Intensity-Aware Single-Image Deraining With Semantic And Color Regularization
IEEE TRANSACTIONS ON IMAGE PROCESSING(2021)
摘要
Rain degrades image visual quality and disrupts object structures, obscuring their details and erasing their colors. Existing deraining methods are primarily based on modeling either visual appearances of rain or its physical characteristics (e.g., rain direction and density), and thus suffer from two common problems. First, due to the stochastic nature of rain, they tend to fail in recognizing rain streaks correctly, and wrongly remove image structures and details. Second, they fail to recover the image colors erased by heavy rain. In this paper, we address these two problems with the following three contributions. First, we propose a novel PHP block to aggregate comprehensive spatial and hierarchical information for removing rain streaks of different sizes. Second, we propose a novel network to first remove rain streaks, then recover objects structures/colors, and finally enhance details. Third, to train the network, we prepare a new dataset, and propose a novel loss function to introduce semantic and color regularization for deraining. Extensive experiments demonstrate the superiority of the proposed method over state-of-the-art deraining methods on both synthesized and real-world data, in terms of visual quality, quantitative accuracy, and running speed.
更多查看译文
关键词
Rain, Image color analysis, Semantics, Task analysis, Visualization, Cameras, Urban areas, Rain removal, image reconstruction, neural networks
AI 理解论文
溯源树
样例
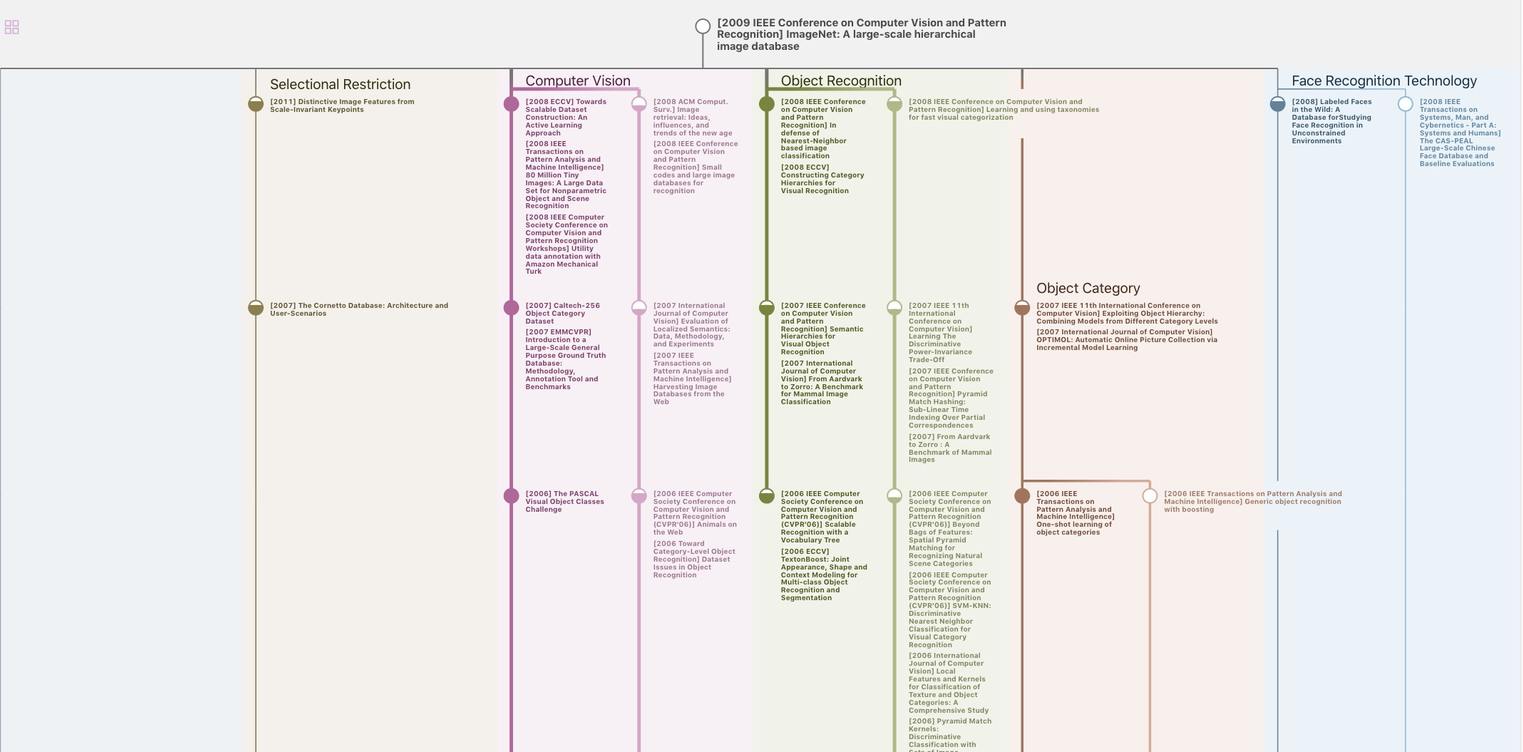
生成溯源树,研究论文发展脉络
Chat Paper
正在生成论文摘要