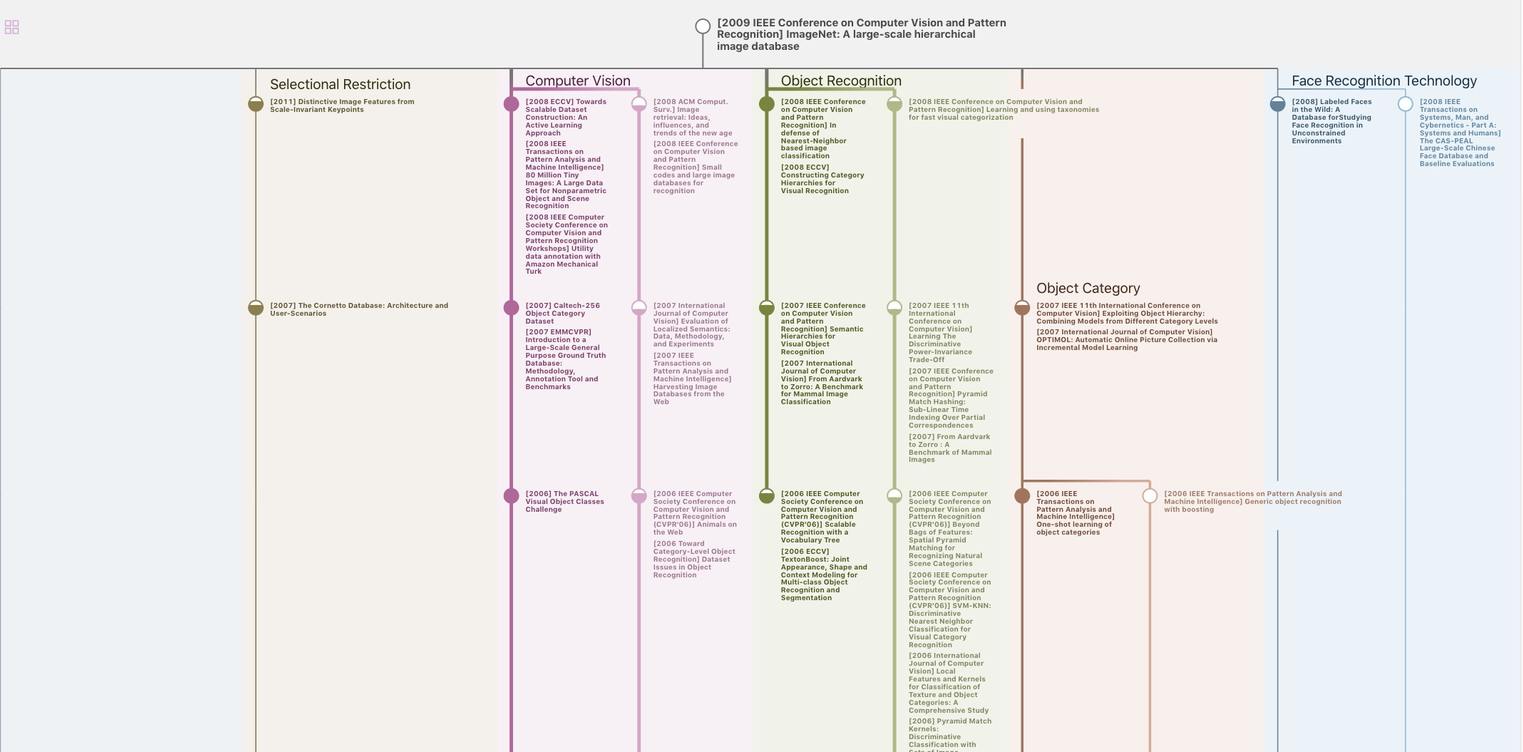
生成溯源树,研究论文发展脉络
Chat Paper
正在生成论文摘要