Aura: Privacy-preserving Augmentation to Improve Test Set Diversity in Speech Enhancement
arxiv(2023)
摘要
Noise suppression models running in production environments are commonly trained on publicly available datasets. However, this approach leads to regressions due to the lack of training/testing on representative customer data. Moreover, due to privacy reasons, developers cannot listen to customer content. This `ears-off' situation motivates augmenting existing datasets in a privacy-preserving manner. In this paper, we present Aura, a solution to make existing noise suppression test sets more challenging and diverse while being sample efficient. Aura is `ears-off' because it relies on a feature extractor and a metric of speech quality, DNSMOS P.835, both pre-trained on data obtained from public sources. As an application of Aura, we augment the INTERSPEECH 2021 DNS challenge by sampling audio files from a new batch of data of 20K clean speech clips from Librivox mixed with noise clips obtained from AudioSet. Aura makes the existing benchmark test set harder by 0.27 in DNSMOS P.835 OVLR (7%), 0.64 harder in DNSMOS P.835 SIG (16%), increases diversity by 31%, and achieves a 26% improvement in Spearman's rank correlation coefficient (SRCC) compared to random sampling. Finally, we open-source Aura to stimulate research of test set development.
更多查看译文
关键词
speech enhancement,test set diversity,aura,privacy-preserving
AI 理解论文
溯源树
样例
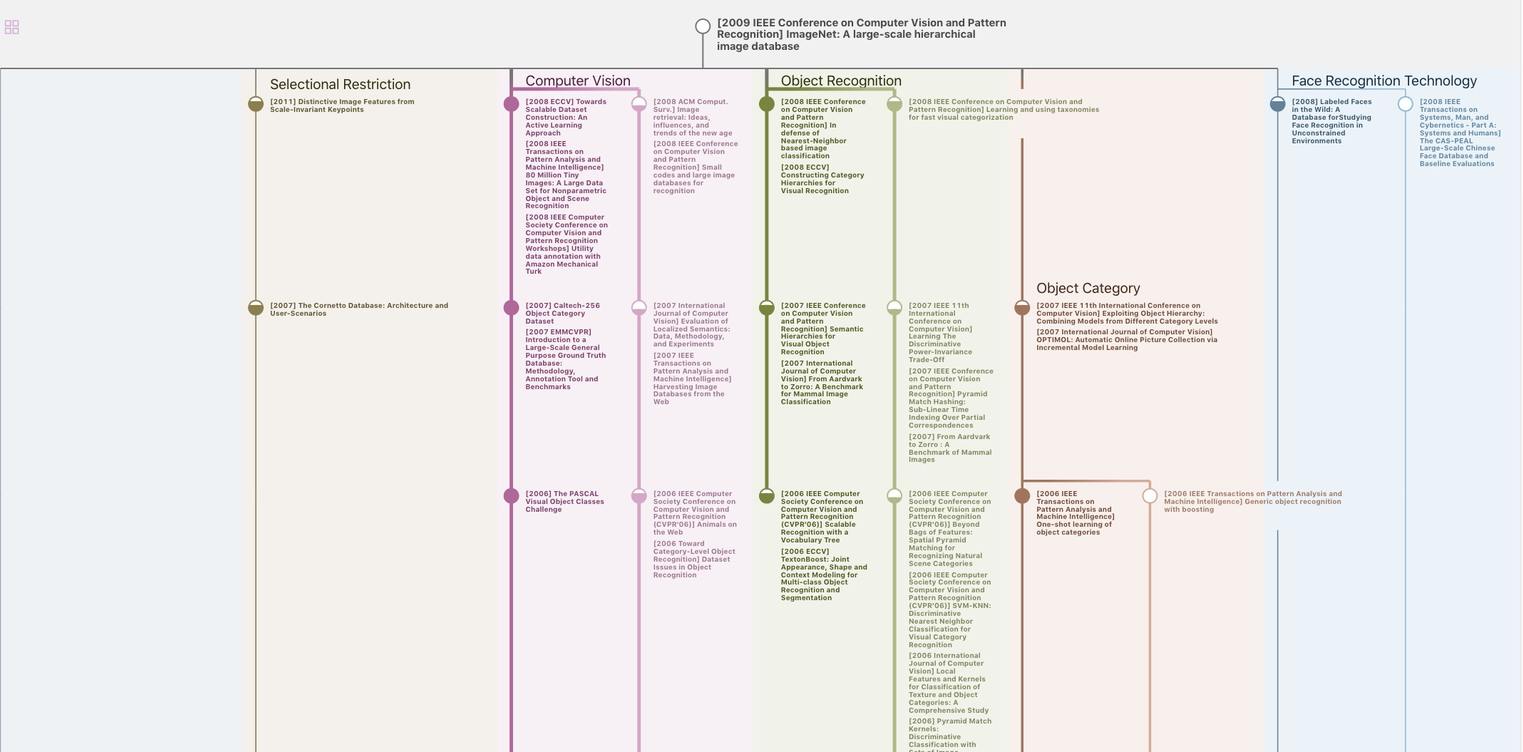
生成溯源树,研究论文发展脉络
Chat Paper
正在生成论文摘要