Intelligent Target Recognition For Distributed Acoustic Sensors By Using Both Manual And Deep Features
APPLIED OPTICS(2021)
摘要
Effective information mining of fiber-optic distributed acoustic sensors (DAS) is so important that it attracts more and more public attention, and various manual and deep feature extraction methods have been developed. However, either way it has limits; for example, the manual features contain insufficient information, and the deep features could be unreliable because of the overfitting problem. Thus, in this paper, to avoid the disadvantages of each and make full use of the effective information carried by DAS signals, an intelligent target recognition method by utilizing both manual and deep features is proposed. The manual features are first extracted in the time domain, frequency domain, semantic domain, and from dynamic models, which are fused with the deep features extracted by a four-layer 1D convolutional neural network (CNN) through feature engineering. The features are ranked and then selected by a combined weighting method of analysis of variance and maximum information coefficient. Then finally, an optimal classifier is selected by comparing support vector machine, extreme gradient boost, random forest, and native Bayesian. In the test with real field data, four types of features, which include the manual features, the CNN features, and the combined features without and with selection, are compared with these different classifiers. As a result, it shows the combined features without selection can improve the identification ability of DAS compared with the recognition with only manual or deep features. The combined features with selection can further improve the computation efficiency and save up to 90% of time with a performance degradation of less than 1%. (C) 2021 Optical Society of America
更多查看译文
AI 理解论文
溯源树
样例
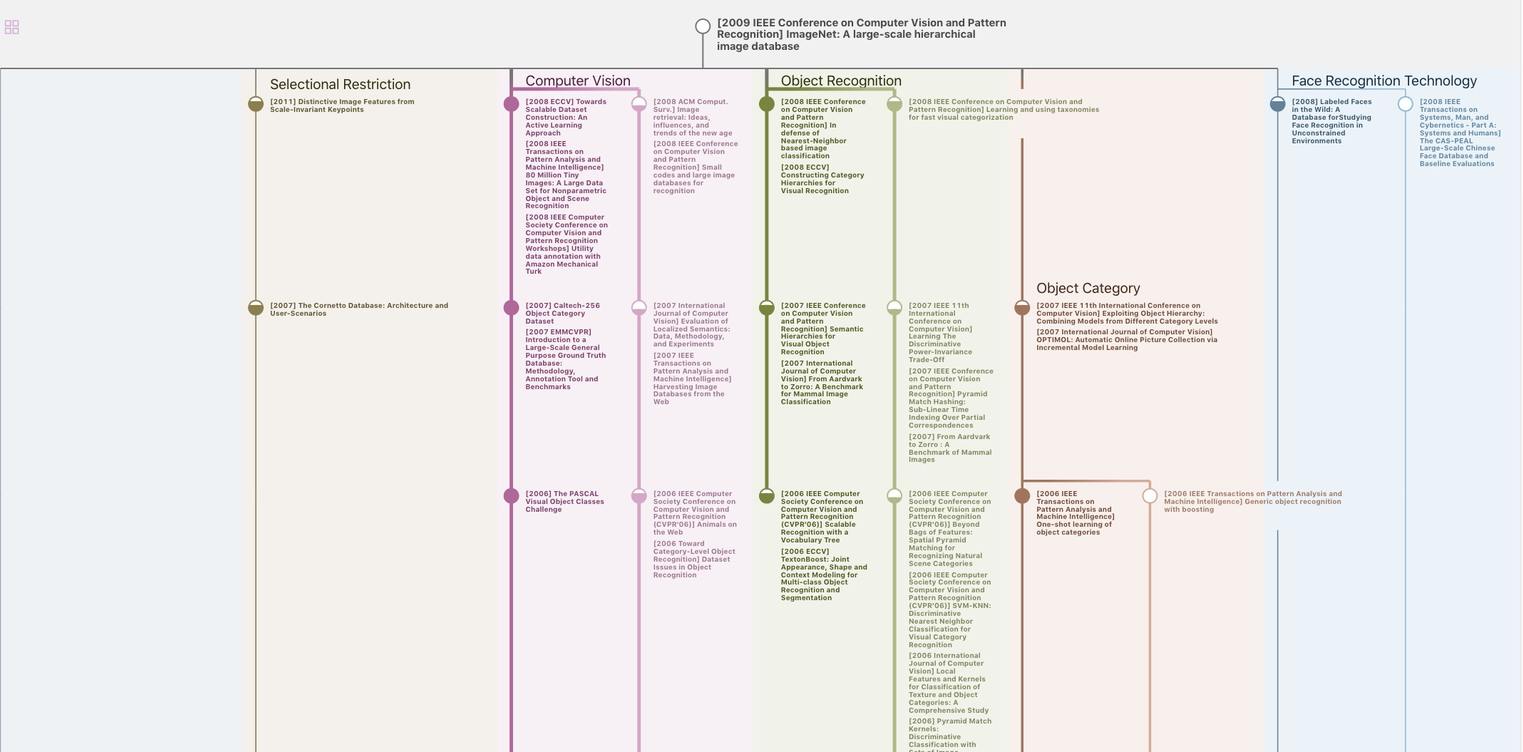
生成溯源树,研究论文发展脉络
Chat Paper
正在生成论文摘要