Inference and De-noising of Non-gaussian Particle Distribution Functions: A Generative Modeling Approach
MACHINE LEARNING, OPTIMIZATION, AND DATA SCIENCE (LOD 2021), PT I(2022)
摘要
The particle-in-cell numerical method of plasma physics balances a trade-off between computational cost and intrinsic noise. Inference on data produced by these simulations generally consists of binning the data to recover the particle distribution function, from which physical processes may be investigated. In addition to containing noise, the distribution function is temporally dynamic and can be non-gaussian and multi-modal, making the task of modeling it difficult. Here we demonstrate the use of normalizing flows to learn a smooth, tractable approximation to the noisy particle distribution function. We demonstrate that the resulting data driven likelihood conserves relevant physics and may be extended to encapsulate the temporal evolution of the distribution function.
更多查看译文
关键词
Normalizing flow, Plasma physics, Particle-in-cell, Core-edge coupling, Likelihood free inference
AI 理解论文
溯源树
样例
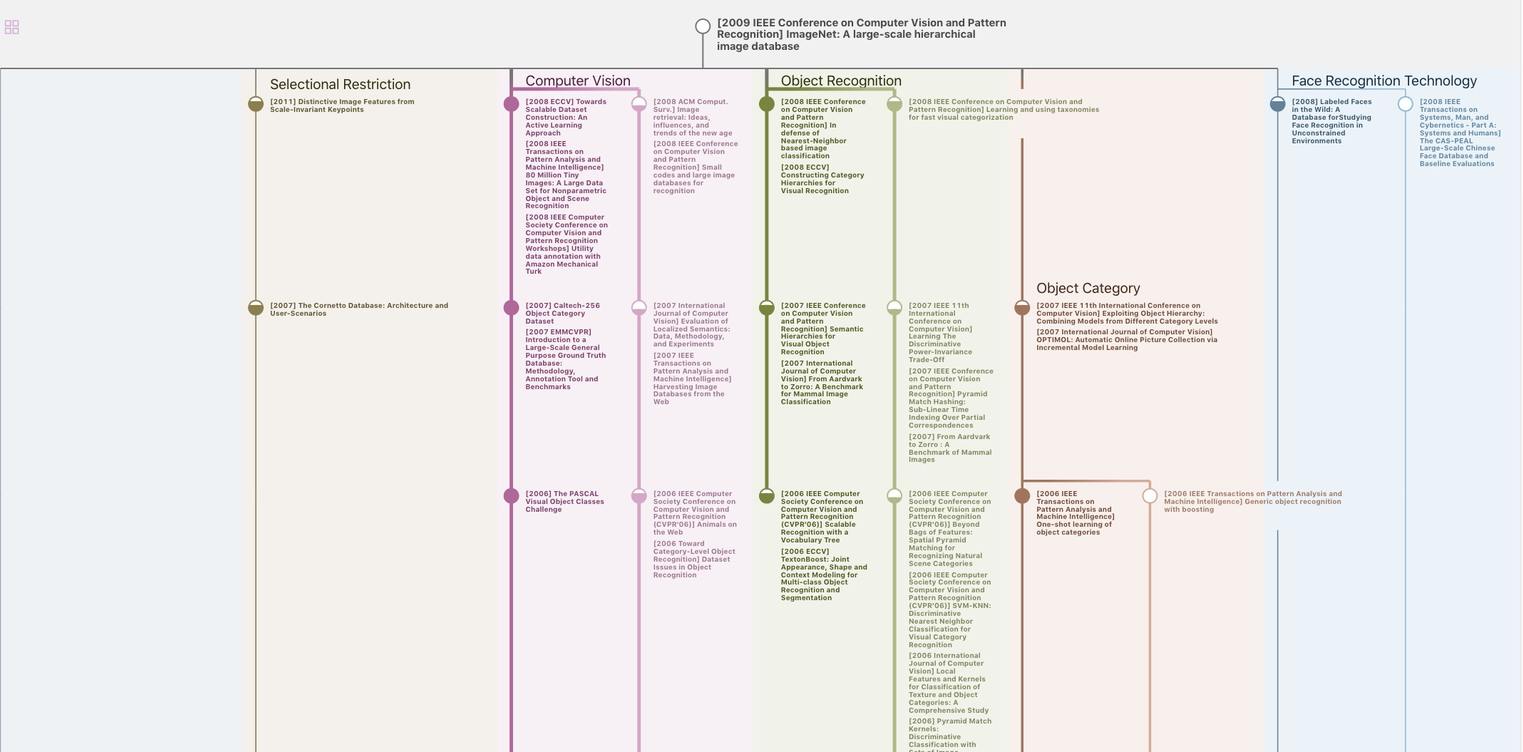
生成溯源树,研究论文发展脉络
Chat Paper
正在生成论文摘要