Bayesian active summarization
COMPUTER SPEECH AND LANGUAGE(2024)
摘要
Bayesian Active Learning has had significant impact to various NLP problems, but nevertheless its application to text summarization has been explored very little. We introduce Bayesian Active Summarization (BAS), as a method of combining active learning methods with stateof-the-art summarization models. Our findings suggest that BAS achieves better and more robust performance, compared to random selection, particularly for small and very small data annotation budgets. More specifically, applying BAS with a summarization model like PEGASUS we managed to reach 95% of the performance of the fully trained model, using less than 150 training samples. Furthermore, we have reduced standard deviation by 18% compared to the conventional random selection strategy. Using BAS we showcase it is possible to leverage large summarization models to effectively solve real-world problems with very limited annotated data.
更多查看译文
关键词
Active learning,Abstractive text summarization,Bayes methods,Monte Carlo methods,Natural language processing,Deep learning
AI 理解论文
溯源树
样例
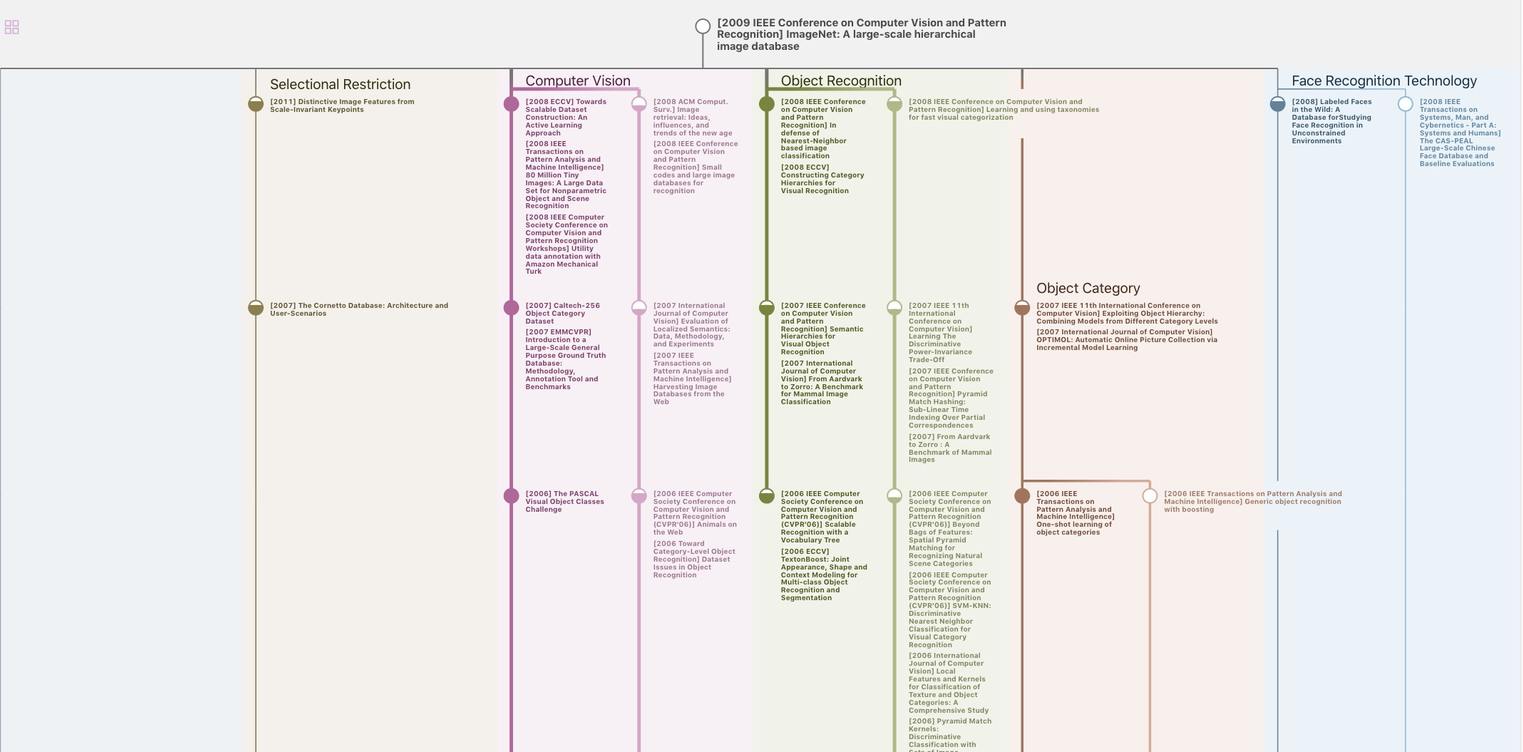
生成溯源树,研究论文发展脉络
Chat Paper
正在生成论文摘要