Gell-Mann-Low Criticality in Neural Networks
PHYSICAL REVIEW LETTERS(2022)
摘要
Criticality is deeply related to optimal computational capacity. The lack of a renormalized theory of critical brain dynamics, however, so far limits insights into this form of biological information processing to mean-field results. These methods neglect a key feature of critical systems: the interaction between degrees of freedom across all length scales, required for complex nonlinear computation. We present a renormalized theory of a prototypical neural field theory, the stochastic Wilson-Cowan equation. We compute the flow of couplings, which parametrize interactions on increasing length scales. Despite similarities with the KardarParisi-Zhang model, the theory is of a Gell-Mann???Low type, the archetypal form of a renormalizable quantum field theory. Here, nonlinear couplings vanish, flowing towards the Gaussian fixed point, but logarithmically slowly, thus remaining effective on most scales. We show this critical structure of interactions to implement a desirable trade-off between linearity, optimal for information storage, and nonlinearity, required for computation.
更多查看译文
关键词
gell-mann–low criticality,neural networks
AI 理解论文
溯源树
样例
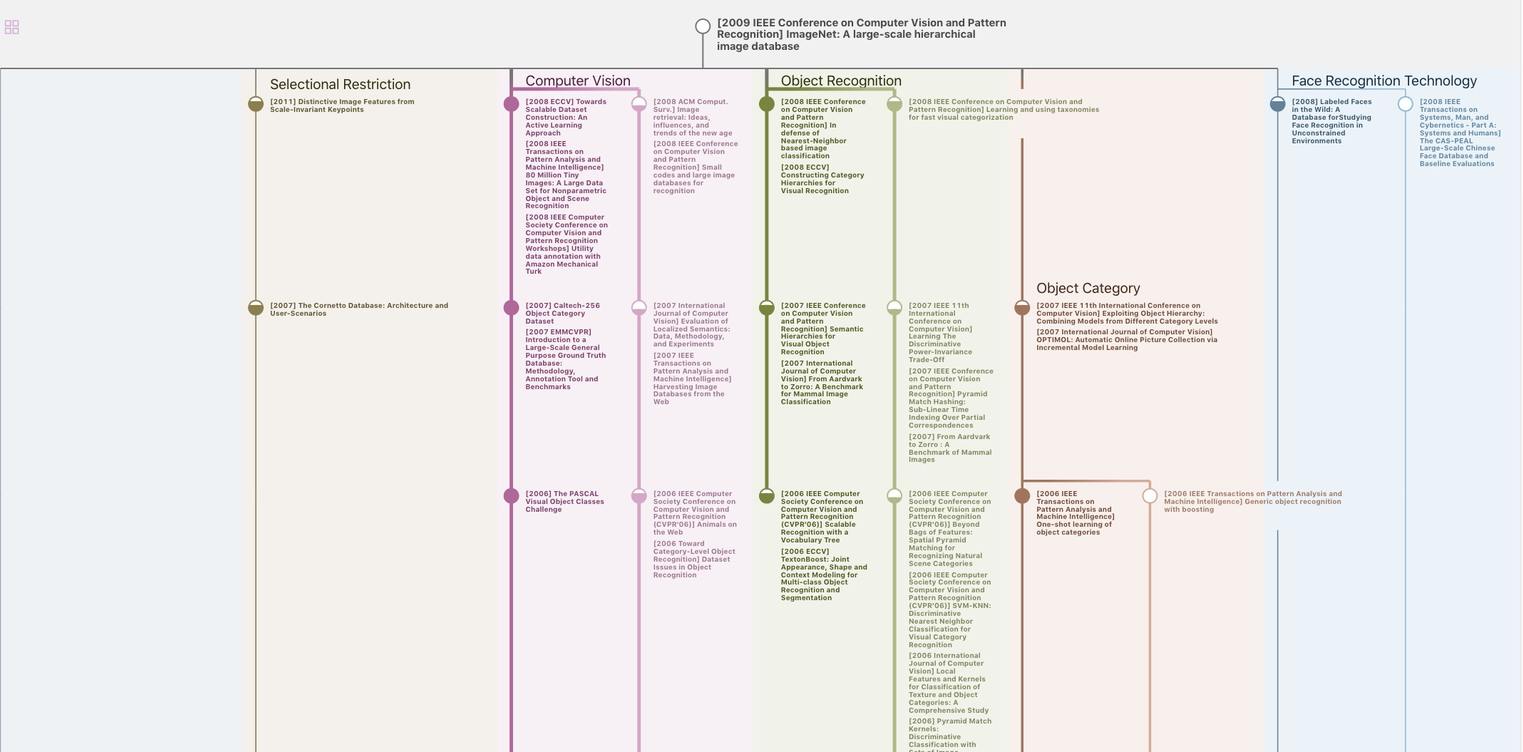
生成溯源树,研究论文发展脉络
Chat Paper
正在生成论文摘要