A comparison of neural network architectures for data-driven reduced-order modeling
Computer Methods in Applied Mechanics and Engineering(2022)
摘要
The popularity of deep convolutional autoencoders (CAEs) has engendered new and effective reduced-order models (ROMs) for the simulation of large-scale dynamical systems. Despite this, it is still unknown whether deep CAEs provide superior performance over established linear techniques or other network-based methods in all modeling scenarios. To elucidate this, the effect of autoencoder architecture on its associated ROM is studied through the comparison of deep CAEs against two alternatives: a simple fully connected autoencoder, and a novel graph convolutional autoencoder. Through benchmark experiments, it is shown that the superior autoencoder architecture for a given ROM application is highly dependent on the size of the latent space and the structure of the snapshot data, with the proposed architecture demonstrating benefits on data with irregular connectivity when the latent space is sufficiently large.
更多查看译文
关键词
65M22,65M60
AI 理解论文
溯源树
样例
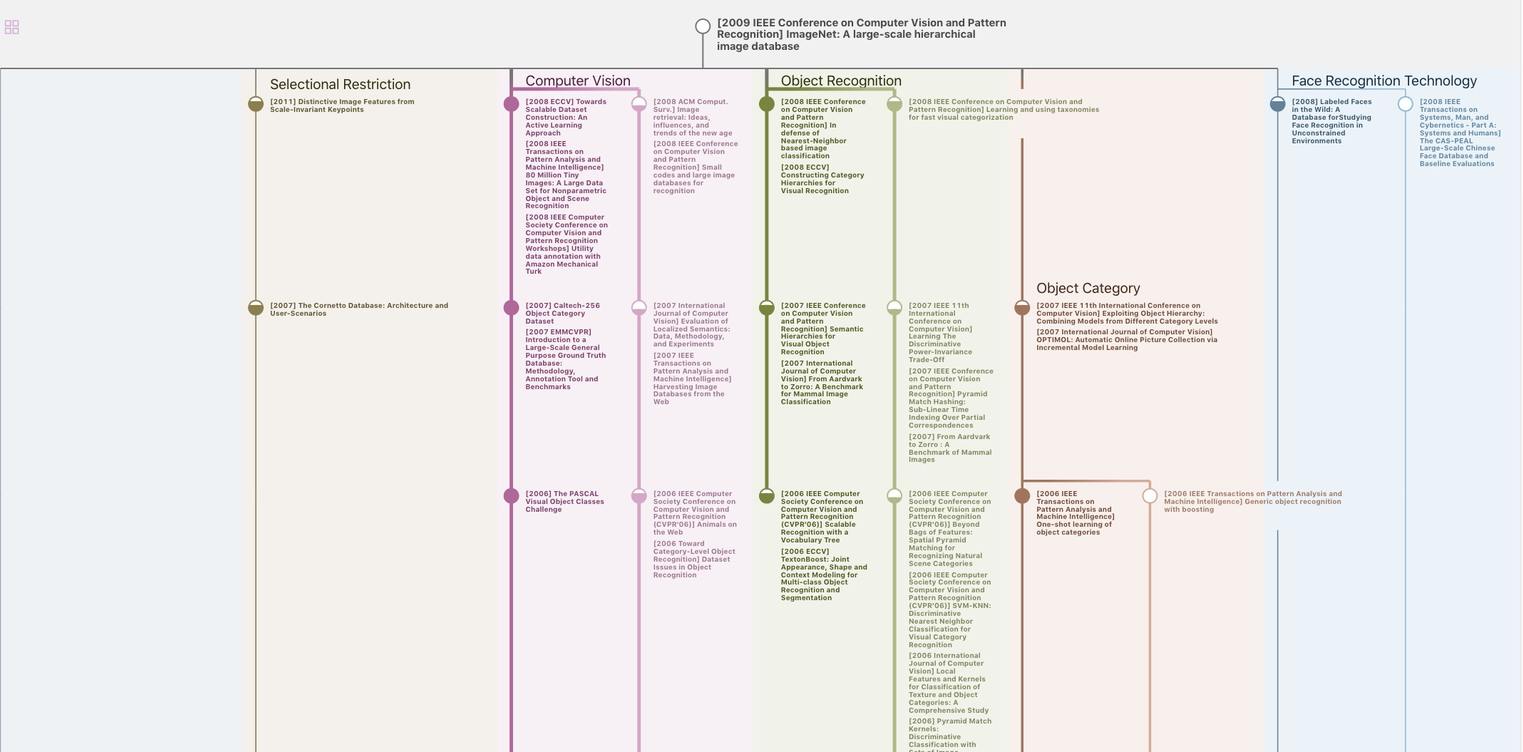
生成溯源树,研究论文发展脉络
Chat Paper
正在生成论文摘要