Bias-Variance Tradeoffs in Single-Sample Binary Gradient Estimators.
GCPR(2021)
摘要
Discrete and especially binary random variables occur in many machine learning models, notably in variational autoencoders with binary latent states and in stochastic binary networks. When learning such models, a key tool is an estimator of the gradient of the expected loss with respect to the probabilities of binary variables. The straight-through (ST) estimator gained popularity due to its simplicity and efficiency, in particular in deep networks where unbiased estimators are impractical. Several techniques were proposed to improve over ST while keeping the same low computational complexity: Gumbel-Softmax, ST-Gumbel-Softmax, BayesBiNN, FouST. We conduct a theoretical analysis of Bias and Variance of these methods in order to understand tradeoffs and verify the originally claimed properties. The presented theoretical results are mainly negative, showing limitations of these methods and in some cases revealing serious issues.
更多查看译文
关键词
bias-variance,single-sample
AI 理解论文
溯源树
样例
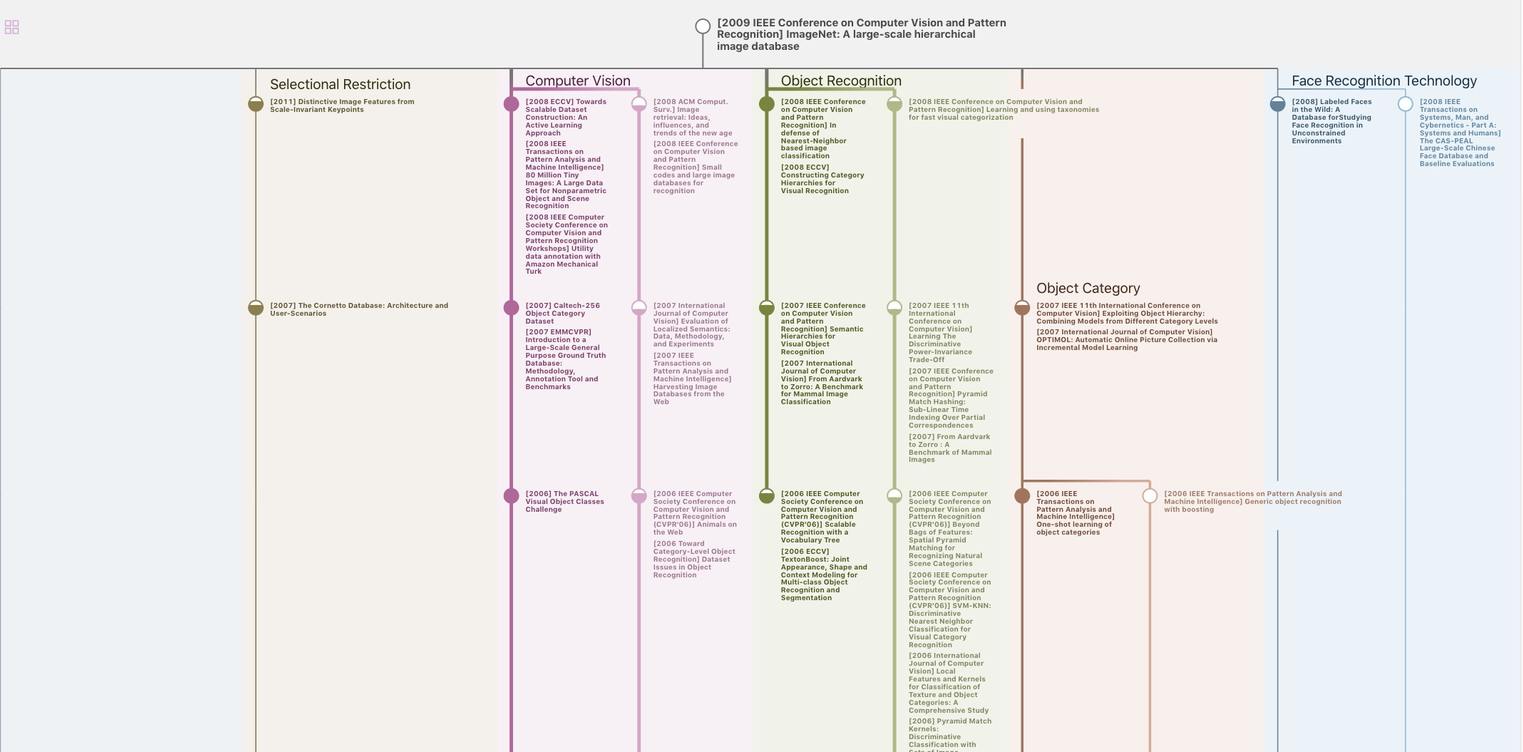
生成溯源树,研究论文发展脉络
Chat Paper
正在生成论文摘要