Kalman Bayesian Neural Networks for Closed-form Online Learning
arxiv(2022)
摘要
Compared to point estimates calculated by standard neural networks, Bayesian neural networks (BNN) provide probability distributions over the output predictions and model parameters, i.e., the weights. Training the weight distribution of a BNN, however, is more involved due to the intractability of the underlying Bayesian inference problem and thus, requires efficient approximations. In this paper, we propose a novel approach for BNN learning via closed-form Bayesian inference. For this purpose, the calculation of the predictive distribution of the output and the update of the weight distribution are treated as Bayesian filtering and smoothing problems, where the weights are modeled as Gaussian random variables. This allows closed-form expressions for training the network's parameters in a sequential/online fashion without gradient descent. We demonstrate our method on several UCI datasets and compare it to the state of the art.
更多查看译文
关键词
online learning,neural networks,closed-form
AI 理解论文
溯源树
样例
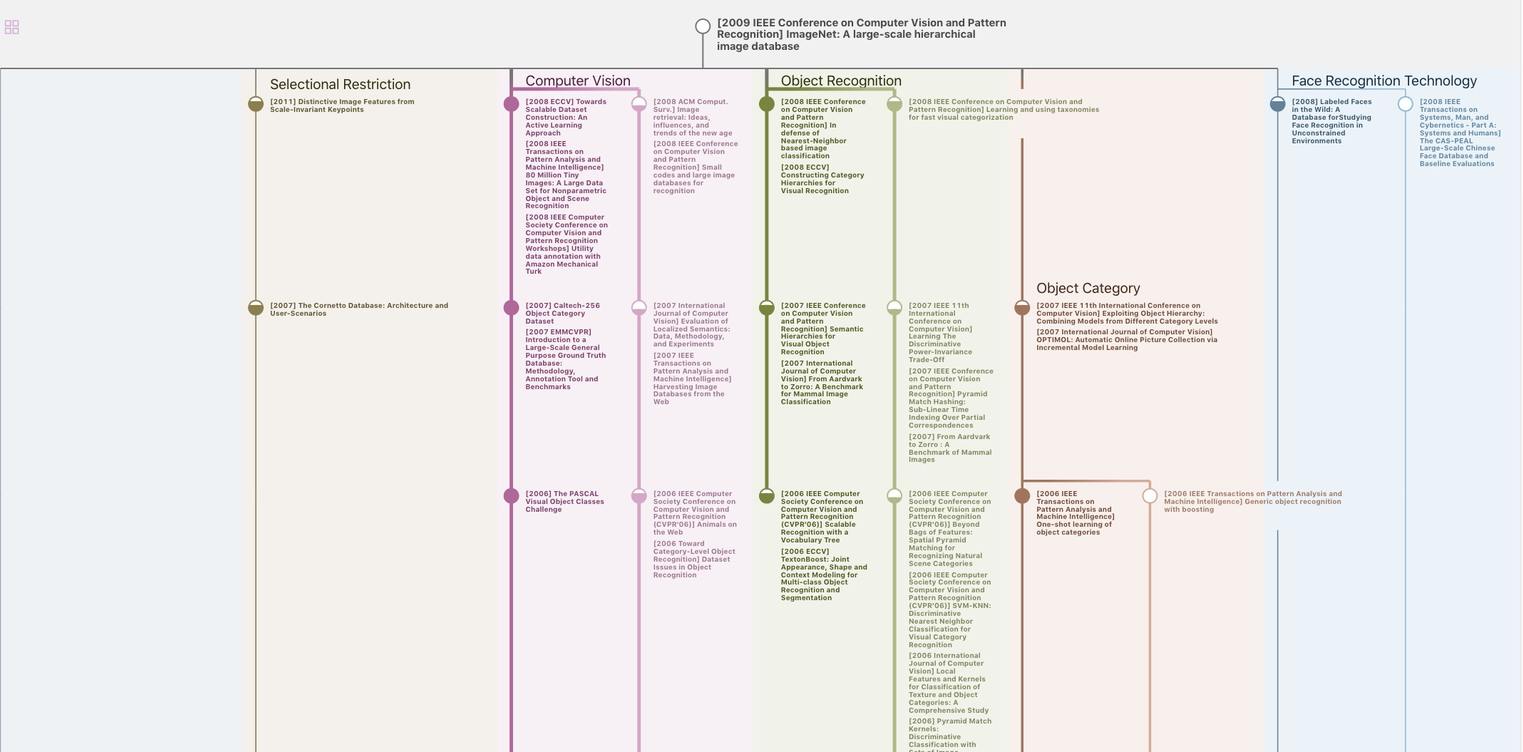
生成溯源树,研究论文发展脉络
Chat Paper
正在生成论文摘要