Performance optimizations on deep noise suppression models
arxiv(2021)
摘要
We study the role of magnitude structured pruning as an architecture search to speed up the inference time of a deep noise suppression (DNS) model. While deep learning approaches have been remarkably successful in enhancing audio quality, their increased complexity inhibits their deployment in real-time applications. We achieve up to a 7.25X inference speedup over the baseline, with a smooth model performance degradation. Ablation studies indicate that our proposed network re-parameterization (i.e., size per layer) is the major driver of the speedup, and that magnitude structured pruning does comparably to directly training a model in the smaller size. We report inference speed because a parameter reduction does not necessitate speedup, and we measure model quality using an accurate non-intrusive objective speech quality metric.
更多查看译文
关键词
deep noise suppression models,performance
AI 理解论文
溯源树
样例
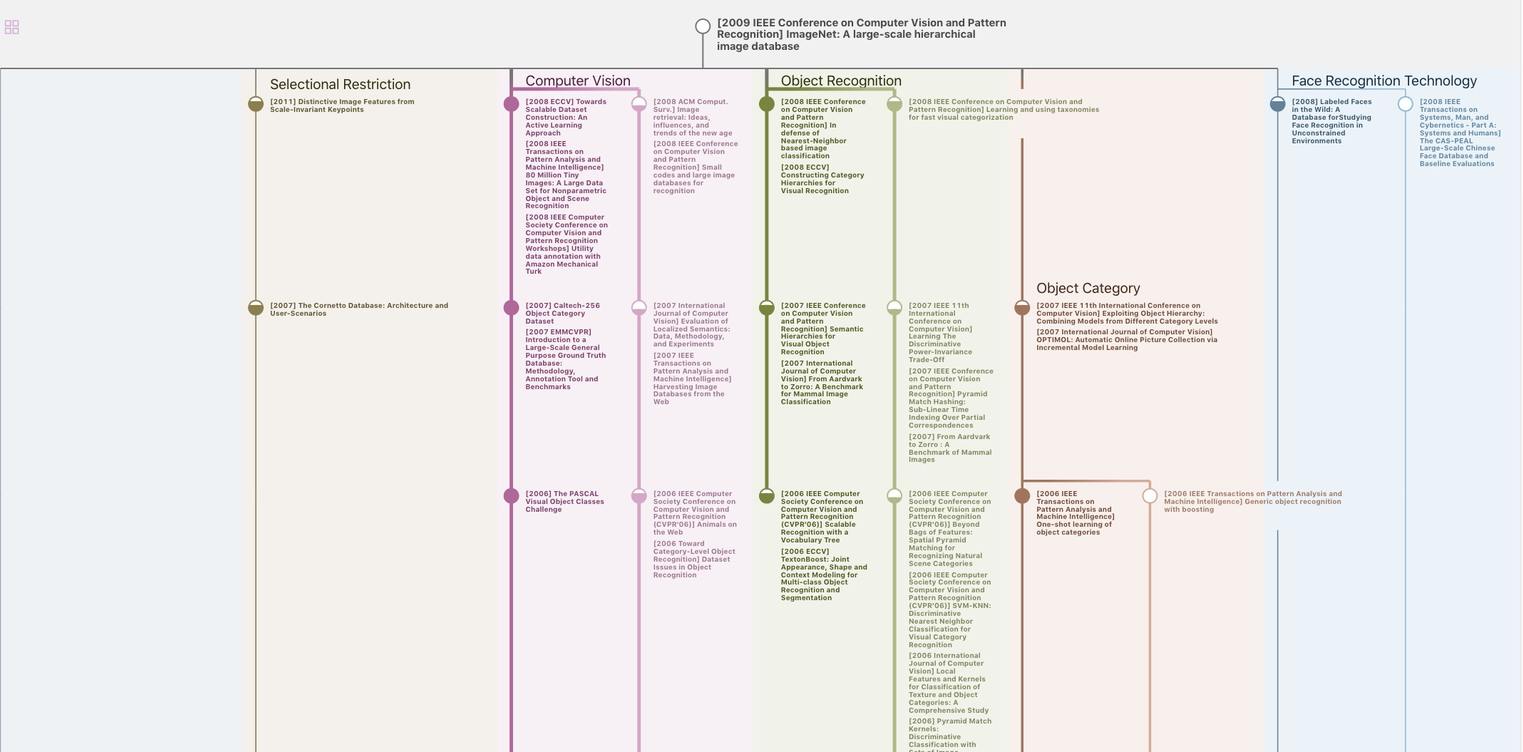
生成溯源树,研究论文发展脉络
Chat Paper
正在生成论文摘要