Optimization with constraint learning: A framework and survey
EUROPEAN JOURNAL OF OPERATIONAL RESEARCH(2024)
摘要
Many real-life optimization problems frequently contain one or more constraints or objectives for which there are no explicit formulae. If however data on feasible and/or infeasible states are available, these data can be used to learn the constraints. The benefits of this approach are clearly seen, however, there is a need for this process to be carried out in a structured manner. This paper, therefore, provides a framework for Optimization with Constraint Learning (OCL) which we believe will help to formalize and direct the process of learning constraints from data. This framework includes the following steps: (i) setup of the conceptual optimization model, (ii) data gathering and preprocessing, (iii) selection and training of predictive models, (iv) resolution of the optimization model, and (v) verification and improvement of the optimization model. We then review the recent OCL literature in light of this framework and highlight current trends, as well as areas for future research.(c) 2023 The Author(s). Published by Elsevier B.V. This is an open access article under the CC BY license ( http://creativecommons.org/licenses/by/4.0/ )
更多查看译文
关键词
Analytics,Optimization,Constraint learning,Machine learning
AI 理解论文
溯源树
样例
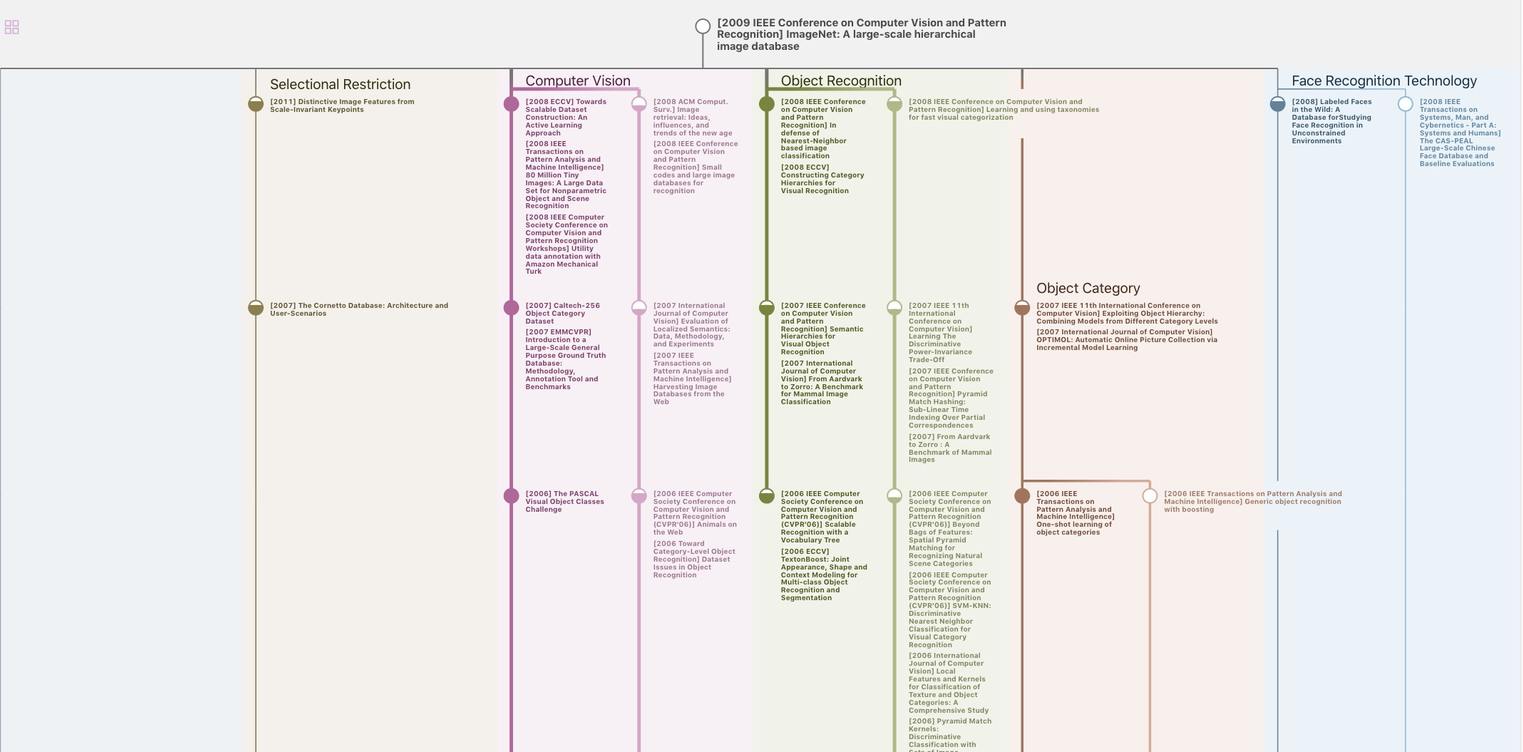
生成溯源树,研究论文发展脉络
Chat Paper
正在生成论文摘要