The Extreme Value Evolving Predictor in Multiple Time Series Learning.
ICAISC(2021)
摘要
This paper extends the evolving fuzzy-rule-based algorithm denoted Extreme Value evolving Predictor (EVeP) to deal with multivariate time series. EVeP offers a statistically well-founded approach to the online definition of the fuzzy granules at the antecedent and consequent parts of evolving fuzzy rules. The interplay established by these granules is used to formulate a regularized multitask learning problem which employs a sparse graph of the structural relationship promoted by the rules. With this multitask strategy, the Takagi-Sugeno consequent terms of the rules are then properly determined. In this extended version, called Extreme Value evolving Predictor in Multiple Time Series Learning (EVeP MTSL), we propose an approach that resorts to the similarity degree among the time series. The similarity is calculated by the distance correlation statistical measure extracted from a sliding window of data points belonging to the multiple time series. Noticing that each fuzzy rule is part of a specific time series predictor, the new unified model called EVeP MTSL updates the sparse graph by composing the relationship established by each pair of fuzzy rules (already provided by EVeP) with the similarity degree of their corresponding time series. We are then exploring not only the current interplay of the multiple rules that compose each evolving predictor, but also the current correlation of the multiple time series being simultaneously predicted. Two computational experiments reveal the superior performance of EVeP MTSL when compared with other contenders devoted to online multivariate time series prediction.
更多查看译文
关键词
Evolving fuzzy-rule-based systems,Online learning,Extreme Value Theory,Multitask learning,Multivariate time series prediction
AI 理解论文
溯源树
样例
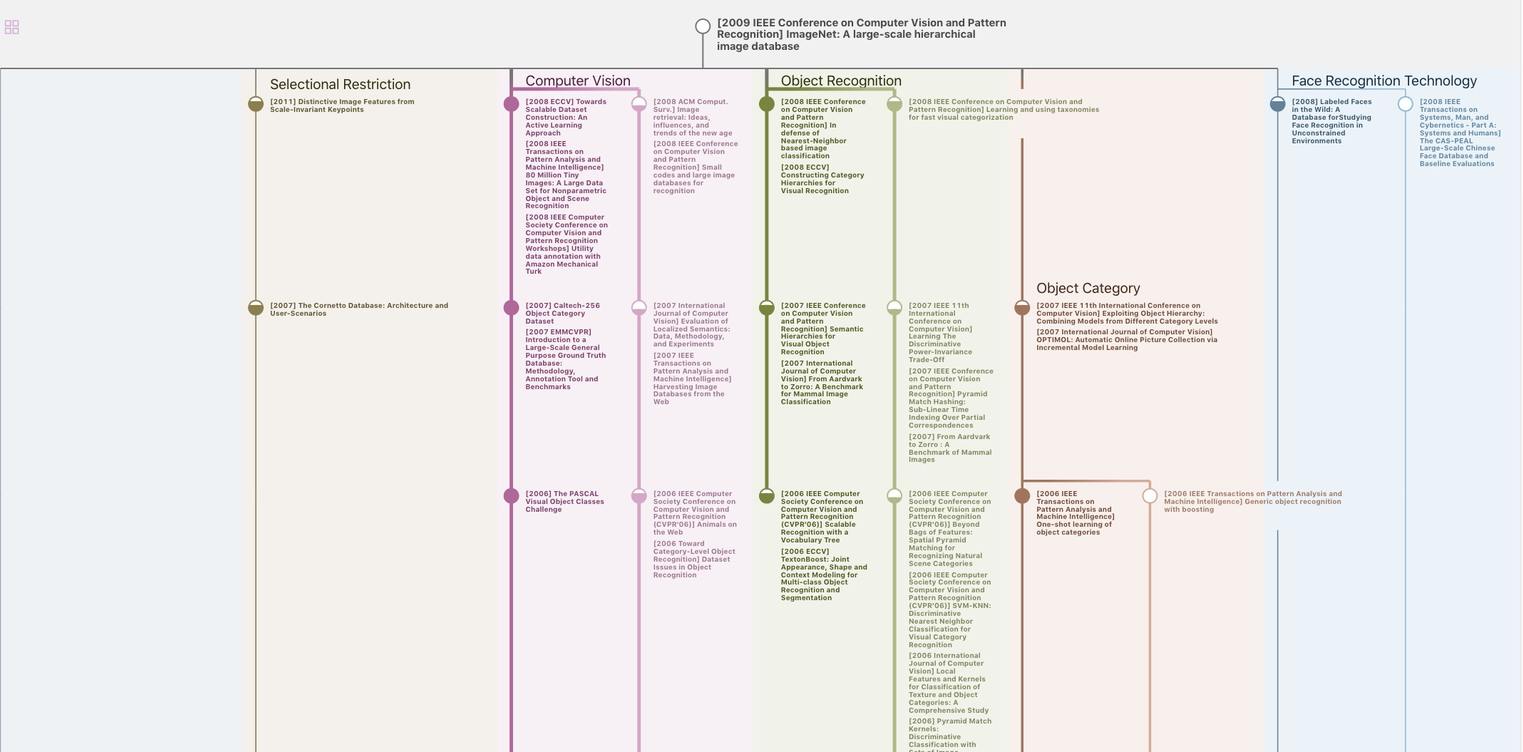
生成溯源树,研究论文发展脉络
Chat Paper
正在生成论文摘要