Spectral Bias in Practice: The Role of Function Frequency in Generalization
NeurIPS 2022(2022)
摘要
Despite their ability to represent highly expressive functions, deep learning models trained with SGD seem to find simple, constrained solutions that generalize surprisingly well. Spectral bias - the tendency of neural networks to prioritize learning low frequency functions - is one possible explanation for this phenomenon, but so far spectral bias has only been observed in theoretical models and simplified experiments. In this work, we propose methodologies for measuring spectral bias in modern image classification networks. We find that these networks indeed exhibit spectral bias, and that networks that generalize well strike a balance between having enough complexity(i.e. high frequencies) to fit the data while being simple enough to avoid overfitting. For example, we experimentally show that larger models learn high frequencies faster than smaller ones, but many forms of regularization, both explicit and implicit, amplify spectral bias and delay the learning of high frequencies. We also explore the connections between function frequency and image frequency and find that spectral bias is sensitive to the low frequencies prevalent in natural images. Our work enables measuring and ultimately controlling the spectral behavior of neural networks used for image classification, and is a step towards understanding why deep models generalize well
更多查看译文
关键词
spectral bias,generalization,data augmentation,self-distillation,image classification
AI 理解论文
溯源树
样例
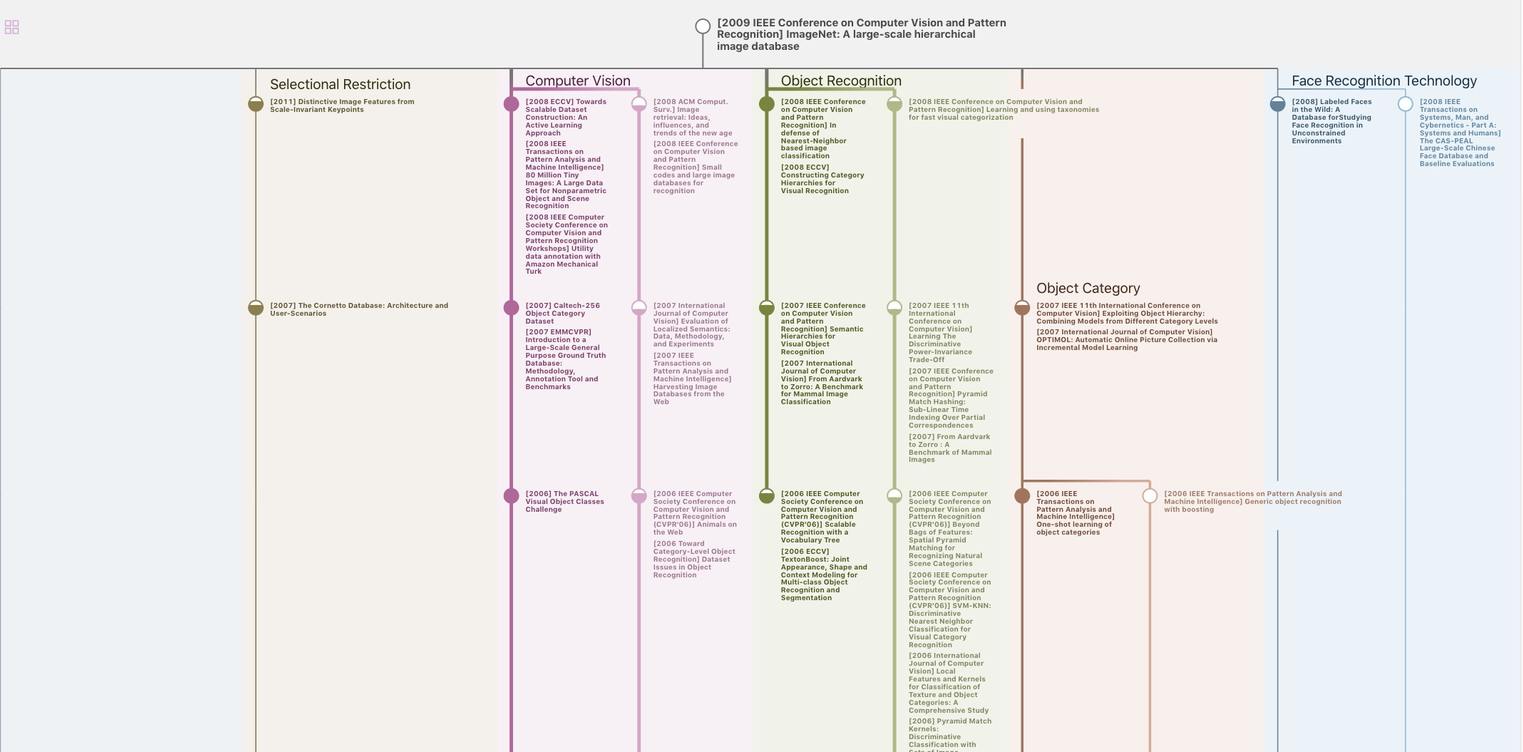
生成溯源树,研究论文发展脉络
Chat Paper
正在生成论文摘要