A Regularized Wasserstein Framework for Graph Kernels
2021 IEEE International Conference on Data Mining (ICDM)(2021)
摘要
We propose a learning framework for graph kernels, which is theoretically grounded on regularizing optimal transport. This framework provides a novel optimal transport distance metric, namely Regularized Wasserstein (RW) discrepancy, which can preserve both features and structure of graphs via Wasserstein distances on features and their local variations, local barycenters and global connectivity. ...
更多查看译文
关键词
Measurement,Databases,Conferences,Benchmark testing,Data models,Data mining,Kernel
AI 理解论文
溯源树
样例
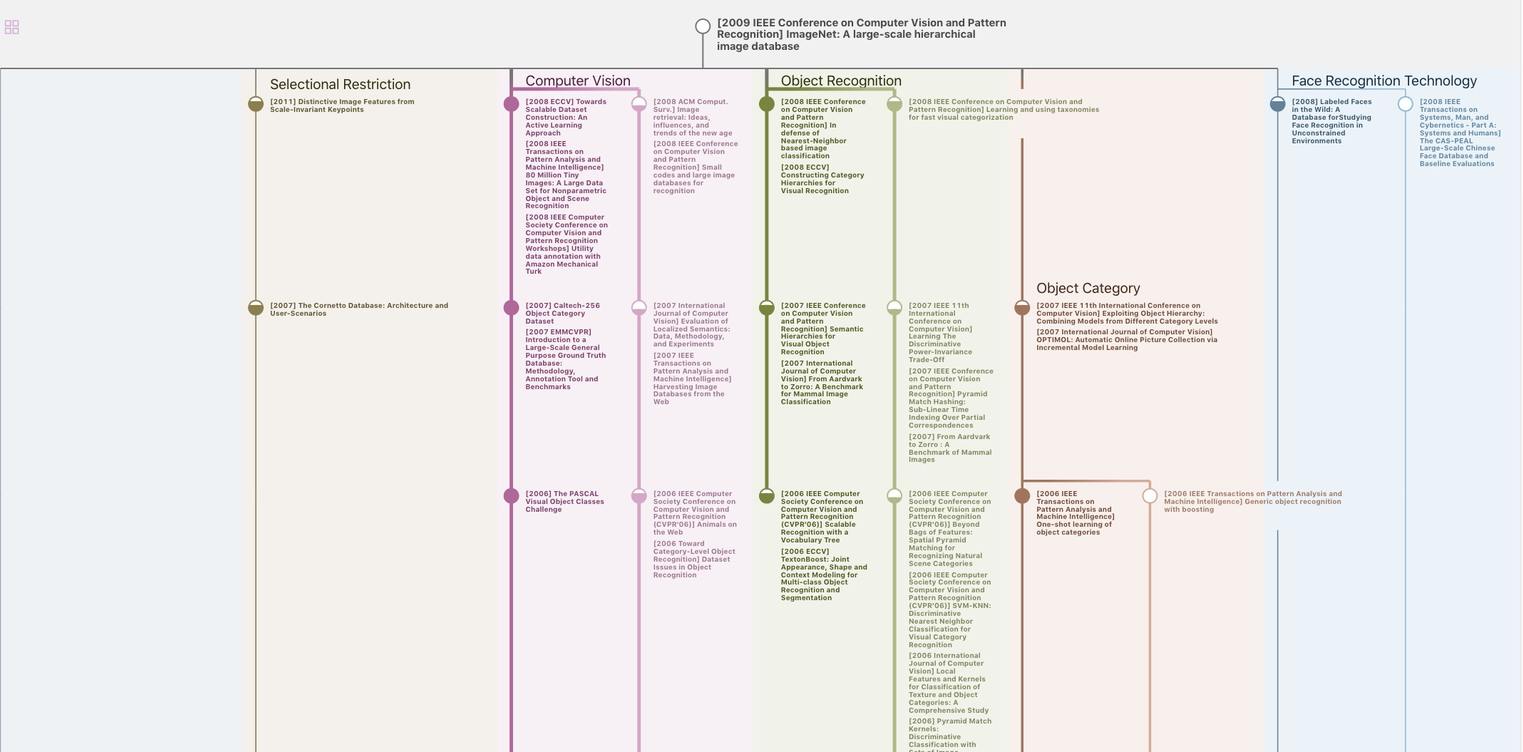
生成溯源树,研究论文发展脉络
Chat Paper
正在生成论文摘要