Evolutionary Algorithms for Solving Unconstrained, Constrained and Multi-objective Noisy Combinatorial Optimisation Problems
arxiv(2021)
摘要
We present an empirical study of a range of evolutionary algorithms applied to various noisy combinatorial optimisation problems. There are three sets of experiments. The first looks at several toy problems, such as OneMax and other linear problems. We find that UMDA and the Paired-Crossover Evolutionary Algorithm (PCEA) are the only ones able to cope robustly with noise, within a reasonable fixed time budget. In the second stage, UMDA and PCEA are then tested on more complex noisy problems: SubsetSum, Knapsack and SetCover. Both perform well under increasing levels of noise, with UMDA being the better of the two. In the third stage, we consider two noisy multi-objective problems (CountingOnesCountingZeros and a multi-objective formulation of SetCover). We compare several adaptations of UMDA for multi-objective problems with the Simple Evolutionary Multi-objective Optimiser (SEMO) and NSGA-II. We conclude that UMDA, and its variants, can be highly effective on a variety of noisy combinatorial optimisation, outperforming many other evolutionary algorithms.
更多查看译文
关键词
algorithms,combinatorial,unconstrained,multi-objective
AI 理解论文
溯源树
样例
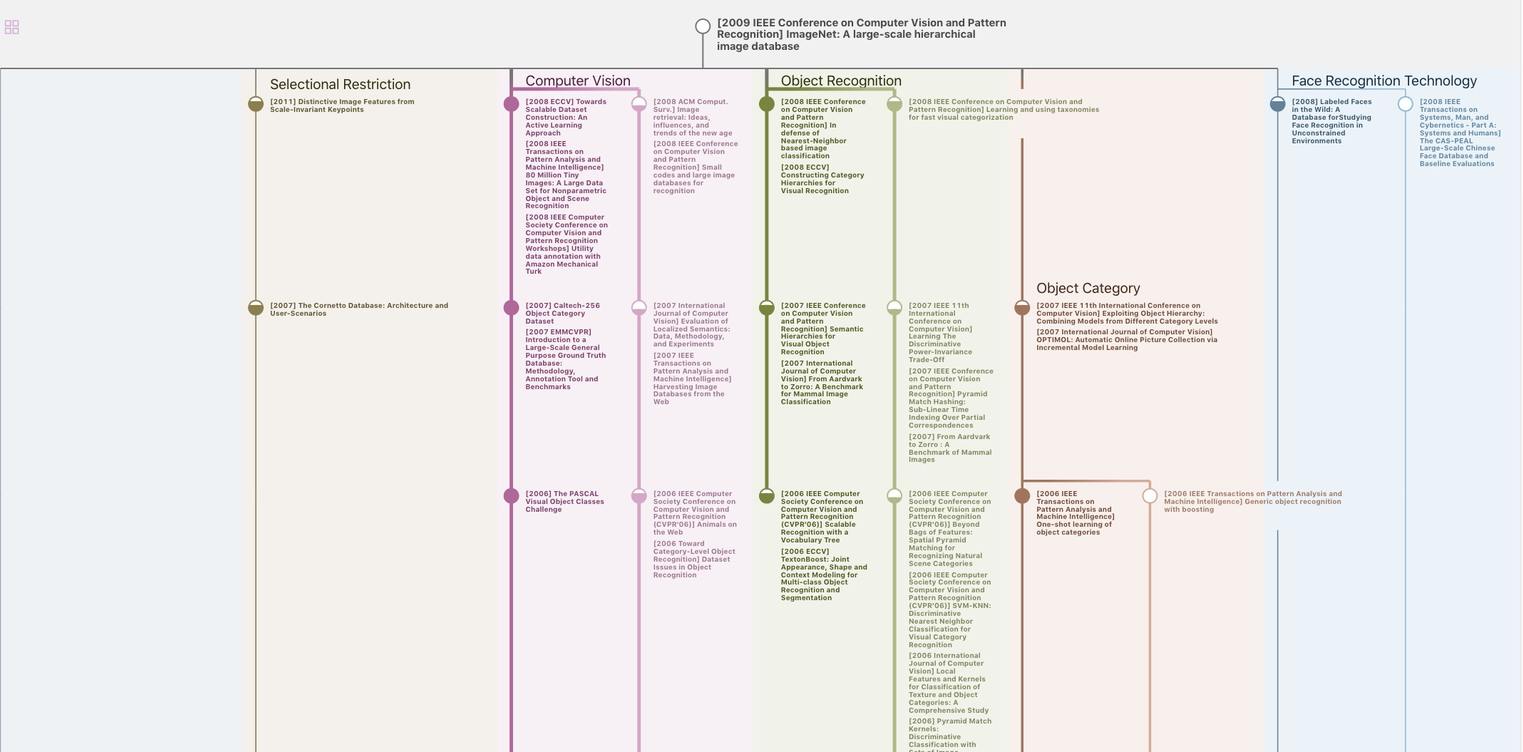
生成溯源树,研究论文发展脉络
Chat Paper
正在生成论文摘要